AI in Balancing Electric Supply and Demand
AI in Balancing Electric Supply and Demand
This presentation explores a case study on using Artificial Intelligence to balance electricity supply and demand. It highlights the technology's impact on load forecasting, energy management, and integration of renewable energy sources. The insights include practical applications from organizations like Hydro-Québec, addressing how AI is revolutionizing the reliability and efficiency of the power grid amidst evolving energy challenges.
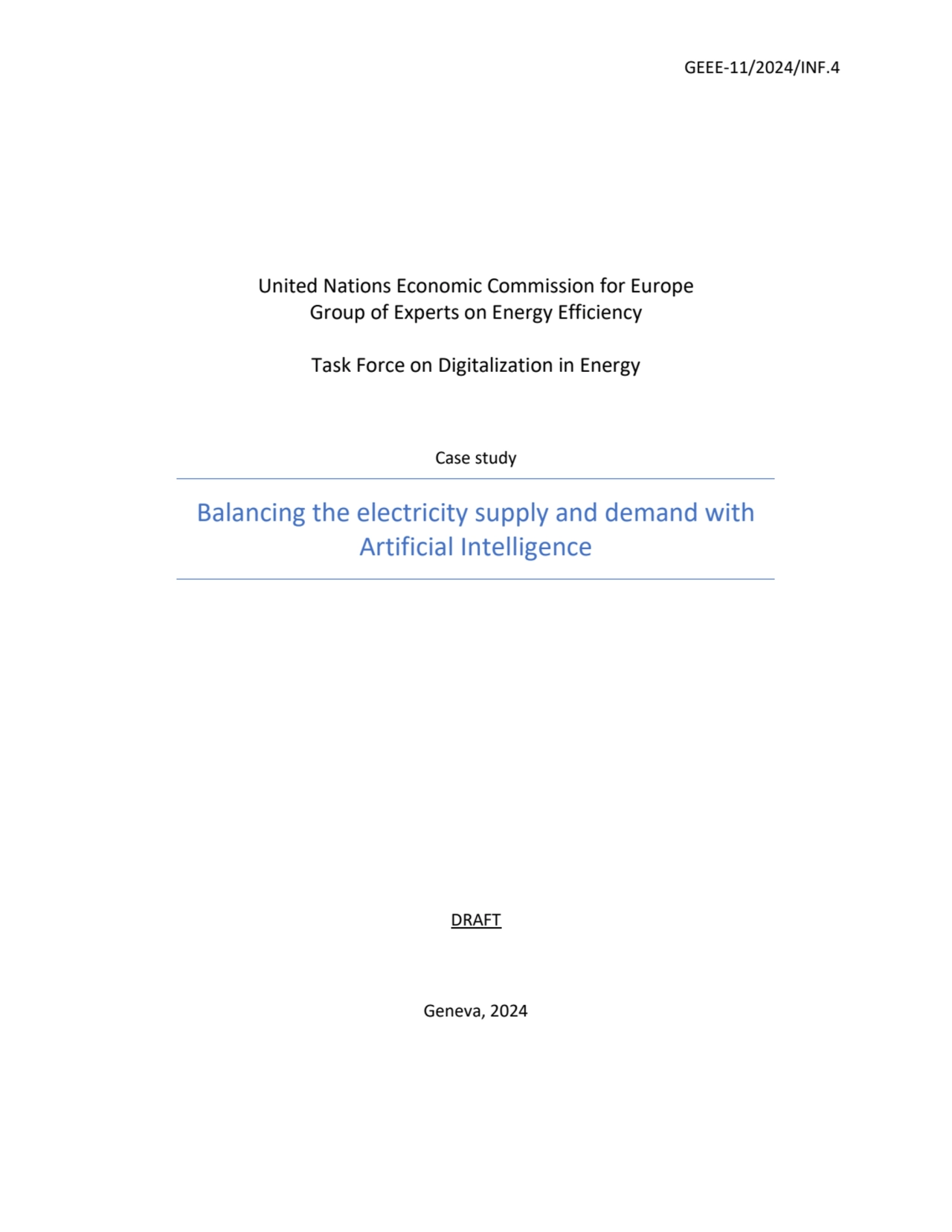
1/17
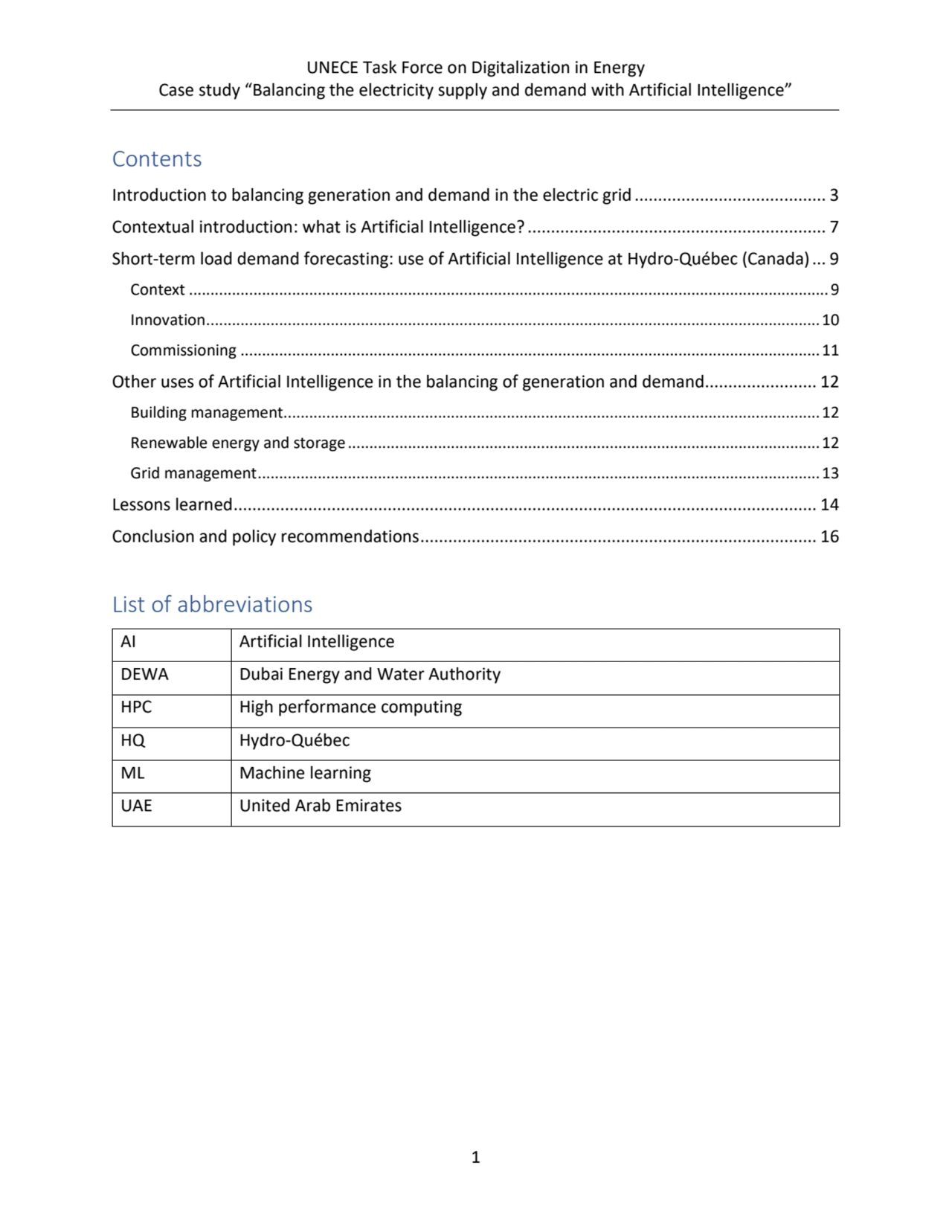
2/17
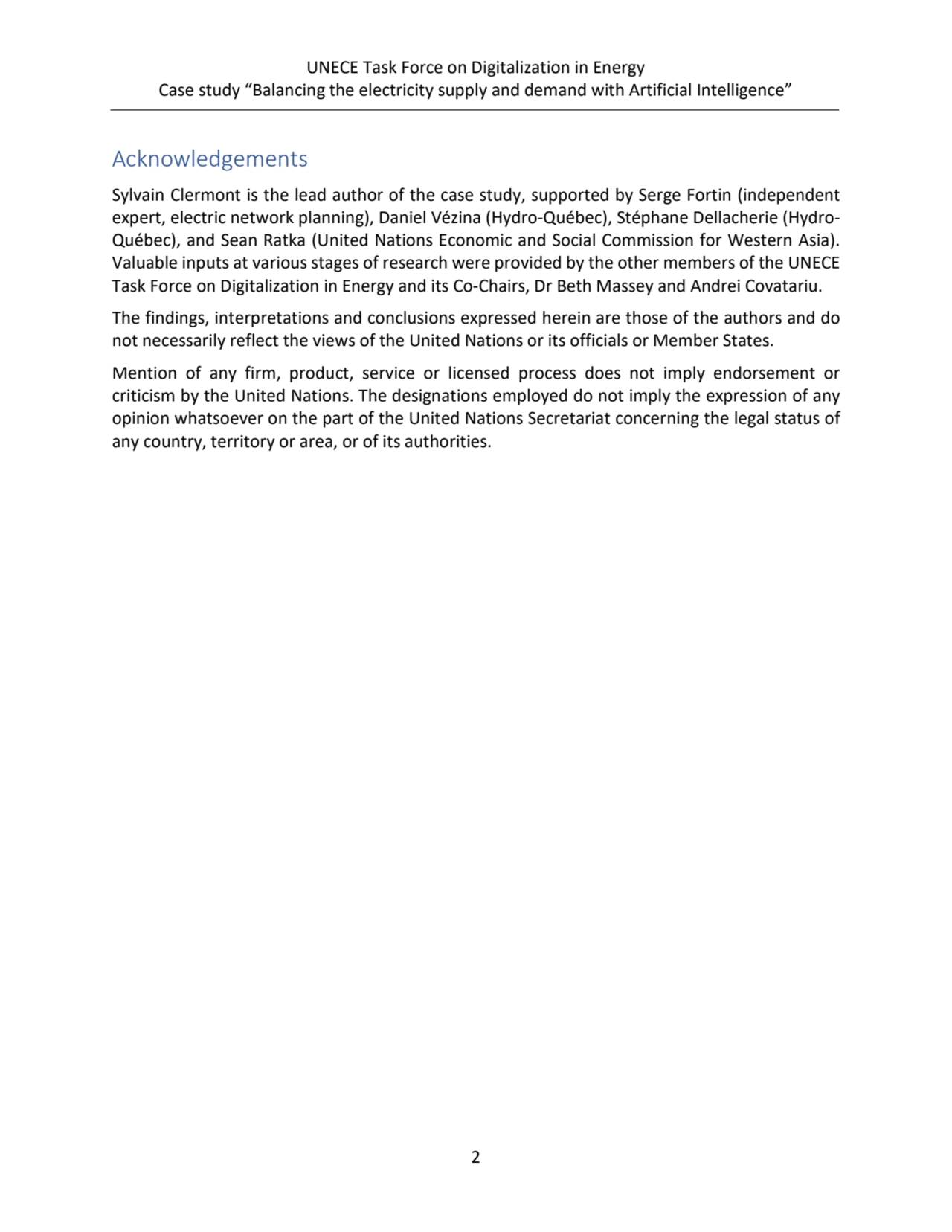
3/17
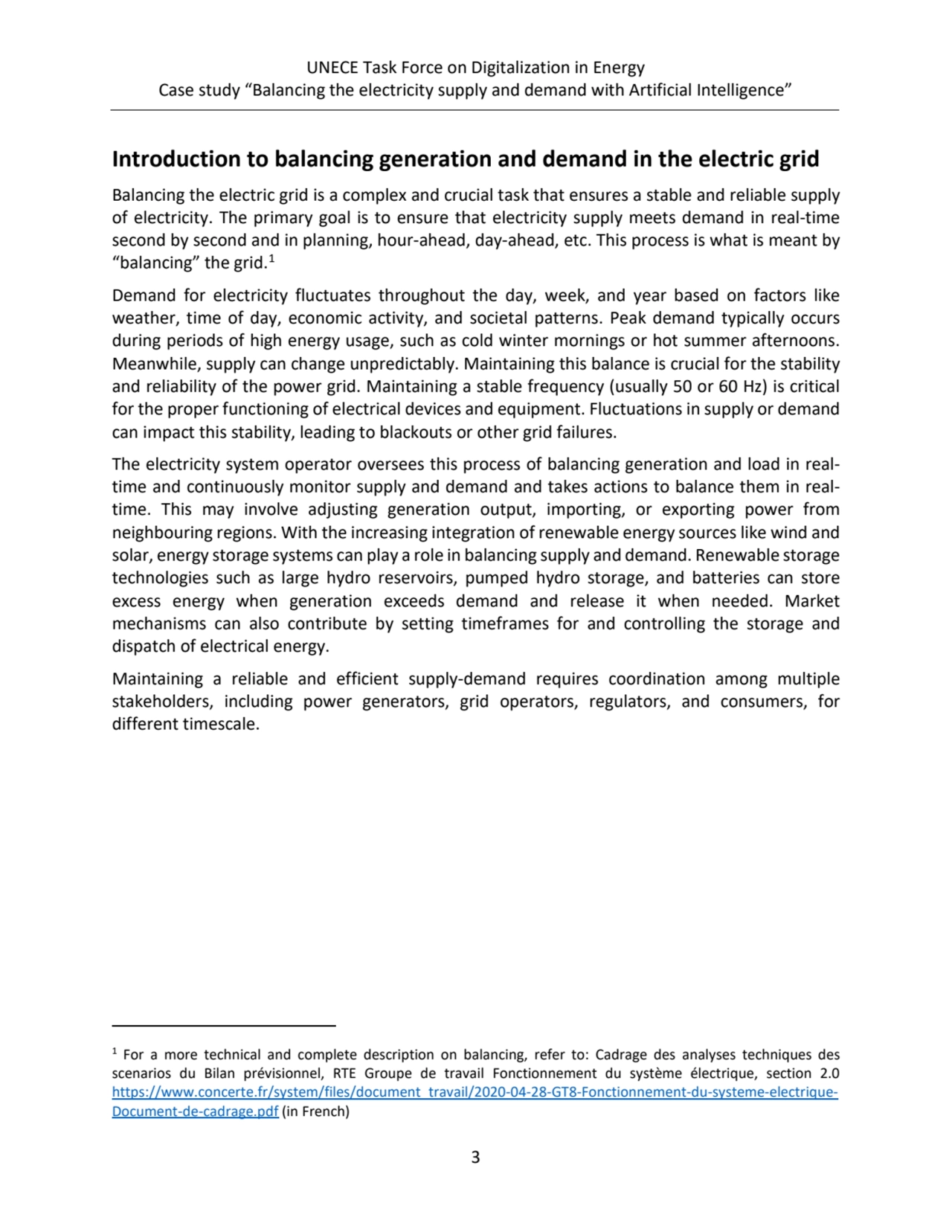
4/17
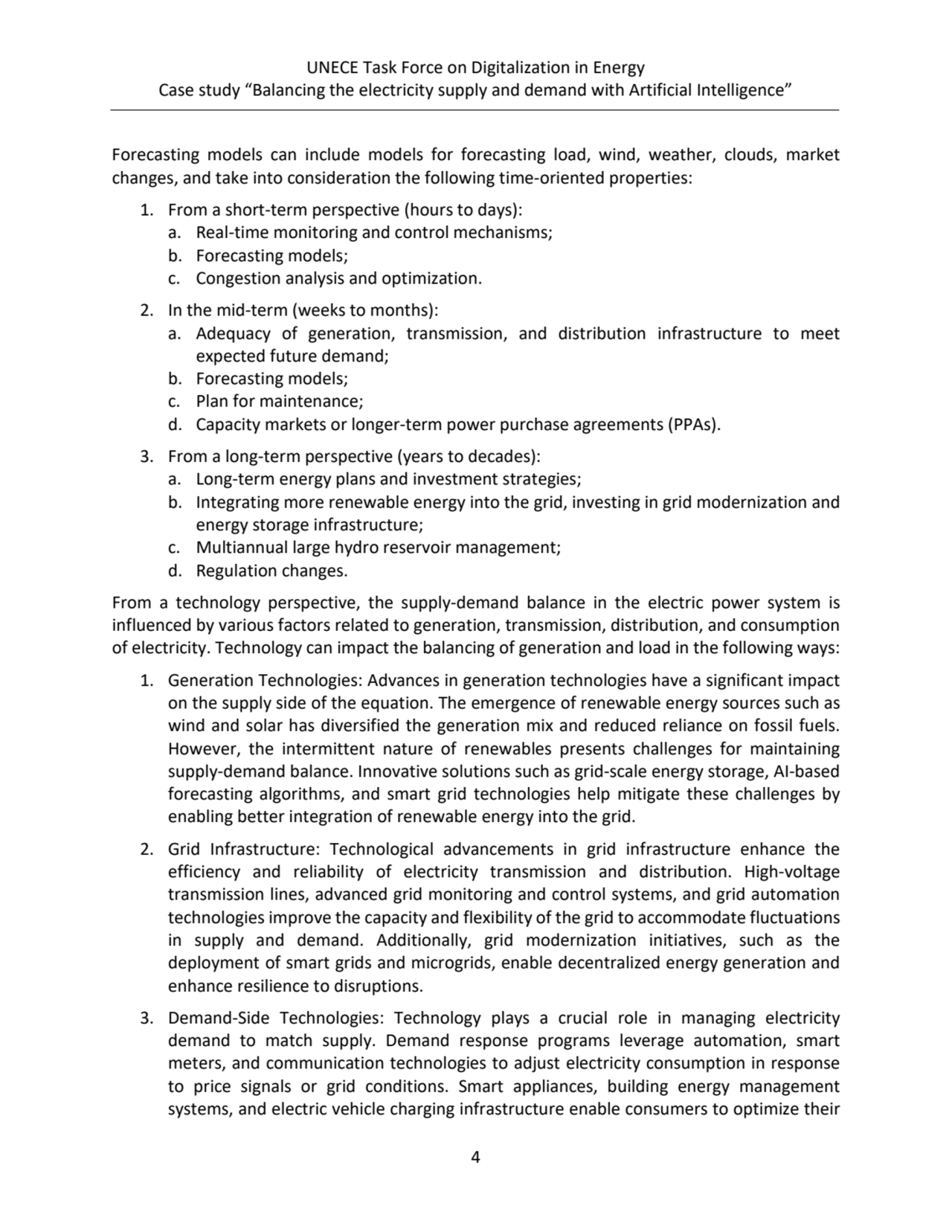
5/17
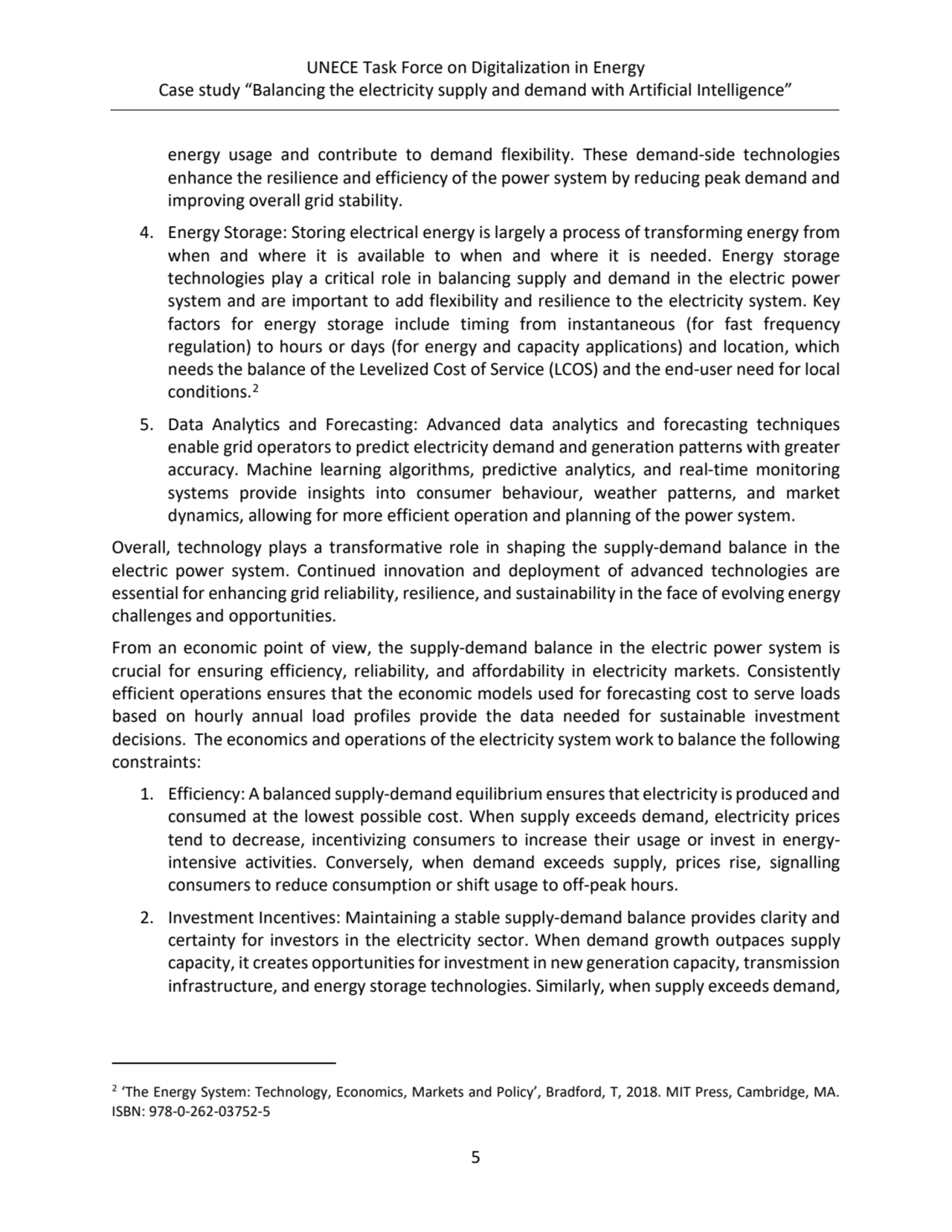
6/17
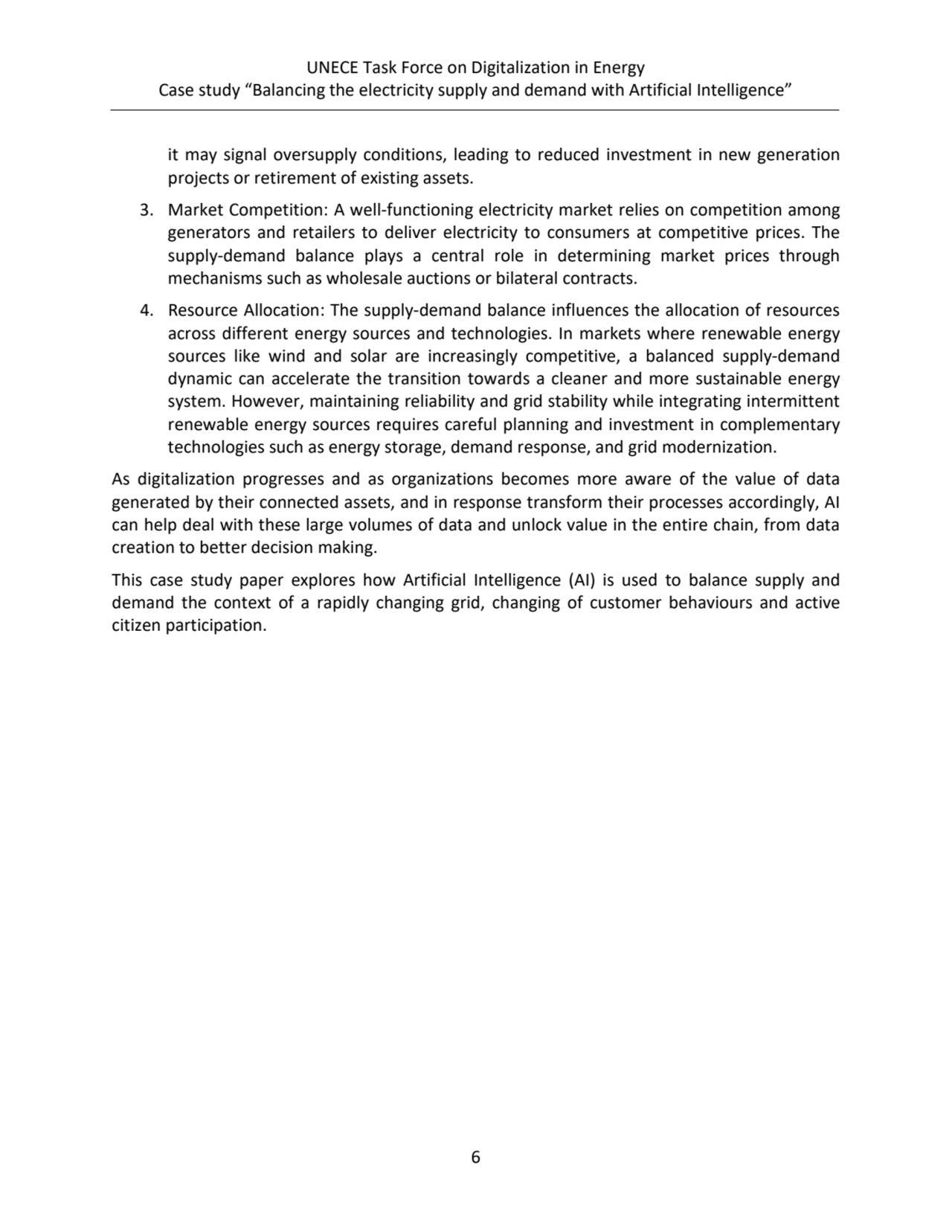
7/17
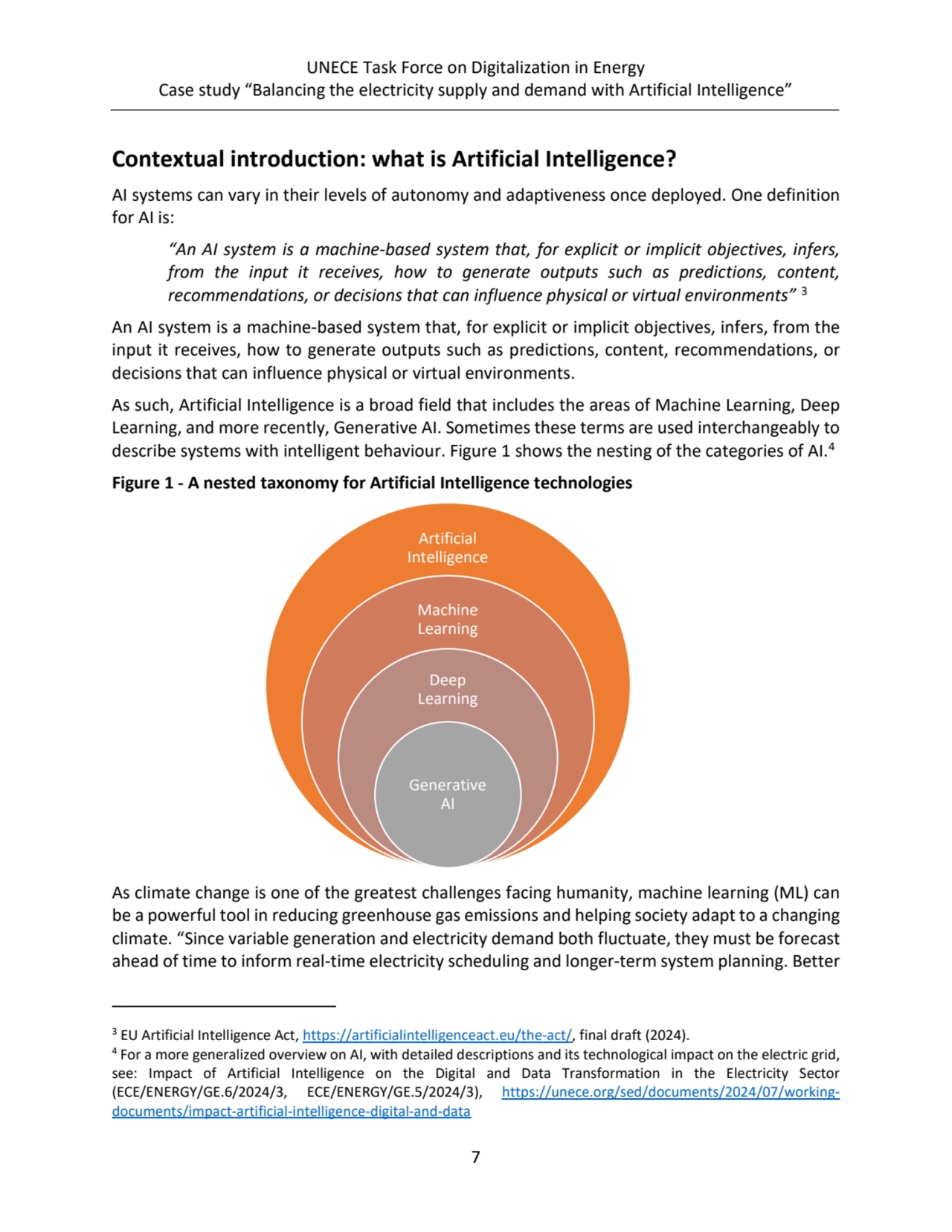
8/17
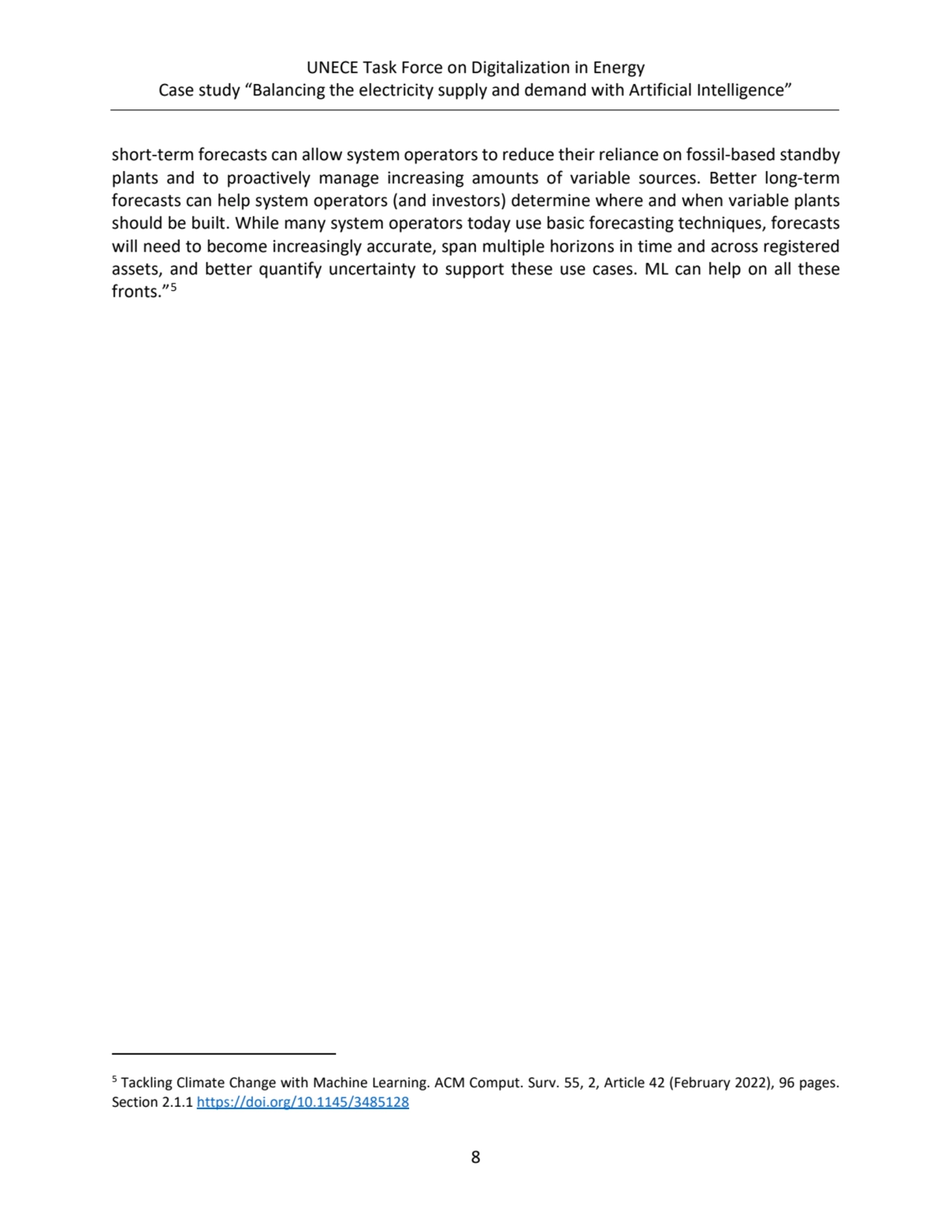
9/17
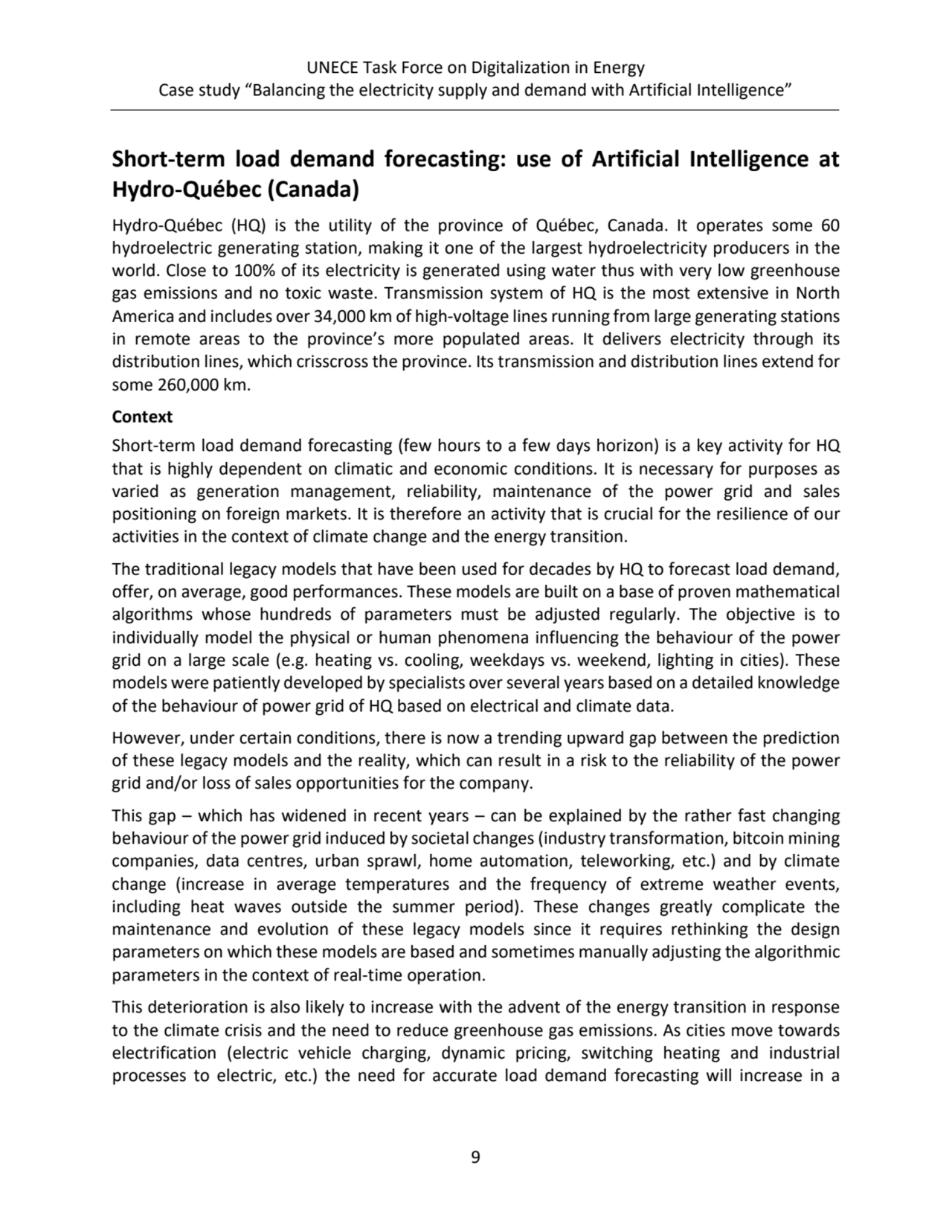
10/17
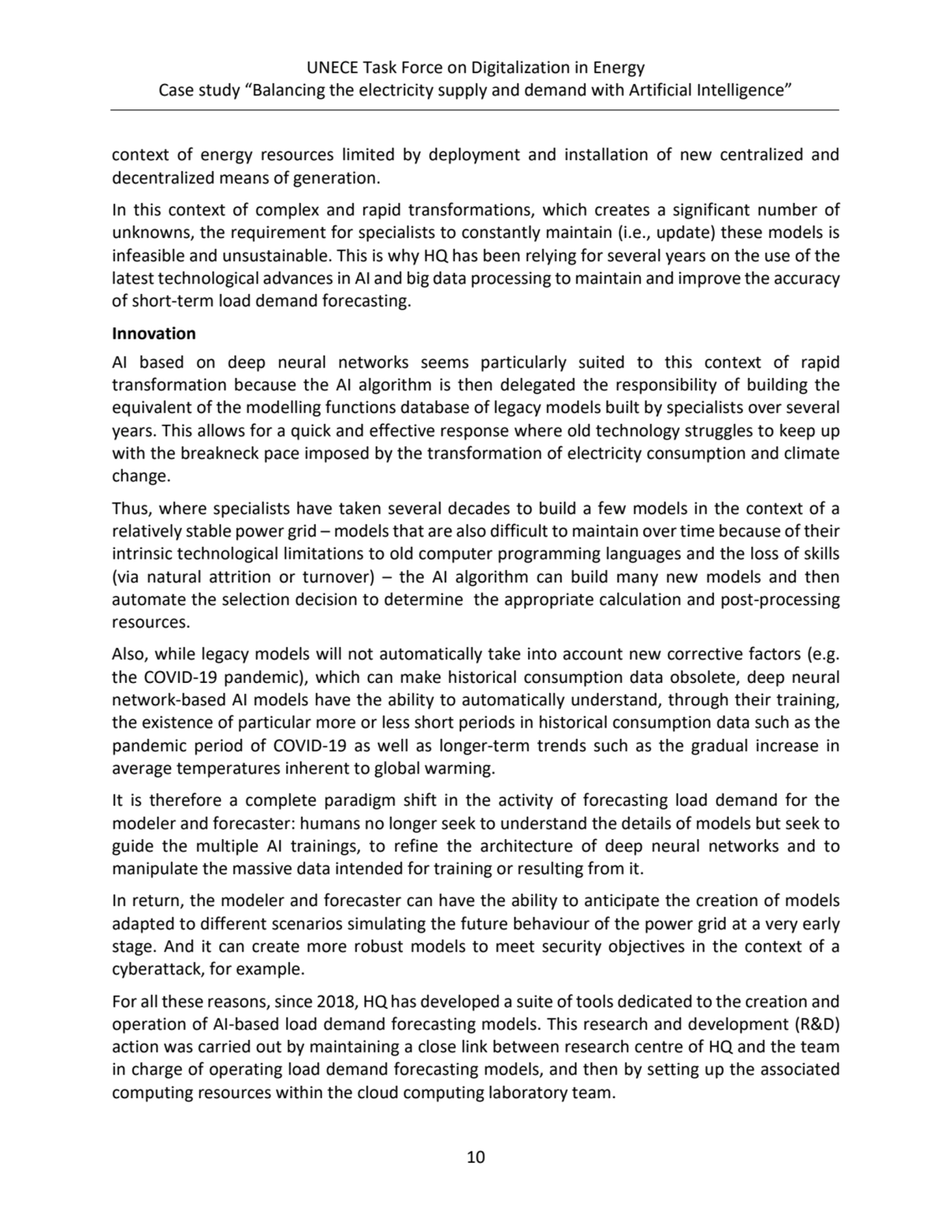
11/17
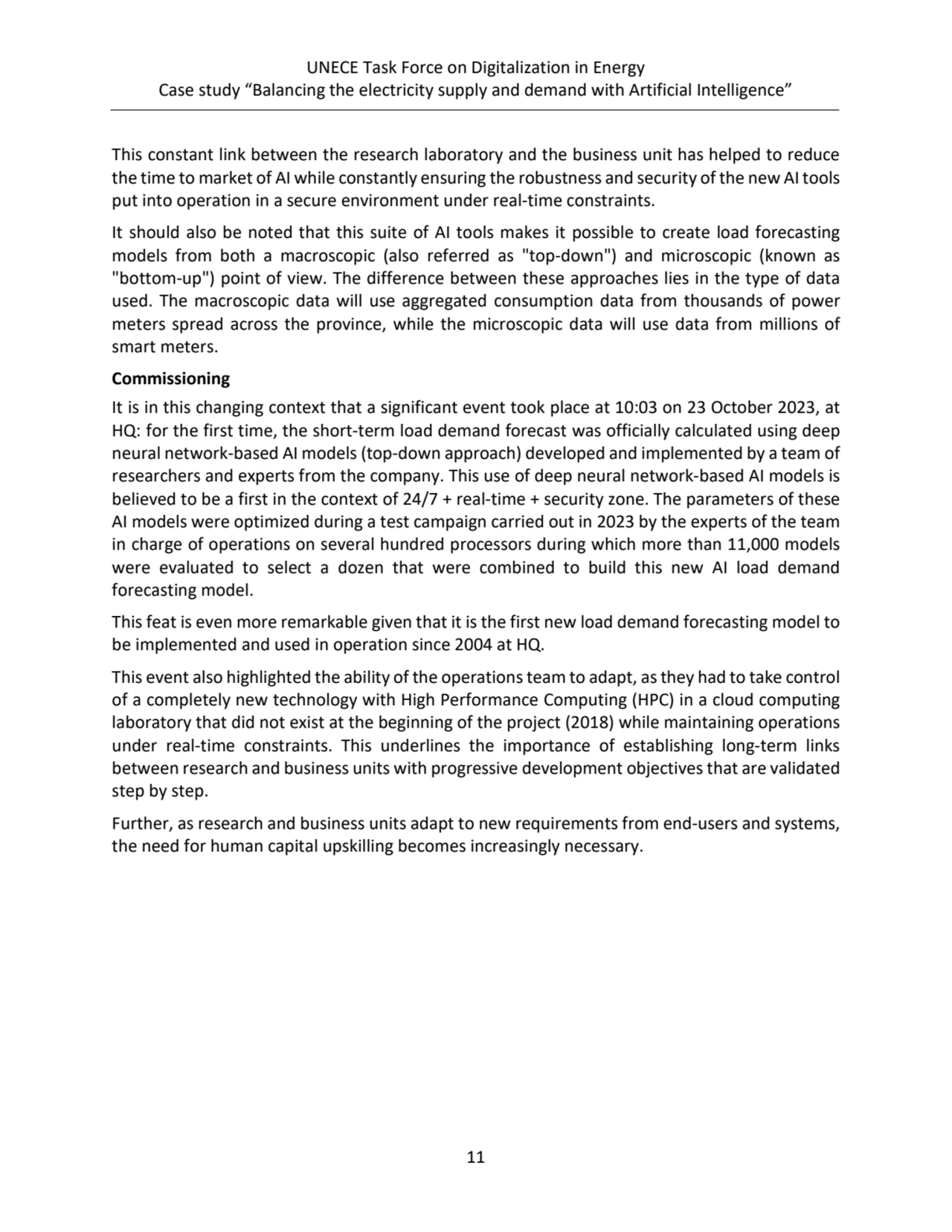
12/17
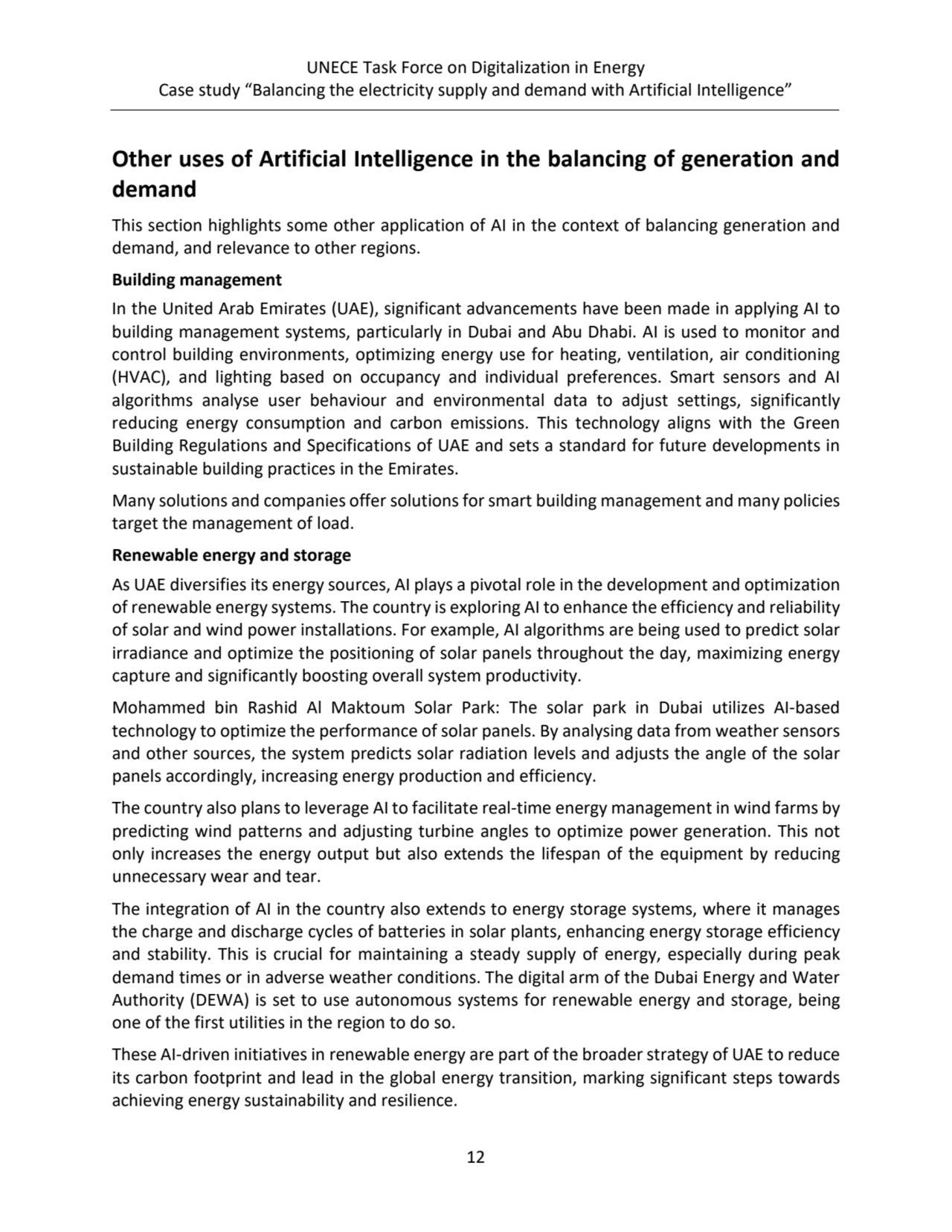
13/17
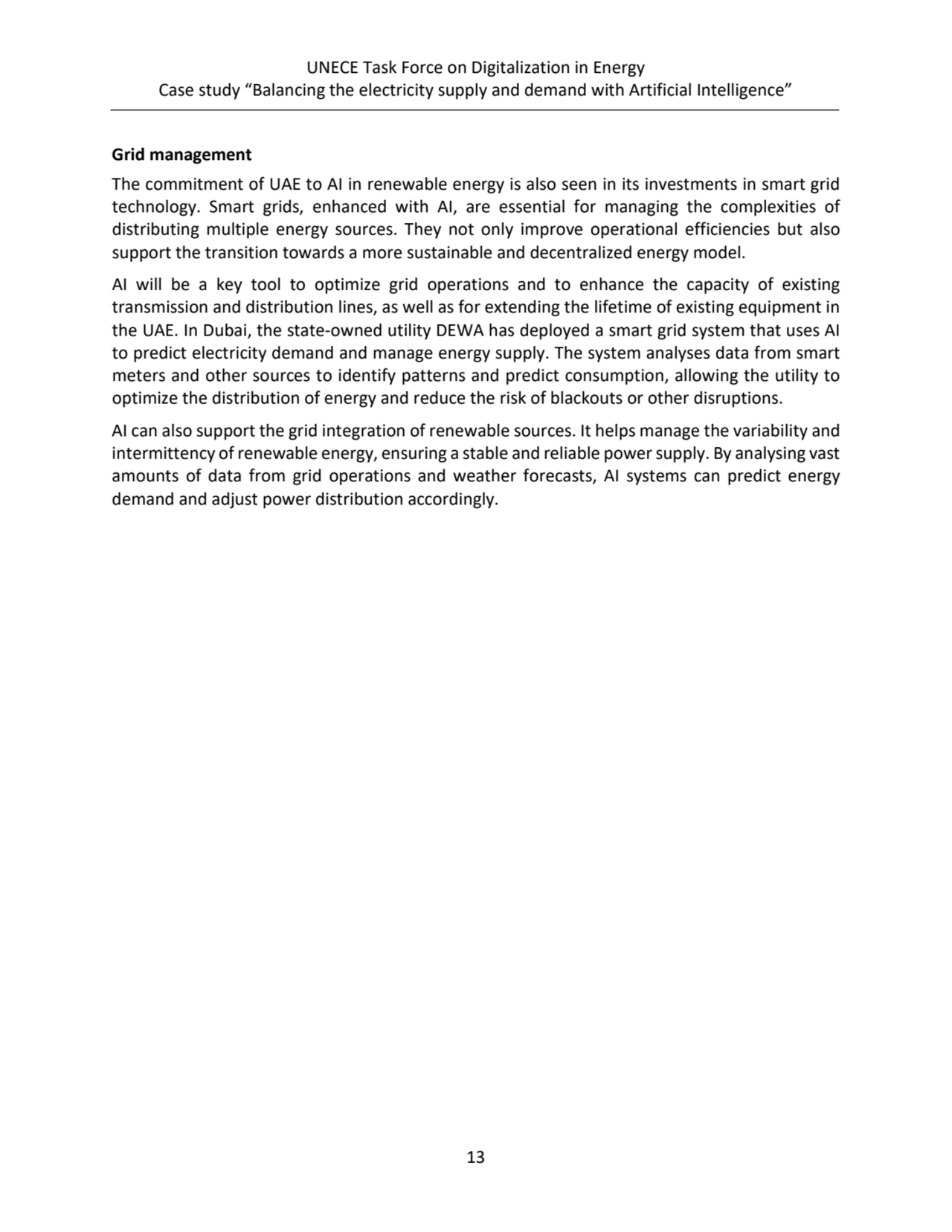
14/17
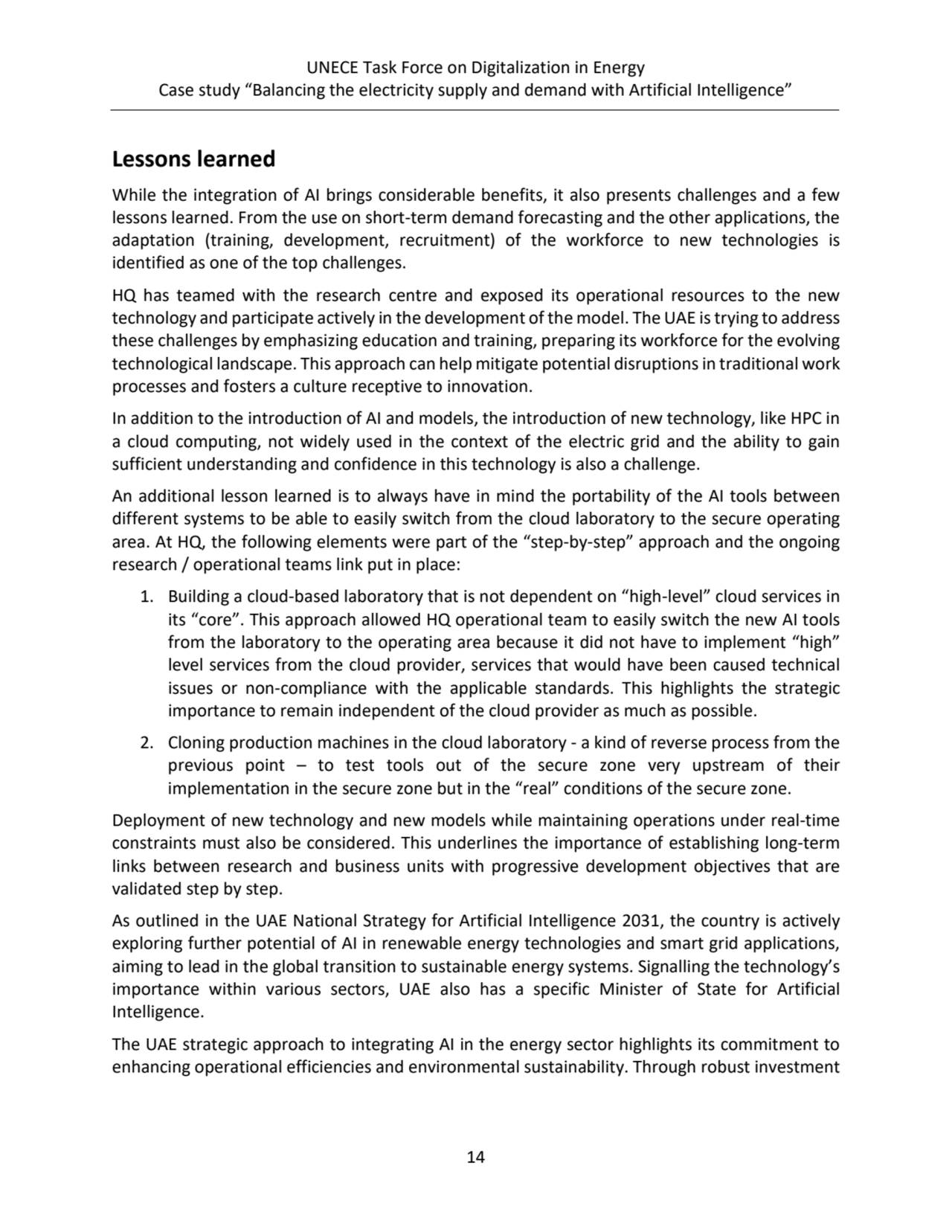
15/17
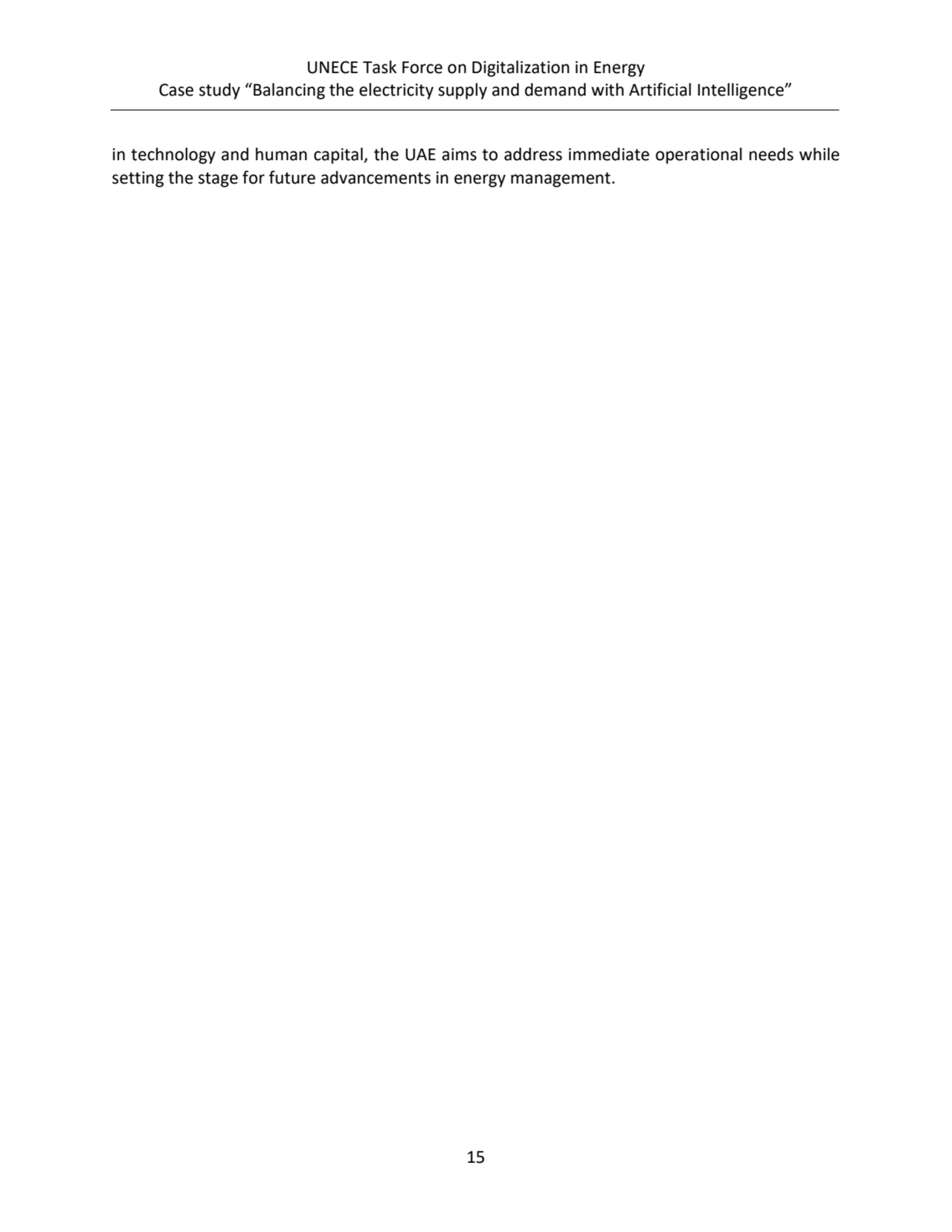
16/17
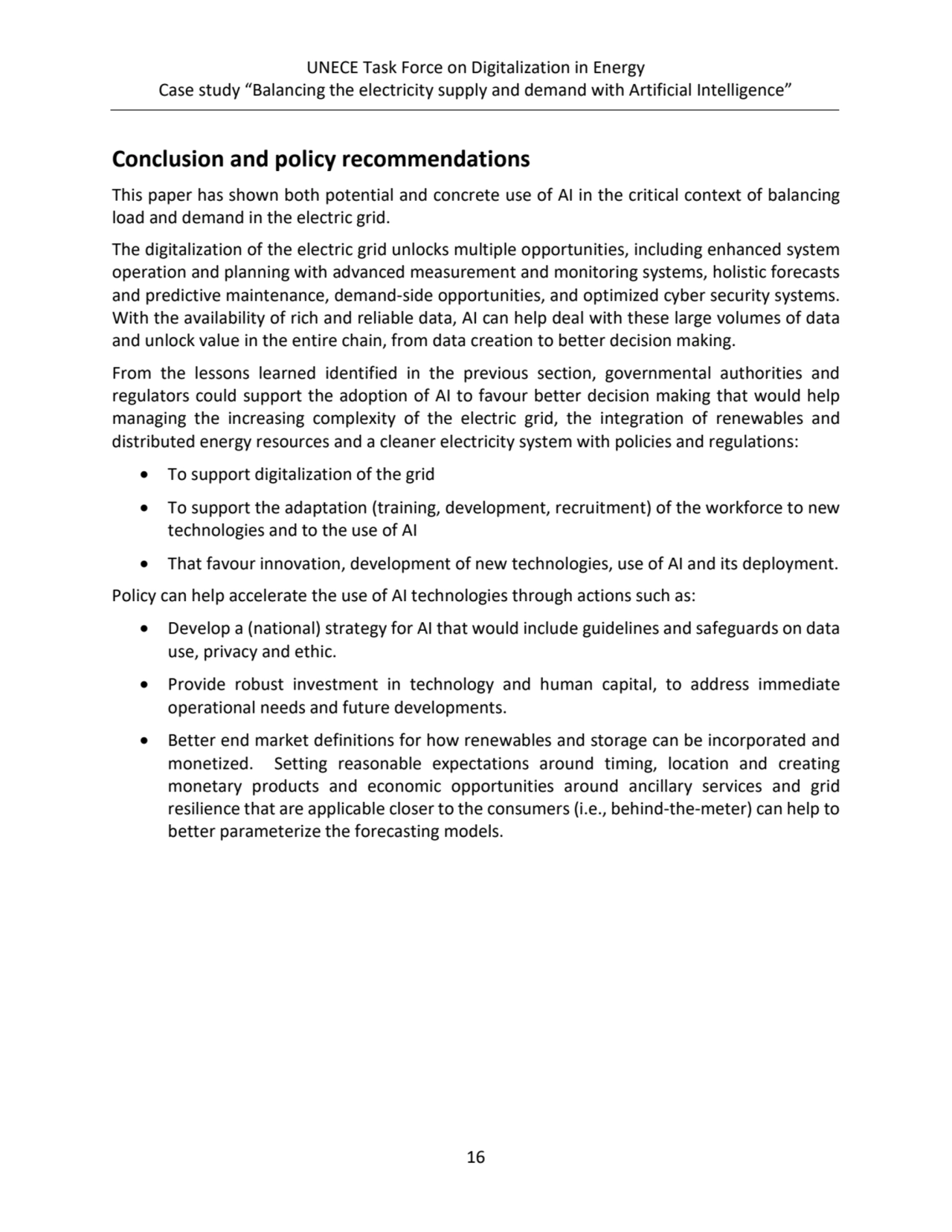
17/17
AI in Balancing Electric Supply and Demand
- 1. GEEE-11/2024/INF.4 United Nations Economic Commission for Europe Group of Experts on Energy Efficiency Task Force on Digitalization in Energy Case study Balancing the electricity supply and demand with Artificial Intelligence DRAFT Geneva, 2024
- 2. UNECE Task Force on Digitalization in Energy Case study “Balancing the electricity supply and demand with Artificial Intelligence” 1 Contents Introduction to balancing generation and demand in the electric grid ......................................... 3 Contextual introduction: what is Artificial Intelligence? ................................................................ 7 Short-term load demand forecasting: use of Artificial Intelligence at Hydro-Québec (Canada)... 9 Context .....................................................................................................................................................9 Innovation...............................................................................................................................................10 Commissioning .......................................................................................................................................11 Other uses of Artificial Intelligence in the balancing of generation and demand........................ 12 Building management.............................................................................................................................12 Renewable energy and storage..............................................................................................................12 Grid management...................................................................................................................................13 Lessons learned............................................................................................................................. 14 Conclusion and policy recommendations..................................................................................... 16 List of abbreviations AI Artificial Intelligence DEWA Dubai Energy and Water Authority HPC High performance computing HQ Hydro-Québec ML Machine learning UAE United Arab Emirates
- 3. UNECE Task Force on Digitalization in Energy Case study “Balancing the electricity supply and demand with Artificial Intelligence” 2 Acknowledgements Sylvain Clermont is the lead author of the case study, supported by Serge Fortin (independent expert, electric network planning), Daniel Vézina (Hydro-Québec), Stéphane Dellacherie (HydroQuébec), and Sean Ratka (United Nations Economic and Social Commission for Western Asia). Valuable inputs at various stages of research were provided by the other members of the UNECE Task Force on Digitalization in Energy and its Co-Chairs, Dr Beth Massey and Andrei Covatariu. The findings, interpretations and conclusions expressed herein are those of the authors and do not necessarily reflect the views of the United Nations or its officials or Member States. Mention of any firm, product, service or licensed process does not imply endorsement or criticism by the United Nations. The designations employed do not imply the expression of any opinion whatsoever on the part of the United Nations Secretariat concerning the legal status of any country, territory or area, or of its authorities.
- 4. UNECE Task Force on Digitalization in Energy Case study “Balancing the electricity supply and demand with Artificial Intelligence” 3 Introduction to balancing generation and demand in the electric grid Balancing the electric grid is a complex and crucial task that ensures a stable and reliable supply of electricity. The primary goal is to ensure that electricity supply meets demand in real-time second by second and in planning, hour-ahead, day-ahead, etc. This process is what is meant by “balancing” the grid.1 Demand for electricity fluctuates throughout the day, week, and year based on factors like weather, time of day, economic activity, and societal patterns. Peak demand typically occurs during periods of high energy usage, such as cold winter mornings or hot summer afternoons. Meanwhile, supply can change unpredictably. Maintaining this balance is crucial for the stability and reliability of the power grid. Maintaining a stable frequency (usually 50 or 60 Hz) is critical for the proper functioning of electrical devices and equipment. Fluctuations in supply or demand can impact this stability, leading to blackouts or other grid failures. The electricity system operator oversees this process of balancing generation and load in realtime and continuously monitor supply and demand and takes actions to balance them in realtime. This may involve adjusting generation output, importing, or exporting power from neighbouring regions. With the increasing integration of renewable energy sources like wind and solar, energy storage systems can play a role in balancing supply and demand. Renewable storage technologies such as large hydro reservoirs, pumped hydro storage, and batteries can store excess energy when generation exceeds demand and release it when needed. Market mechanisms can also contribute by setting timeframes for and controlling the storage and dispatch of electrical energy. Maintaining a reliable and efficient supply-demand requires coordination among multiple stakeholders, including power generators, grid operators, regulators, and consumers, for different timescale. 1 For a more technical and complete description on balancing, refer to: Cadrage des analyses techniques des scenarios du Bilan prévisionnel, RTE Groupe de travail Fonctionnement du système électrique, section 2.0 https://www.concerte.fr/system/files/document_travail/2020-04-28-GT8-Fonctionnement-du-systeme-electriqueDocument-de-cadrage.pdf (in French)
- 5. UNECE Task Force on Digitalization in Energy Case study “Balancing the electricity supply and demand with Artificial Intelligence” 4 Forecasting models can include models for forecasting load, wind, weather, clouds, market changes, and take into consideration the following time-oriented properties: 1. From a short-term perspective (hours to days): a. Real-time monitoring and control mechanisms; b. Forecasting models; c. Congestion analysis and optimization. 2. In the mid-term (weeks to months): a. Adequacy of generation, transmission, and distribution infrastructure to meet expected future demand; b. Forecasting models; c. Plan for maintenance; d. Capacity markets or longer-term power purchase agreements (PPAs). 3. From a long-term perspective (years to decades): a. Long-term energy plans and investment strategies; b. Integrating more renewable energy into the grid, investing in grid modernization and energy storage infrastructure; c. Multiannual large hydro reservoir management; d. Regulation changes. From a technology perspective, the supply-demand balance in the electric power system is influenced by various factors related to generation, transmission, distribution, and consumption of electricity. Technology can impact the balancing of generation and load in the following ways: 1. Generation Technologies: Advances in generation technologies have a significant impact on the supply side of the equation. The emergence of renewable energy sources such as wind and solar has diversified the generation mix and reduced reliance on fossil fuels. However, the intermittent nature of renewables presents challenges for maintaining supply-demand balance. Innovative solutions such as grid-scale energy storage, AI-based forecasting algorithms, and smart grid technologies help mitigate these challenges by enabling better integration of renewable energy into the grid. 2. Grid Infrastructure: Technological advancements in grid infrastructure enhance the efficiency and reliability of electricity transmission and distribution. High-voltage transmission lines, advanced grid monitoring and control systems, and grid automation technologies improve the capacity and flexibility of the grid to accommodate fluctuations in supply and demand. Additionally, grid modernization initiatives, such as the deployment of smart grids and microgrids, enable decentralized energy generation and enhance resilience to disruptions. 3. Demand-Side Technologies: Technology plays a crucial role in managing electricity demand to match supply. Demand response programs leverage automation, smart meters, and communication technologies to adjust electricity consumption in response to price signals or grid conditions. Smart appliances, building energy management systems, and electric vehicle charging infrastructure enable consumers to optimize their
- 6. UNECE Task Force on Digitalization in Energy Case study “Balancing the electricity supply and demand with Artificial Intelligence” 5 energy usage and contribute to demand flexibility. These demand-side technologies enhance the resilience and efficiency of the power system by reducing peak demand and improving overall grid stability. 4. Energy Storage: Storing electrical energy is largely a process of transforming energy from when and where it is available to when and where it is needed. Energy storage technologies play a critical role in balancing supply and demand in the electric power system and are important to add flexibility and resilience to the electricity system. Key factors for energy storage include timing from instantaneous (for fast frequency regulation) to hours or days (for energy and capacity applications) and location, which needs the balance of the Levelized Cost of Service (LCOS) and the end-user need for local conditions. 2 5. Data Analytics and Forecasting: Advanced data analytics and forecasting techniques enable grid operators to predict electricity demand and generation patterns with greater accuracy. Machine learning algorithms, predictive analytics, and real-time monitoring systems provide insights into consumer behaviour, weather patterns, and market dynamics, allowing for more efficient operation and planning of the power system. Overall, technology plays a transformative role in shaping the supply-demand balance in the electric power system. Continued innovation and deployment of advanced technologies are essential for enhancing grid reliability, resilience, and sustainability in the face of evolving energy challenges and opportunities. From an economic point of view, the supply-demand balance in the electric power system is crucial for ensuring efficiency, reliability, and affordability in electricity markets. Consistently efficient operations ensures that the economic models used for forecasting cost to serve loads based on hourly annual load profiles provide the data needed for sustainable investment decisions. The economics and operations of the electricity system work to balance the following constraints: 1. Efficiency: A balanced supply-demand equilibrium ensures that electricity is produced and consumed at the lowest possible cost. When supply exceeds demand, electricity prices tend to decrease, incentivizing consumers to increase their usage or invest in energyintensive activities. Conversely, when demand exceeds supply, prices rise, signalling consumers to reduce consumption or shift usage to off-peak hours. 2. Investment Incentives: Maintaining a stable supply-demand balance provides clarity and certainty for investors in the electricity sector. When demand growth outpaces supply capacity, it creates opportunities for investment in new generation capacity, transmission infrastructure, and energy storage technologies. Similarly, when supply exceeds demand, 2 ‘The Energy System: Technology, Economics, Markets and Policy’, Bradford, T, 2018. MIT Press, Cambridge, MA. ISBN: 978-0-262-03752-5
- 7. UNECE Task Force on Digitalization in Energy Case study “Balancing the electricity supply and demand with Artificial Intelligence” 6 it may signal oversupply conditions, leading to reduced investment in new generation projects or retirement of existing assets. 3. Market Competition: A well-functioning electricity market relies on competition among generators and retailers to deliver electricity to consumers at competitive prices. The supply-demand balance plays a central role in determining market prices through mechanisms such as wholesale auctions or bilateral contracts. 4. Resource Allocation: The supply-demand balance influences the allocation of resources across different energy sources and technologies. In markets where renewable energy sources like wind and solar are increasingly competitive, a balanced supply-demand dynamic can accelerate the transition towards a cleaner and more sustainable energy system. However, maintaining reliability and grid stability while integrating intermittent renewable energy sources requires careful planning and investment in complementary technologies such as energy storage, demand response, and grid modernization. As digitalization progresses and as organizations becomes more aware of the value of data generated by their connected assets, and in response transform their processes accordingly, AI can help deal with these large volumes of data and unlock value in the entire chain, from data creation to better decision making. This case study paper explores how Artificial Intelligence (AI) is used to balance supply and demand the context of a rapidly changing grid, changing of customer behaviours and active citizen participation.
- 8. UNECE Task Force on Digitalization in Energy Case study “Balancing the electricity supply and demand with Artificial Intelligence” 7 Contextual introduction: what is Artificial Intelligence? AI systems can vary in their levels of autonomy and adaptiveness once deployed. One definition for AI is: “An AI system is a machine-based system that, for explicit or implicit objectives, infers, from the input it receives, how to generate outputs such as predictions, content, recommendations, or decisions that can influence physical or virtual environments” 3 An AI system is a machine-based system that, for explicit or implicit objectives, infers, from the input it receives, how to generate outputs such as predictions, content, recommendations, or decisions that can influence physical or virtual environments. As such, Artificial Intelligence is a broad field that includes the areas of Machine Learning, Deep Learning, and more recently, Generative AI. Sometimes these terms are used interchangeably to describe systems with intelligent behaviour. Figure 1 shows the nesting of the categories of AI. 4 Figure 1 - A nested taxonomy for Artificial Intelligence technologies As climate change is one of the greatest challenges facing humanity, machine learning (ML) can be a powerful tool in reducing greenhouse gas emissions and helping society adapt to a changing climate. “Since variable generation and electricity demand both fluctuate, they must be forecast ahead of time to inform real-time electricity scheduling and longer-term system planning. Better 3 EU Artificial Intelligence Act, https://artificialintelligenceact.eu/the-act/, final draft (2024). 4 For a more generalized overview on AI, with detailed descriptions and its technological impact on the electric grid, see: Impact of Artificial Intelligence on the Digital and Data Transformation in the Electricity Sector (ECE/ENERGY/GE.6/2024/3, ECE/ENERGY/GE.5/2024/3), https://unece.org/sed/documents/2024/07/workingdocuments/impact-artificial-intelligence-digital-and-data Artificial Intelligence Machine Learning Deep Learning Generative AI
- 9. UNECE Task Force on Digitalization in Energy Case study “Balancing the electricity supply and demand with Artificial Intelligence” 8 short-term forecasts can allow system operators to reduce their reliance on fossil-based standby plants and to proactively manage increasing amounts of variable sources. Better long-term forecasts can help system operators (and investors) determine where and when variable plants should be built. While many system operators today use basic forecasting techniques, forecasts will need to become increasingly accurate, span multiple horizons in time and across registered assets, and better quantify uncertainty to support these use cases. ML can help on all these fronts.”5 5 Tackling Climate Change with Machine Learning. ACM Comput. Surv. 55, 2, Article 42 (February 2022), 96 pages. Section 2.1.1 https://doi.org/10.1145/3485128
- 10. UNECE Task Force on Digitalization in Energy Case study “Balancing the electricity supply and demand with Artificial Intelligence” 9 Short-term load demand forecasting: use of Artificial Intelligence at Hydro-Québec (Canada) Hydro-Québec (HQ) is the utility of the province of Québec, Canada. It operates some 60 hydroelectric generating station, making it one of the largest hydroelectricity producers in the world. Close to 100% of its electricity is generated using water thus with very low greenhouse gas emissions and no toxic waste. Transmission system of HQ is the most extensive in North America and includes over 34,000 km of high-voltage lines running from large generating stations in remote areas to the province’s more populated areas. It delivers electricity through its distribution lines, which crisscross the province. Its transmission and distribution lines extend for some 260,000 km. Context Short-term load demand forecasting (few hours to a few days horizon) is a key activity for HQ that is highly dependent on climatic and economic conditions. It is necessary for purposes as varied as generation management, reliability, maintenance of the power grid and sales positioning on foreign markets. It is therefore an activity that is crucial for the resilience of our activities in the context of climate change and the energy transition. The traditional legacy models that have been used for decades by HQ to forecast load demand, offer, on average, good performances. These models are built on a base of proven mathematical algorithms whose hundreds of parameters must be adjusted regularly. The objective is to individually model the physical or human phenomena influencing the behaviour of the power grid on a large scale (e.g. heating vs. cooling, weekdays vs. weekend, lighting in cities). These models were patiently developed by specialists over several years based on a detailed knowledge of the behaviour of power grid of HQ based on electrical and climate data. However, under certain conditions, there is now a trending upward gap between the prediction of these legacy models and the reality, which can result in a risk to the reliability of the power grid and/or loss of sales opportunities for the company. This gap – which has widened in recent years – can be explained by the rather fast changing behaviour of the power grid induced by societal changes (industry transformation, bitcoin mining companies, data centres, urban sprawl, home automation, teleworking, etc.) and by climate change (increase in average temperatures and the frequency of extreme weather events, including heat waves outside the summer period). These changes greatly complicate the maintenance and evolution of these legacy models since it requires rethinking the design parameters on which these models are based and sometimes manually adjusting the algorithmic parameters in the context of real-time operation. This deterioration is also likely to increase with the advent of the energy transition in response to the climate crisis and the need to reduce greenhouse gas emissions. As cities move towards electrification (electric vehicle charging, dynamic pricing, switching heating and industrial processes to electric, etc.) the need for accurate load demand forecasting will increase in a
- 11. UNECE Task Force on Digitalization in Energy Case study “Balancing the electricity supply and demand with Artificial Intelligence” 10 context of energy resources limited by deployment and installation of new centralized and decentralized means of generation. In this context of complex and rapid transformations, which creates a significant number of unknowns, the requirement for specialists to constantly maintain (i.e., update) these models is infeasible and unsustainable. This is why HQ has been relying for several years on the use of the latest technological advances in AI and big data processing to maintain and improve the accuracy of short-term load demand forecasting. Innovation AI based on deep neural networks seems particularly suited to this context of rapid transformation because the AI algorithm is then delegated the responsibility of building the equivalent of the modelling functions database of legacy models built by specialists over several years. This allows for a quick and effective response where old technology struggles to keep up with the breakneck pace imposed by the transformation of electricity consumption and climate change. Thus, where specialists have taken several decades to build a few models in the context of a relatively stable power grid – models that are also difficult to maintain over time because of their intrinsic technological limitations to old computer programming languages and the loss of skills (via natural attrition or turnover) – the AI algorithm can build many new models and then automate the selection decision to determine the appropriate calculation and post-processing resources. Also, while legacy models will not automatically take into account new corrective factors (e.g. the COVID-19 pandemic), which can make historical consumption data obsolete, deep neural network-based AI models have the ability to automatically understand, through their training, the existence of particular more or less short periods in historical consumption data such as the pandemic period of COVID-19 as well as longer-term trends such as the gradual increase in average temperatures inherent to global warming. It is therefore a complete paradigm shift in the activity of forecasting load demand for the modeler and forecaster: humans no longer seek to understand the details of models but seek to guide the multiple AI trainings, to refine the architecture of deep neural networks and to manipulate the massive data intended for training or resulting from it. In return, the modeler and forecaster can have the ability to anticipate the creation of models adapted to different scenarios simulating the future behaviour of the power grid at a very early stage. And it can create more robust models to meet security objectives in the context of a cyberattack, for example. For all these reasons, since 2018, HQ has developed a suite of tools dedicated to the creation and operation of AI-based load demand forecasting models. This research and development (R&D) action was carried out by maintaining a close link between research centre of HQ and the team in charge of operating load demand forecasting models, and then by setting up the associated computing resources within the cloud computing laboratory team.
- 12. UNECE Task Force on Digitalization in Energy Case study “Balancing the electricity supply and demand with Artificial Intelligence” 11 This constant link between the research laboratory and the business unit has helped to reduce the time to market of AI while constantly ensuring the robustness and security of the new AI tools put into operation in a secure environment under real-time constraints. It should also be noted that this suite of AI tools makes it possible to create load forecasting models from both a macroscopic (also referred as "top-down") and microscopic (known as "bottom-up") point of view. The difference between these approaches lies in the type of data used. The macroscopic data will use aggregated consumption data from thousands of power meters spread across the province, while the microscopic data will use data from millions of smart meters. Commissioning It is in this changing context that a significant event took place at 10:03 on 23 October 2023, at HQ: for the first time, the short-term load demand forecast was officially calculated using deep neural network-based AI models (top-down approach) developed and implemented by a team of researchers and experts from the company. This use of deep neural network-based AI models is believed to be a first in the context of 24/7 + real-time + security zone. The parameters of these AI models were optimized during a test campaign carried out in 2023 by the experts of the team in charge of operations on several hundred processors during which more than 11,000 models were evaluated to select a dozen that were combined to build this new AI load demand forecasting model. This feat is even more remarkable given that it is the first new load demand forecasting model to be implemented and used in operation since 2004 at HQ. This event also highlighted the ability of the operations team to adapt, as they had to take control of a completely new technology with High Performance Computing (HPC) in a cloud computing laboratory that did not exist at the beginning of the project (2018) while maintaining operations under real-time constraints. This underlines the importance of establishing long-term links between research and business units with progressive development objectives that are validated step by step. Further, as research and business units adapt to new requirements from end-users and systems, the need for human capital upskilling becomes increasingly necessary.
- 13. UNECE Task Force on Digitalization in Energy Case study “Balancing the electricity supply and demand with Artificial Intelligence” 12 Other uses of Artificial Intelligence in the balancing of generation and demand This section highlights some other application of AI in the context of balancing generation and demand, and relevance to other regions. Building management In the United Arab Emirates (UAE), significant advancements have been made in applying AI to building management systems, particularly in Dubai and Abu Dhabi. AI is used to monitor and control building environments, optimizing energy use for heating, ventilation, air conditioning (HVAC), and lighting based on occupancy and individual preferences. Smart sensors and AI algorithms analyse user behaviour and environmental data to adjust settings, significantly reducing energy consumption and carbon emissions. This technology aligns with the Green Building Regulations and Specifications of UAE and sets a standard for future developments in sustainable building practices in the Emirates. Many solutions and companies offer solutionsfor smart building management and many policies target the management of load. Renewable energy and storage As UAE diversifies its energy sources, AI plays a pivotal role in the development and optimization of renewable energy systems. The country is exploring AI to enhance the efficiency and reliability of solar and wind power installations. For example, AI algorithms are being used to predict solar irradiance and optimize the positioning of solar panels throughout the day, maximizing energy capture and significantly boosting overall system productivity. Mohammed bin Rashid Al Maktoum Solar Park: The solar park in Dubai utilizes AI-based technology to optimize the performance of solar panels. By analysing data from weather sensors and other sources, the system predicts solar radiation levels and adjusts the angle of the solar panels accordingly, increasing energy production and efficiency. The country also plans to leverage AI to facilitate real-time energy management in wind farms by predicting wind patterns and adjusting turbine angles to optimize power generation. This not only increases the energy output but also extends the lifespan of the equipment by reducing unnecessary wear and tear. The integration of AI in the country also extends to energy storage systems, where it manages the charge and discharge cycles of batteries in solar plants, enhancing energy storage efficiency and stability. This is crucial for maintaining a steady supply of energy, especially during peak demand times or in adverse weather conditions. The digital arm of the Dubai Energy and Water Authority (DEWA) is set to use autonomous systems for renewable energy and storage, being one of the first utilities in the region to do so. These AI-driven initiatives in renewable energy are part of the broader strategy of UAE to reduce its carbon footprint and lead in the global energy transition, marking significant steps towards achieving energy sustainability and resilience.
- 14. UNECE Task Force on Digitalization in Energy Case study “Balancing the electricity supply and demand with Artificial Intelligence” 13 Grid management The commitment of UAE to AI in renewable energy is also seen in its investments in smart grid technology. Smart grids, enhanced with AI, are essential for managing the complexities of distributing multiple energy sources. They not only improve operational efficiencies but also support the transition towards a more sustainable and decentralized energy model. AI will be a key tool to optimize grid operations and to enhance the capacity of existing transmission and distribution lines, as well as for extending the lifetime of existing equipment in the UAE. In Dubai, the state-owned utility DEWA has deployed a smart grid system that uses AI to predict electricity demand and manage energy supply. The system analyses data from smart meters and other sources to identify patterns and predict consumption, allowing the utility to optimize the distribution of energy and reduce the risk of blackouts or other disruptions. AI can also support the grid integration of renewable sources. It helps manage the variability and intermittency of renewable energy, ensuring a stable and reliable power supply. By analysing vast amounts of data from grid operations and weather forecasts, AI systems can predict energy demand and adjust power distribution accordingly.
- 15. UNECE Task Force on Digitalization in Energy Case study “Balancing the electricity supply and demand with Artificial Intelligence” 14 Lessons learned While the integration of AI brings considerable benefits, it also presents challenges and a few lessons learned. From the use on short-term demand forecasting and the other applications, the adaptation (training, development, recruitment) of the workforce to new technologies is identified as one of the top challenges. HQ has teamed with the research centre and exposed its operational resources to the new technology and participate actively in the development of the model. The UAE is trying to address these challenges by emphasizing education and training, preparing its workforce for the evolving technological landscape. This approach can help mitigate potential disruptions in traditional work processes and fosters a culture receptive to innovation. In addition to the introduction of AI and models, the introduction of new technology, like HPC in a cloud computing, not widely used in the context of the electric grid and the ability to gain sufficient understanding and confidence in this technology is also a challenge. An additional lesson learned is to always have in mind the portability of the AI tools between different systems to be able to easily switch from the cloud laboratory to the secure operating area. At HQ, the following elements were part of the “step-by-step” approach and the ongoing research / operational teams link put in place: 1. Building a cloud-based laboratory that is not dependent on “high-level” cloud services in its “core”. This approach allowed HQ operational team to easily switch the new AI tools from the laboratory to the operating area because it did not have to implement “high” level services from the cloud provider, services that would have been caused technical issues or non-compliance with the applicable standards. This highlights the strategic importance to remain independent of the cloud provider as much as possible. 2. Cloning production machines in the cloud laboratory - a kind of reverse process from the previous point – to test tools out of the secure zone very upstream of their implementation in the secure zone but in the “real” conditions of the secure zone. Deployment of new technology and new models while maintaining operations under real-time constraints must also be considered. This underlines the importance of establishing long-term links between research and business units with progressive development objectives that are validated step by step. As outlined in the UAE National Strategy for Artificial Intelligence 2031, the country is actively exploring further potential of AI in renewable energy technologies and smart grid applications, aiming to lead in the global transition to sustainable energy systems. Signalling the technology’s importance within various sectors, UAE also has a specific Minister of State for Artificial Intelligence. The UAE strategic approach to integrating AI in the energy sector highlights its commitment to enhancing operational efficiencies and environmental sustainability. Through robust investment
- 16. UNECE Task Force on Digitalization in Energy Case study “Balancing the electricity supply and demand with Artificial Intelligence” 15 in technology and human capital, the UAE aims to address immediate operational needs while setting the stage for future advancements in energy management.
- 17. UNECE Task Force on Digitalization in Energy Case study “Balancing the electricity supply and demand with Artificial Intelligence” 16 Conclusion and policy recommendations This paper has shown both potential and concrete use of AI in the critical context of balancing load and demand in the electric grid. The digitalization of the electric grid unlocks multiple opportunities, including enhanced system operation and planning with advanced measurement and monitoring systems, holistic forecasts and predictive maintenance, demand-side opportunities, and optimized cyber security systems. With the availability of rich and reliable data, AI can help deal with these large volumes of data and unlock value in the entire chain, from data creation to better decision making. From the lessons learned identified in the previous section, governmental authorities and regulators could support the adoption of AI to favour better decision making that would help managing the increasing complexity of the electric grid, the integration of renewables and distributed energy resources and a cleaner electricity system with policies and regulations: • To support digitalization of the grid • To support the adaptation (training, development, recruitment) of the workforce to new technologies and to the use of AI • That favour innovation, development of new technologies, use of AI and its deployment. Policy can help accelerate the use of AI technologies through actions such as: • Develop a (national) strategy for AI that would include guidelines and safeguards on data use, privacy and ethic. • Provide robust investment in technology and human capital, to address immediate operational needs and future developments. • Better end market definitions for how renewables and storage can be incorporated and monetized. Setting reasonable expectations around timing, location and creating monetary products and economic opportunities around ancillary services and grid resilience that are applicable closer to the consumers (i.e., behind-the-meter) can help to better parameterize the forecasting models.
Related Jaunts
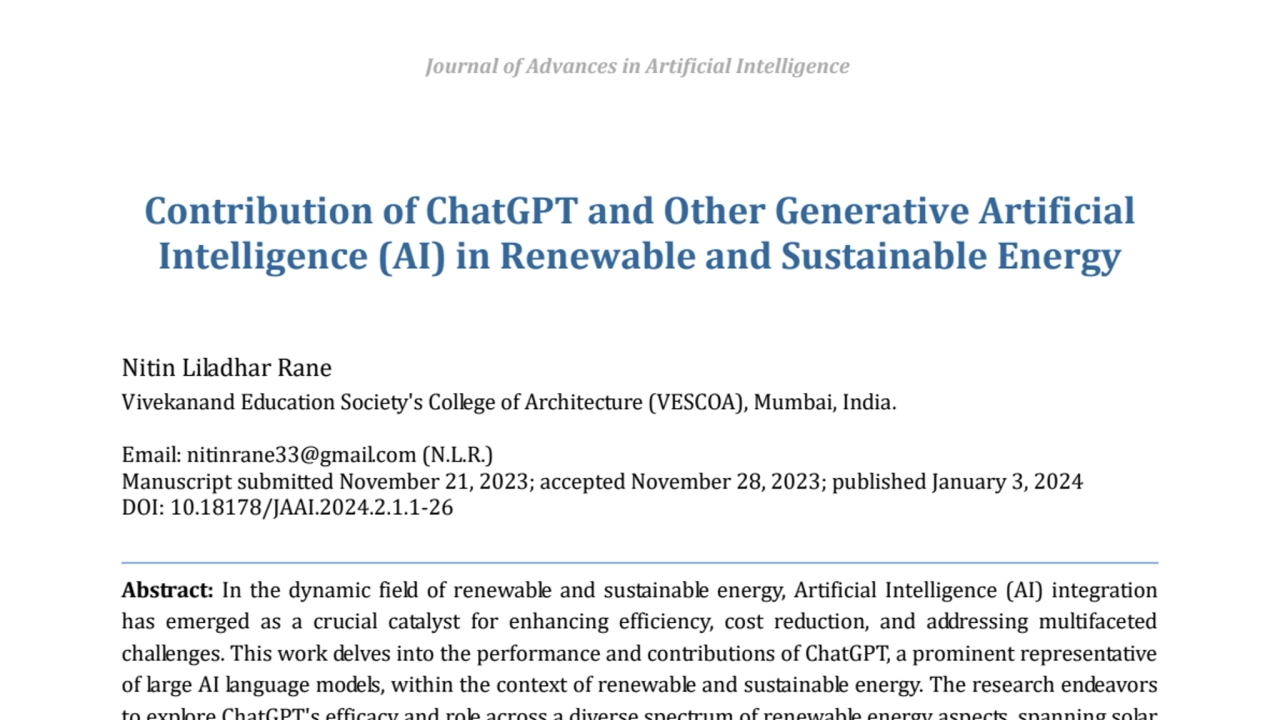
Chat GPT uses 10 times as much Energy as Google Search -Energy Consumption Increase
@Blockchainboss
4 months ago •
292 views
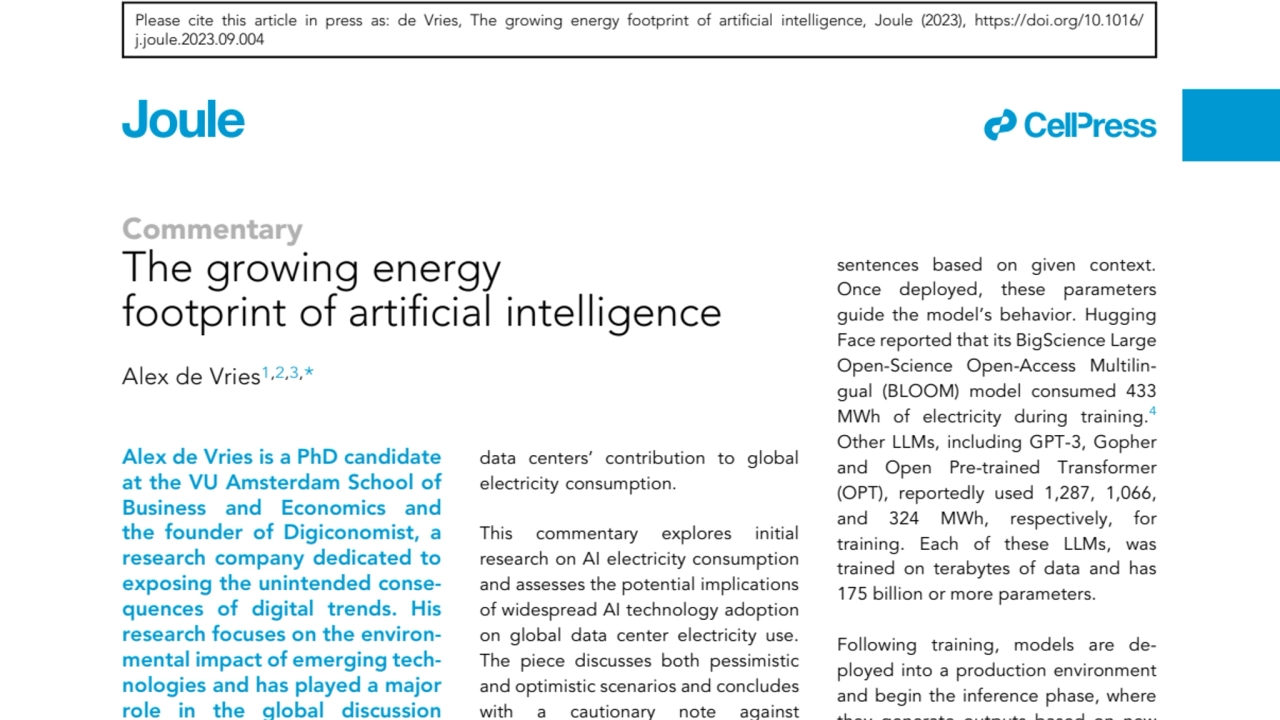
AI's Increasing Energy Consumption and demand for electricity
@Blockchainboss
4 months ago •
392 views
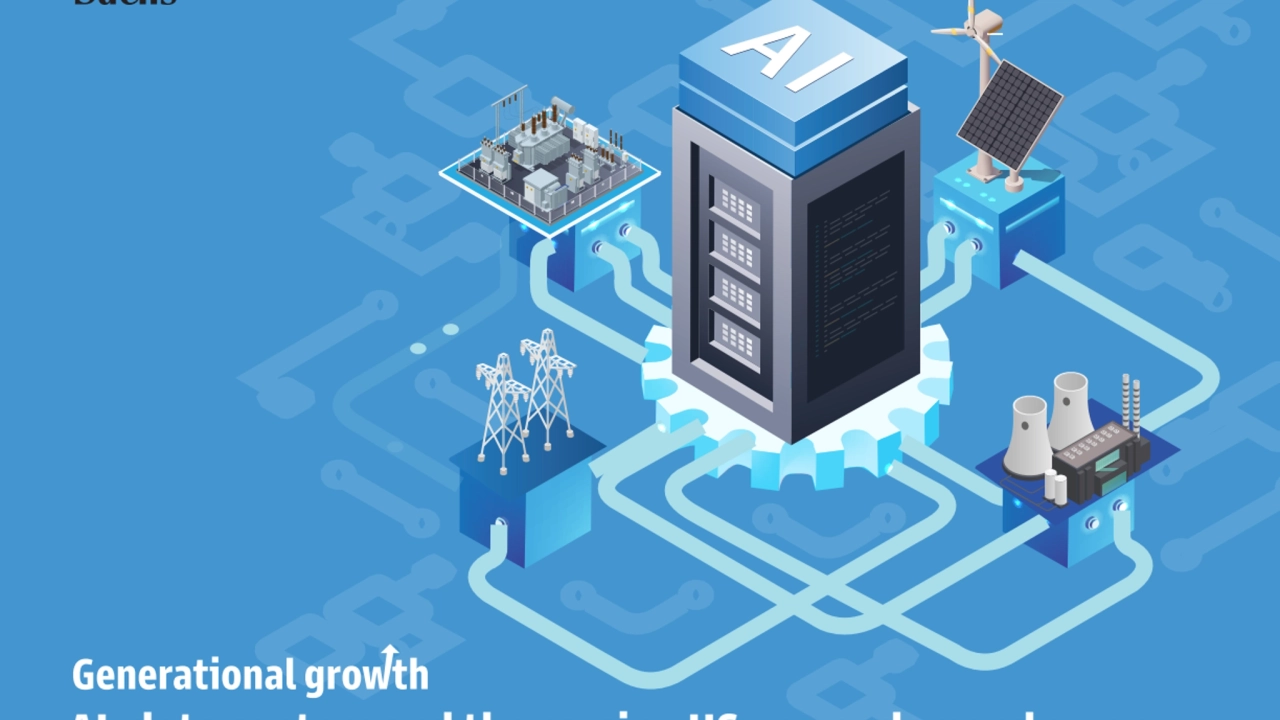
Generational Growth AI, data centers and the coming US power demand surge
@financepresentations
4 months ago •
360 views
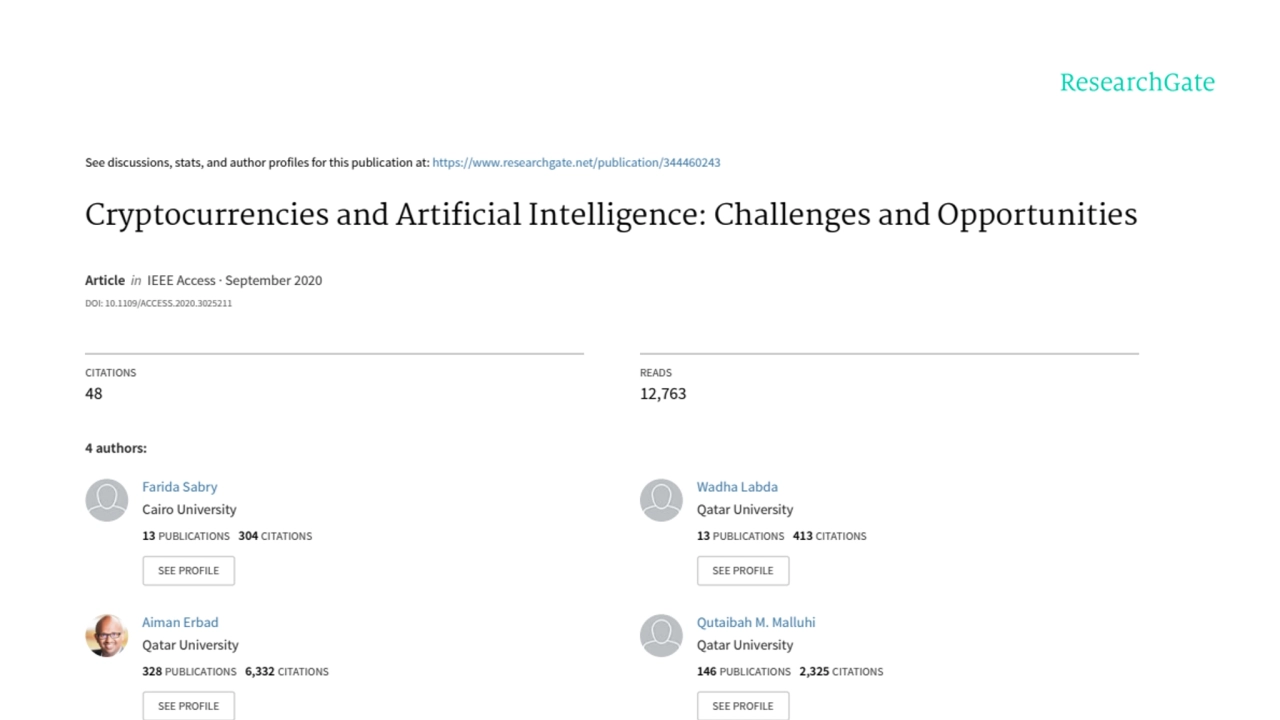
Cryptocurrencies and Artificial Intelligence: Challenges and Opportunities
@Blockchainboss
4 months ago •
368 views
More from author
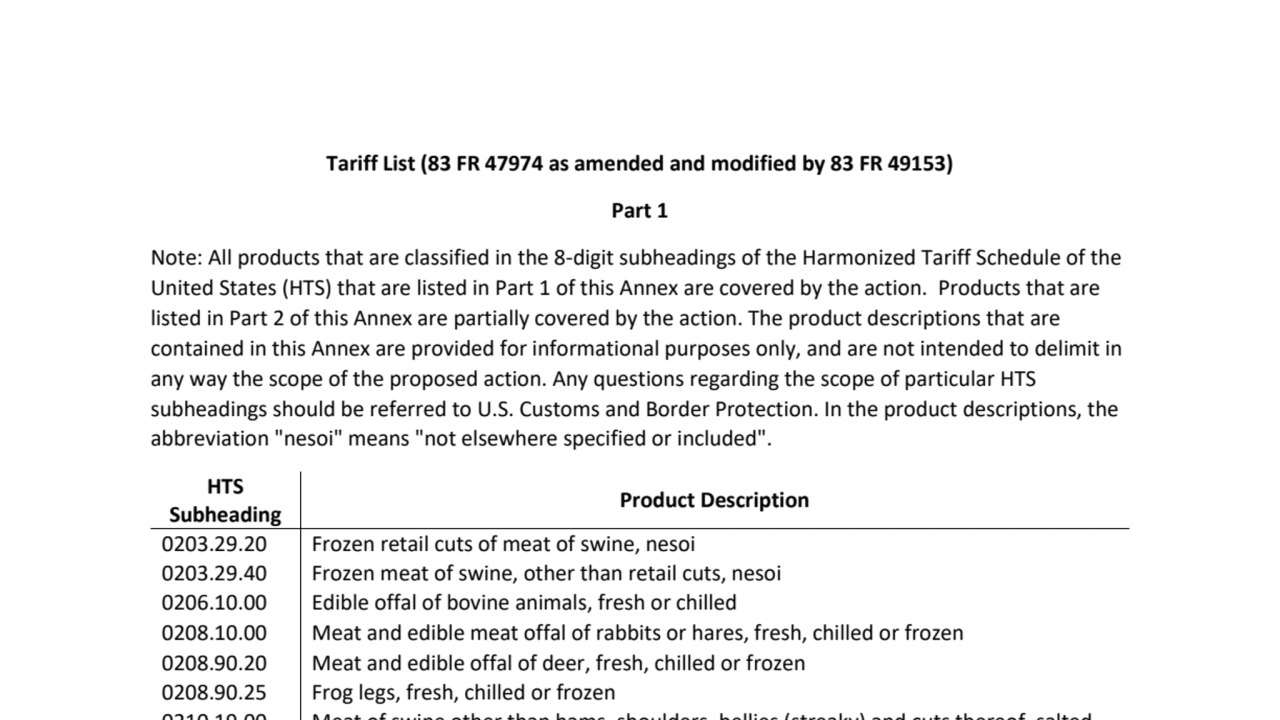
Tariff List (83 FR 47974 as amended and modified by 83 FR 49153)
@Blockchainboss
2 months ago •
153 views
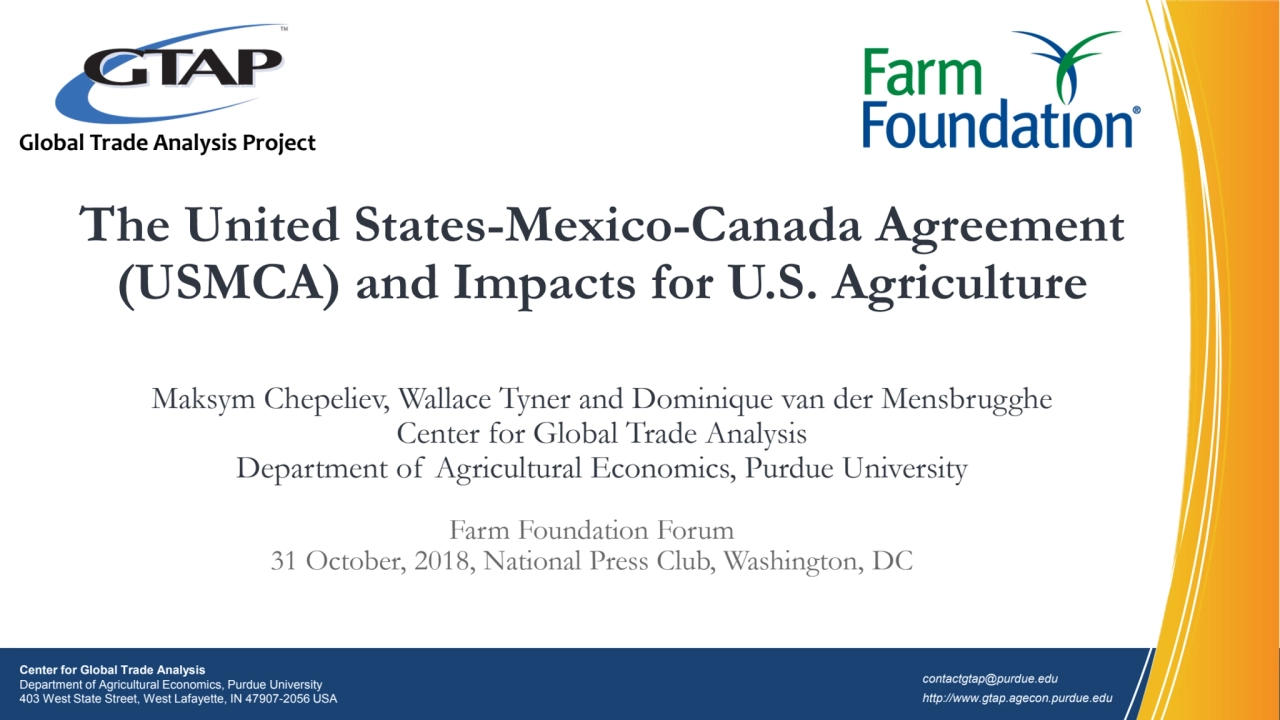
The United States-Mexico-Canada Agreement and Impacts for U.S. Agriculture
@Blockchainboss
2 months ago •
160 views
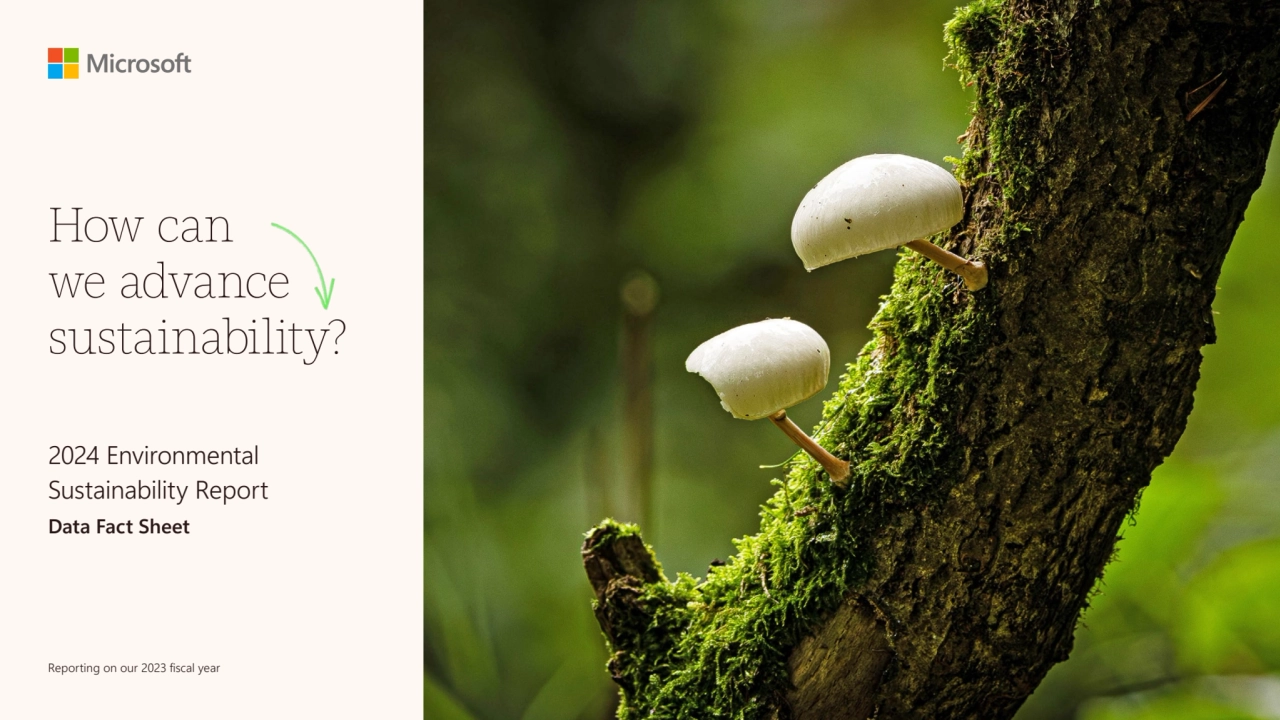
Microsoft 2024 Environmental Sustainability Report Data Fact Sheet
@Blockchainboss
3 months ago •
383 views