Chat GPT uses 10 times as much Energy as Google Search -Energy Consumption Increase
AI Summary
Key Insights
- AI, especially through generative models like ChatGPT, is shown to enhance efficiency and innovation across renewable energy sectors including solar, wind, biomass, hydropower, and geothermal.
- AI facilitates optimization in diverse areas such as solar panel placement, wind turbine maintenance, and biomass feedstock selection, leading to more efficient energy production and resource management.
- The research underscores AI's contribution to grid integration, energy storage, and the development of smart grids, ensuring a more stable and dependable energy supply from renewable sources.
- AI models like ChatGPT aid in data analysis, policy recommendations, and public education, promoting informed decision-making and increased adoption of renewable energy technologies.
- Ethical and environmental implications of AI deployment in the energy sector are discussed, emphasizing the need for responsible integration to maximize benefits and minimize potential risks.
![Contribution of ChatGPT and Other Generative Artificial
Intelligence (AI) in Renewable and Sustainable Energy
Nitin Liladhar Rane
Vivekanand Education Society's College of Architecture (VESCOA), Mumbai, India.
Email: nitinrane33@gmail.com (N.L.R.)
Manuscript submitted November 21, 2023; accepted November 28, 2023; published January 3, 2024
Abstract: In the dynamic field of renewable and sustainable energy, Artificial Intelligence (AI) integration
has emerged as a crucial catalyst for enhancing efficiency, cost reduction, and addressing multifaceted
challenges. This work delves into the performance and contributions of ChatGPT, a prominent representative
of large AI language models, within the context of renewable and sustainable energy. The research endeavors
to explore ChatGPT's efficacy and role across a diverse spectrum of renewable energy aspects, spanning solar
energy, wind energy, biomass energy, hydropower, geothermal energy, energy storage, grid integration,
energy efficiency, materials science, policy, economics, environmental impact, energy accessibility, and
advanced biofuels. The investigation commences with solar energy, where ChatGPT showcases impressive
capabilities in data analysis, modeling, and forecasting. It facilitates solar panel placement optimization,
energy production prediction, and overall efficiency enhancement. Likewise, in the realm of wind energy,
ChatGPT proves invaluable for tasks such as wind turbine control, maintenance scheduling, and wind speed
prediction. In biomass energy, ChatGPT aids in feedstock selection, process optimization, and emissions
reduction. In the hydropower sector, it supports reservoir management and river flow prediction, thereby
optimizing energy output. Geothermal energy benefits from ChatGPT's geological analysis, reservoir
modeling, and exploration strategies. Energy storage solutions are indispensable for grid stability, and
ChatGPT contributes to the advancement of cutting-edge battery technologies, augmenting energy storage
capacity and lifespan. Furthermore, ChatGPT's insights foster the evolution of smart grids, ensuring efficient
energy distribution and effective demand management. Additionally, ChatGPT provides invaluable insights
into policy and economics, offering recommendations for incentivizing the adoption of renewable energy
sources while considering environmental impact and sustainability. It also contributes to the advancement of
tidal and wave energy technologies, as well as the development of advanced biofuels. The outcomes of this
research paper underscore the potential of AI and ChatGPT in shaping the future of renewable energy,
expediting progress toward a sustainable energy ecosystem.
Keywords: ChatGPT, artificial intelligence, generative AI, large language models, renewable energy,
sustainable energy
1. Introduction
In an age marked by unprecedented technological progress, the significance of artificial intelligence (AI)
has grown substantially in addressing the world's most urgent challenges [1–4]. Among these challenges, the
pursuit of sustainable and renewable energy sources emerges as a critical mission to combat climate change
and ensure the long-term health of our planet [5–7]. This research paper explores the role and performance
of advanced generative AI models like ChatGPT in the realm of renewable and sustainable energy. The rise of
Journal of Advances in Artificial Intelligence
1 Volume 2, Number 1, 2024
DOI: 10.18178/JAAI.2024.2.1.1-26](https://d2z384uprhdr6y.cloudfront.net/xMzRKA7VaWD_354QjXp8JwZgi4pCZRVuqhAnLmIjJKQ/rt:fill/q:100/w:1280/h:0/gravity:sm/czM6Ly9qYXVudC1wcm9kdWN0aW9uLXVwbG9hZHMvMjAyNC8xMi8yNS8xMDE0ZTQ0OC03ZGViLTQyODMtYjRkMi03MDRlMGQ5OTI0Mzcvc2xpZGVfMS1sLndlYnA=.webp)
![such large language models has opened new avenues for enhancing our comprehension, decision-making,
and innovation in this field. By scrutinizing their capabilities and limitations in comparison to other AI
approaches, we aim to uncover the transformative potential of AI in expediting the transition to a greener
and more sustainable energy landscape. The global energy landscape is undergoing a profound shift, driven
by the urgent need to curtail greenhouse gas emissions and mitigate climate change's effects [8]. This
transformation encompasses a diverse range of energy sources and technologies, including solar and wind
energy, hydropower, geothermal energy, and advanced biofuels [9]. It also involves complex aspects such as
energy storage, grid integration, energy efficiency, materials science, policy and economics, environmental
impact, energy access, and equity. As we embark on this transformative journey, harnessing AI's power,
especially through generative AI models like ChatGPT, becomes crucial to optimize, innovate, and accelerate
the development and deployment of sustainable and renewable energy solutions.
Solar energy is a promising and abundant renewable power source with the potential to revolutionize our
energy landscape [10]. AI can boost the efficiency of photovoltaic systems, optimize the placement of solar
panels, and forecast solar irradiance more accurately. Similarly, wind energy benefits from advanced AI
algorithms that optimize turbine placement, predict wind patterns, and enhance maintenance procedures,
ensuring a more reliable and efficient energy source. Biomass energy, hydropower, and geothermal energy
also gain from AI applications that optimize plant operations and resource allocation for sustainable energy
production. Energy storage plays a pivotal role in enabling renewable energy sources to mitigate
intermittency and ensure a stable power supply [11]. AI-driven algorithms can optimize energy storage
systems, maximizing their capacity and lifespan. Grid integration and the development of smart grids are
crucial components of a sustainable energy future, and AI can manage the intricate dynamics of these
systems. Furthermore, AI-driven advancements in energy efficiency, materials science, and nanotechnology
have the potential to reduce energy consumption and enhance the performance of renewable energy
technologies. Fig. 1 shows the co-occurrence analysis of the keywords.
Fig. 1. Co-occurrence analysis of the keywords.
AI also profoundly influences the policy and economics surrounding renewable energy [12, 13]. AI can
Journal of Advances in Artificial Intelligence
2 Volume 2, Number 1, 2024](https://d2z384uprhdr6y.cloudfront.net/yxZ1LF7M0SKDyPmYS7iv75XSZ7xIpeE_kmoA4tInz0w/rt:fill/q:100/w:1280/h:0/gravity:sm/czM6Ly9qYXVudC1wcm9kdWN0aW9uLXVwbG9hZHMvMjAyNC8xMi8yNS8xMDE0ZTQ0OC03ZGViLTQyODMtYjRkMi03MDRlMGQ5OTI0Mzcvc2xpZGVfMi1sLndlYnA=.webp)
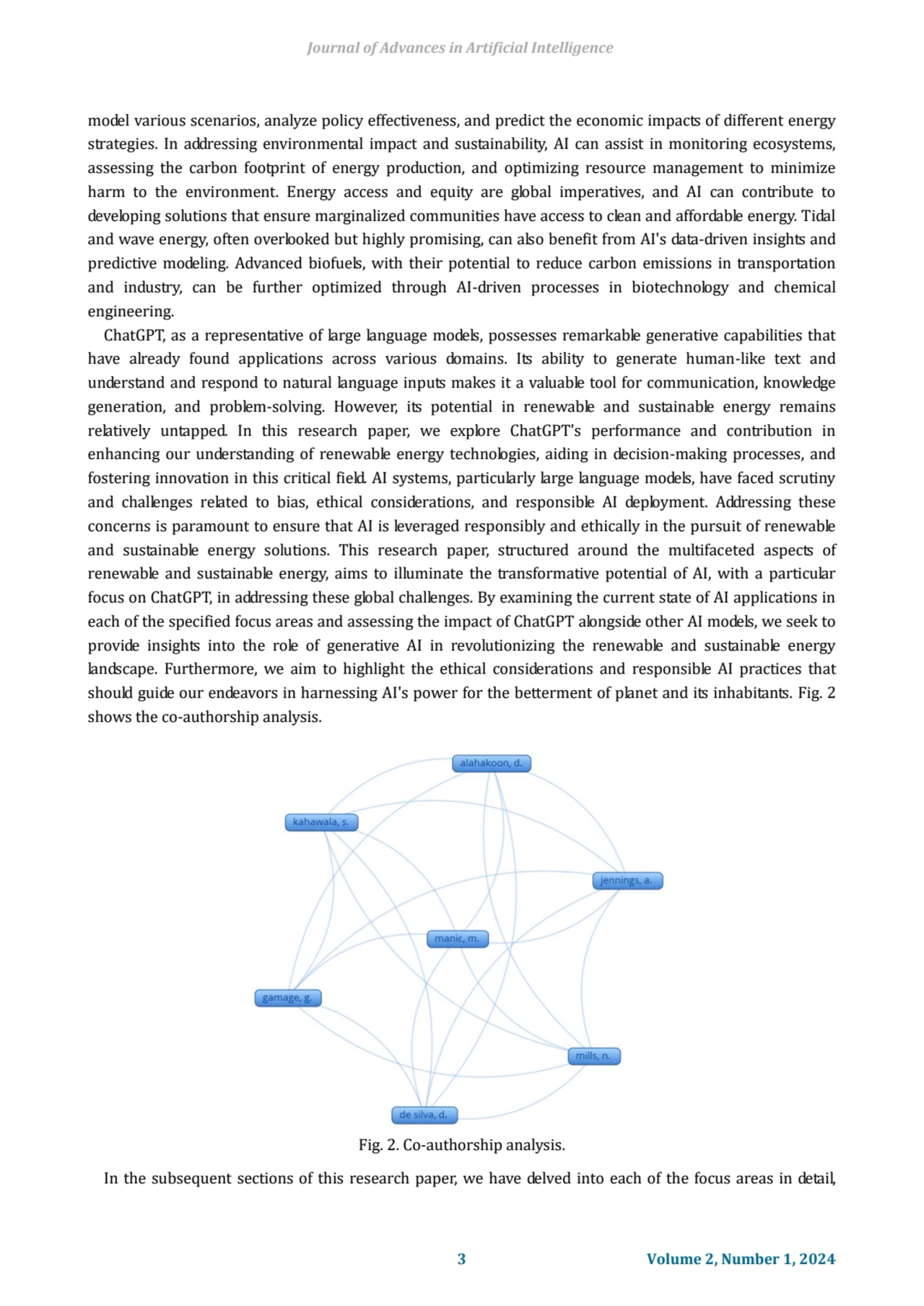
![exploring the contributions and potential of AI models like ChatGPT. Additionally, we have delved into the
ethical and responsible AI considerations that must accompany these advancements. Through this
comprehensive analysis, we aspire to provide a holistic perspective on the symbiotic relationship between AI,
renewable energy, and the sustainable future we all strive to achieve.
2. Contribution of ChatGPT in Solar Energy
Generative artificial intelligence (AI) systems, such as ChatGPT, possess the capacity to make substantial
contributions to the advancement of solar energy as a renewable and sustainable power source [14]. Solar
energy harnesses the sun's energy to generate electricity and heat, offering a multitude of environmental and
economic advantages [15, 16]. AI technologies can play a pivotal role in elevating the efficiency, dependability,
and affordability of solar energy systems.
Enhancing solar panel placement and design:
One of the primary challenges in solar energy generation is the optimization of solar panel placement and
design to maximize energy output [17]. AI, including generative models like ChatGPT, can facilitate this
process. By scrutinizing geographical data, weather patterns, and local shading variables, AI can propose
ideal sites for solar panel installations. Furthermore, AI can contribute to the creation of tailored solar panel
configurations that consider unique architectural constraints and aesthetic preferences, thereby increasing
the appeal of solar adoption for homeowners and businesses.
Predictive maintenance and fault detection:
AI can elevate the reliability of solar energy systems by means of predictive maintenance and fault
detection [18]. Solar panels are subjected to various environmental stressors, leading to wear and tear over
time. Generative AI can evaluate historical and real-time data from solar installations to predict maintenance
needs, thereby reducing downtime and elevating energy production. Additionally, AI-powered algorithms can
swiftly pinpoint and diagnose issues in solar panels, enabling technicians to promptly address them,
minimizing energy losses, and extending equipment lifespan.
Energy forecasting and grid integration:
The effective integration of solar energy into the power grid necessitates precise energy forecasting [19,
20]. ChatGPT and comparable AI models can scrutinize historical weather data, solar panel performance data,
and other pertinent information to forecast solar energy generation with exceptional accuracy. This
information is invaluable for grid operators, allowing them to efficiently balance energy supply and demand.
AI can also assist in optimizing the grid's response to fluctuations in solar generation, thereby ensuring a
stable and dependable energy supply.
2.1.Energy Consumption Optimization
Generative AI can be applied to optimize energy consumption in residential, commercial, and industrial
settings [21, 22]. ChatGPT can contribute to the development of personalized energy management strategies,
taking into account factors such as user schedules, energy-efficient appliances, and real-time electricity
pricing. This can result in reduced energy costs, reduced reliance on fossil fuels, and an overall more
sustainable energy consumption pattern.
Advancing solar materials and technologies:
AI can expedite the development of cutting-edge solar materials and technologies [23]. By analyzing
extensive datasets and simulating various materials and designs, AI-driven research can pinpoint innovative
materials with superior efficiency and durability. Generative models like ChatGPT can assist in the design and
refinement of solar cells, aiding researchers in uncovering breakthroughs that render solar energy more
accessible and cost-effective.
2.2.Energy Storage Solutions
Journal of Advances in Artificial Intelligence 4 Volume 2, Number 1, 2024](https://d2z384uprhdr6y.cloudfront.net/nNiNAueBGSjMWQBR6T9RVMtAcnYre6PZxNfPC-s-S6E/rt:fill/q:100/w:1280/h:0/gravity:sm/czM6Ly9qYXVudC1wcm9kdWN0aW9uLXVwbG9hZHMvMjAyNC8xMi8yNS8xMDE0ZTQ0OC03ZGViLTQyODMtYjRkMi03MDRlMGQ5OTI0Mzcvc2xpZGVfNC1sLndlYnA=.webp)
![The intermittent nature of solar energy poses a challenge; which AI can help address through the
optimization of energy storage solutions like batteries [24]. Generative AI models can analyze historical
energy consumption patterns and environmental data to ascertain the most efficient size, type, and
configuration of energy storage systems. This ensures surplus solar energy is stored for use during overcast
days or at night, reducing the reliance on backup fossil fuel power generation.
2.3.Policy and Market Insights
AI can provide valuable insights into the policy and market dynamics surrounding solar energy. ChatGPT
can analyze legislative documents, market trends, and public sentiment to assist policymakers in making
informed decisions that bolster solar adoption. Additionally, AI can aid businesses in identifying market
opportunities and investment strategies related to solar energy, ultimately promoting growth in the sector.
2.4.Customer Engagement and Education
Effective communication plays a pivotal role in promoting the adoption of renewable energy sources.
Generative AI can be employed to generate engaging and personalized content for consumers, aiding them
in comprehending the advantages of solar energy and the steps involved in installation. AI-driven chatbots
can answer queries, provide real-time estimates of energy savings, and guide potential customers through
the decision-making process, making solar energy more accessible to a wider audience.
2.5.Environmental Impact Assessment
AI can also contribute to the sustainability aspect of solar energy by evaluating its environmental footprint.
Generative models can analyze data related to the manufacturing, installation, and operation of solar panels to
identify areas where resource efficiency and carbon footprint can be improved. This information can guide
the industry towards more sustainable practices, mitigating any negative environmental consequences
associated with solar energy production.
2.6.Data security and Grid Resilience
As solar energy systems become increasingly interconnected and reliant on digital infrastructure, AI can
play a pivotal role in ensuring data security and grid resilience [25]. ChatGPT and similar AI models can aid in
the development of robust cybersecurity protocols to safeguard solar installations from cyber threats.
Additionally, AI can simulate and analyze grid scenarios to identify vulnerabilities and recommend strategies
to enhance grid resilience against potential disruptions [26].
ChatGPT and generative AI technologies hold the potential to revolutionize the solar energy industry by
addressing critical challenges and fostering innovation. From optimizing solar panel placement and design to
enhancing energy forecasting, AI can enhance the efficiency, dependability, and affordability of solar energy
systems. Furthermore, AI can support research into advanced materials and technologies, facilitate policy
and market analysis, educate consumers, and assess the environmental impact of solar energy. As the world
strives to transition to more sustainable energy sources, the synergy between AI and solar energy promises a
greener and more sustainable future. Table 1 shows the role of ChatGPT and generative AI in solar energy.
Table 1. Contribution of ChatGPT and Generative AI in Solar Energy
Sr.
No.
Aspect of Solar
Energy
Contribution of ChatGPT and Generative AI Potential Benefits Challenges and
Considerations
1 Solar Panel Design - Generating innovative solar panel designs by
simulating various materials, shapes, and
configurations to maximize efficiency and
aesthetics.
- Increased energy
generation
- Improved aesthetics
and integration
- Material feasibility
- Manufacturing
constraints
2 Energy Production - Utilizing historical data and weather patterns - Reduced energy - Data accuracy
Journal of Advances in Artificial Intelligence 5 Volume 2, Number 1, 2024](https://d2z384uprhdr6y.cloudfront.net/B0StNgp0yegwj71MsSoBPmEmd0gzXvnxnzlP6VLC0SQ/rt:fill/q:100/w:1280/h:0/gravity:sm/czM6Ly9qYXVudC1wcm9kdWN0aW9uLXVwbG9hZHMvMjAyNC8xMi8yNS8xMDE0ZTQ0OC03ZGViLTQyODMtYjRkMi03MDRlMGQ5OTI0Mzcvc2xpZGVfNS1sLndlYnA=.webp)
![Forecasting to provide accurate solar energy production
forecasts, helping grid operators manage energy
supply and demand efficiently.
wastage
- Better grid stability
- Weather variability
3 Solar Farm
Optimization
- Optimizing the placement and orientation of
solar panels in large solar farms to maximize
energy output and minimize shading.
- Higher energy yields
- Land use efficiency
- Site-specific
considerations
- Cost of
reconfiguration
4 Efficiency
Enhancement
- Suggesting improvements in solar cell
materials and coatings to increase energy
conversion efficiency through materials science
simulations.
- Enhanced solar cell
performance
- Reduced
manufacturing costs
- Real-world
validation
- Time and resource
constraints
5 Maintenance and
Repairs
- Assisting in identifying and diagnosing issues
with solar panels through image analysis,
reducing downtime and maintenance costs.
- Minimized
downtime
- Cost savings
- Data privacy
- Sensor availability
6 Energy Storage - Recommending energy storage solutions
based on energy generation patterns and
demand forecasts, optimizing the use of
batteries or other storage technologies.
- Enhanced grid
reliability
- Lower energy costs
- Battery degradation
- Initial investment
7 Customer
Engagement
- Enhancing customer understanding of solar
technology and benefits through AI-generated
content, answering FAQs, and explaining
complex concepts.
- Increased adoption
rates
- Informed
decision-making
- Ethical use of AI
- Privacy concerns
8 Policy and
Regulations
- Analyzing and summarizing policy documents,
helping policymakers make informed decisions
regarding solar energy incentives and
regulations.
- Streamlined policy
development
- Reduced ambiguity
- Ethical
considerations
- Interpretation
nuances
9 Supply Chain
Optimization
- Optimizing the supply chain for solar
equipment, predicting demand fluctuations, and
suggesting inventory management strategies.
- Cost savings
- Improved
sustainability
- Data accuracy
- Supplier
relationships
10 Environmental
Impact
Assessment
- Assessing the environmental impact of solar
projects, considering factors like land use, water
usage, and emissions, to make informed
decisions on project planning.
- Sustainable project
development
- Regulatory
compliance
- Complex modeling
- Data availability
11 Energy Market
Integration
- Providing insights into integrating solar energy
into existing energy markets, considering
pricing models, grid integration, and market
dynamics.
- Market
competitiveness
- Grid stability
- Regulatory hurdles -
Market volatility
12 Research and
Development
- Generating hypotheses and suggesting
research directions for advancing solar
technology, aiding scientists and engineers in
their work.
- Accelerated
innovation
- Targeted research
efforts
- Scientific validation
- Resource allocation
13 Public Awareness
Campaigns
- Generating engaging and informative content
for educational initiatives and public awareness
campaigns to promote solar energy adoption.
- Increased public
support
- Behavior change
- Ethical messaging
- Cultural differences
14 Data Analysis - Analyzing large datasets of solar energy
production and consumption to identify trends,
anomalies, and optimization opportunities.
- Informed
decision-making
- Data-driven insights
- Data privacy
- Data quality
3. Role of ChatGPT in Wind Energy
Generative artificial intelligence, such as ChatGPT, holds the promise of making substantial contributions to
the renewable and sustainable energy sector, with a particular focus on wind energy [27, 28]. Wind energy
plays a pivotal role in the worldwide transition toward cleaner and more sustainable power generation [29].
The utilization of wind to produce electricity offers a multitude of environmental and economic advantages,
and AI-driven technologies stand poised to revolutionize wind energy production, rendering it more efficient,
cost-effective, and ecologically friendly. One primary avenue through which ChatGPT and similar generative
Journal of Advances in Artificial Intelligence 6 Volume 2, Number 1, 2024](https://d2z384uprhdr6y.cloudfront.net/XlEzNlUGPSfpFPM-Tx0y62XrhmPtHOLmVnsdpsuw-Sw/rt:fill/q:100/w:1280/h:0/gravity:sm/czM6Ly9qYXVudC1wcm9kdWN0aW9uLXVwbG9hZHMvMjAyNC8xMi8yNS8xMDE0ZTQ0OC03ZGViLTQyODMtYjRkMi03MDRlMGQ5OTI0Mzcvc2xpZGVfNi1sLndlYnA=.webp)
![AI models can impact the realm of wind energy is advanced forecasting and predictive modeling. Given that
wind energy generation is heavily contingent on weather conditions, especially wind speed and direction,
precise weather forecasts are essential for optimizing wind farm operations. Generative AI models, including
ChatGPT, have the capability to analyze extensive historical weather data, current atmospheric conditions,
and predictive models to generate highly accurate forecasts for wind energy production. These forecasts
empower wind farm operators to make informed decisions regarding electricity generation, thereby
reducing the intermittency typically associated with wind energy and enhancing grid stability.
Furthermore, ChatGPT can provide real-time insights and recommendations based on continuously
changing weather data. By processing this information, it can propose adjustments to turbine settings, such
as rotor speed and blade pitch, to maximize energy capture during fluctuating wind conditions. This dynamic
optimization can significantly boost the overall efficiency of wind energy production, rendering it a more
dependable and appealing source of renewable energy. Beyond forecasting, generative AI can also assist in
the design and layout of wind farms. The optimization of wind farm layouts is crucial for maximizing energy
generation while minimizing adverse environmental impacts. AI models can scrutinize terrain data, wind
patterns, and other geographic factors to suggest the most efficient placement of wind turbines within a
given area. By optimizing turbine placement, developers can reduce the land footprint of wind farms, mitigate
disruption to local ecosystems, and enhance overall energy output.
Moreover, ChatGPT can facilitate the maintenance and operation of wind turbines. Wind turbine
maintenance can be a costly and labor-intensive endeavor, often requiring routine inspections and repairs.
AI-driven predictive maintenance models can analyze sensor data from turbines, including factors such as
temperature, vibration, and oil quality, to predict when components may fail or require maintenance.
Proactively scheduling maintenance can reduce downtime and extend the lifespan of turbines, ultimately
yielding a higher return on investment for these renewable energy assets. Generative AI can also play a
pivotal role in optimizing energy storage systems, a critical aspect of managing the intermittent nature of
wind energy. AI models can scrutinize historical energy production data, demand patterns, and pricing
information to make informed decisions about when to store excess energy and when to release it into the
grid. This aids in balancing supply and demand, reducing wastage, and maximizing the economic feasibility of
wind energy projects. Furthermore, ChatGPT can contribute to the development of innovative wind turbine
designs and materials. AI-powered simulations can model and evaluate various turbine configurations, taking
into account factors like aerodynamics, material durability, and environmental conditions. By rapidly
iterating through design options, AI can assist engineers in identifying more efficient and cost-effective
solutions, potentially leading to the creation of more powerful and long-lasting wind turbines.
Another significant area where generative AI can make a substantial impact is in energy policy and
regulation [30, 31]. ChatGPT can assist in the analysis of extensive volumes of legislative documents, research
papers, and industry reports to provide insights into the latest developments in wind energy policy, regulatory
changes, and market trends. This information can be invaluable for policymakers in making informed
decisions concerning incentives, subsidies, and regulations that support the growth of wind energy as a
sustainable energy source. Moreover, generative AI can contribute to public awareness and education about
wind energy. ChatGPT can generate informative content, articles, and presentations to help elucidate the
advantages of wind energy, dispel misconceptions, and address concerns. By providing accurate and
accessible information, AI can play a pivotal role in shaping public perception and garnering support for
wind energy projects. Table 2 shows the role of ChatGPT and AI in Wind Energy.
Generative artificial intelligence, exemplified by ChatGPT, possesses the potential to transform the wind
energy sector across various critical domains. It can enhance the precision of wind energy production
forecasts, optimize wind farm layouts, streamline turbine maintenance and operation, aid in energy storage
Journal of Advances in Artificial Intelligence 7 Volume 2, Number 1, 2024](https://d2z384uprhdr6y.cloudfront.net/DDU6l2-Zm52ncwxORNY2LVC77KVPS2AmH5NkLvSSIzw/rt:fill/q:100/w:1280/h:0/gravity:sm/czM6Ly9qYXVudC1wcm9kdWN0aW9uLXVwbG9hZHMvMjAyNC8xMi8yNS8xMDE0ZTQ0OC03ZGViLTQyODMtYjRkMi03MDRlMGQ5OTI0Mzcvc2xpZGVfNy1sLndlYnA=.webp)
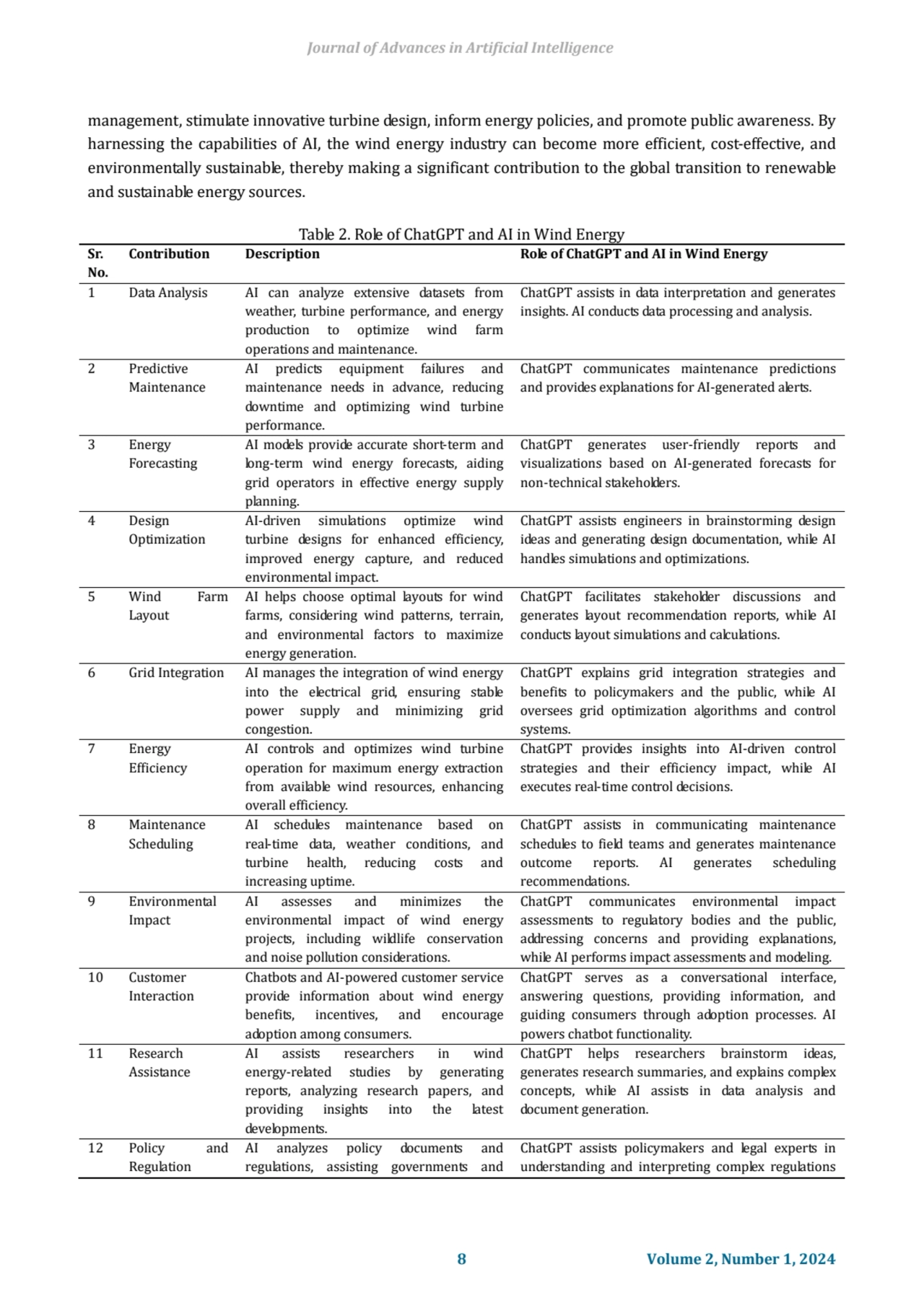
![organizations in informed decisions to
promote wind energy adoption and
sustainability.
and generates policy reports, while AI performs
regulatory analysis and compliance checks.
4. Contribution of ChatGPT in Biomass Energy
Generative artificial intelligence, exemplified by ChatGPT, possesses significant potential to advance the
utilization of biomass energy as a sustainable and renewable energy source [32]. Biomass energy is
harnessed from organic materials such as wood, agricultural residues, and waste products, rendering it a
valuable renewable energy resource with the capacity to mitigate greenhouse gas emissions and reduce
dependence on fossil fuels.
4.1.Biomass Resource Assessment and Prediction
A central challenge in biomass energy production revolves around the dependable assessment and
prediction of biomass resources [33]. AI algorithms, including ChatGPT, can analyze extensive data from
diverse sources, encompassing satellite imagery, meteorological forecasts, and historical data, to estimate the
availability and growth potential of biomass. This information proves indispensable for biomass power
plants and biofuel manufacturers, facilitating efficient operational planning and ensuring a steady supply of
feedstock.
4.2.Optimization of Biomass Supply Chain
AI holds the capability to optimize the entire biomass supply chain, encompassing harvesting,
transportation, storage, and processing. ChatGPT can contribute to scheduling and route planning for
biomass collection and transportation, considering factors such as fuel efficiency, logistics costs, and
environmental impact. Through supply chain streamlining, AI aids in waste reduction, energy conservation,
and cost minimization.
4.3.Biomass Conversion Technologies
The conversion of biomass into energy typically involves diverse technologies like combustion,
gasification, and fermentation. AI can enhance the optimization of these processes by continuously
analyzing real-time data from sensors and making adjustments to enhance efficiency and decrease emissions
[34, 35]. For instance, AI can fine-tune combustion parameters in biomass power plants to optimize energy
output while minimizing environmental repercussions.
4.4.Energy Production Forecasting
AI models, akin to ChatGPT, can develop predictive models for biomass energy production. Through
scrutiny of historical data, weather predictions, and operational variables, AI can forecast energy production
levels, empowering grid operators and energy firms to adeptly integrate biomass energy into the electrical
grid. Precise forecasts underpin grid stability and diminish the need for backup power sources.
4.5.Energy Demand Management
AI also contributes to demand-side management in the realm of biomass energy. By analyzing consumer
behavior and energy consumption patterns, AI aids in harmonizing the supply and demand for biomass
energy. Smart grids infused with AI algorithms optimize energy distribution, ensuring judicious use of
biomass energy and minimizing wastage.
4.6.Emissions Monitoring and Reduction
Despite its renewable nature, biomass energy can still generate emissions if not managed meticulously. AI
Journal of Advances in Artificial Intelligence 9 Volume 2, Number 1, 2024](https://d2z384uprhdr6y.cloudfront.net/2mDSx23aptCUVycfIH66qX2zJnMeoe_ghvYj4iua3-4/rt:fill/q:100/w:1280/h:0/gravity:sm/czM6Ly9qYXVudC1wcm9kdWN0aW9uLXVwbG9hZHMvMjAyNC8xMi8yNS8xMDE0ZTQ0OC03ZGViLTQyODMtYjRkMi03MDRlMGQ5OTI0Mzcvc2xpZGVfOS1sLndlYnA=.webp)
![stands poised to monitor emissions in real-time, employing sensors and data analysis to detect and mitigate
pollutant levels. ChatGPT plays a role in data interpretation and decision-making, ensuring adherence to
environmental regulations and reduction of the carbon footprint associated with biomass energy operations.
4.7.Research and Development
AI can expedite research and development endeavors in the sphere of biomass energy. ChatGPT can
scrutinize scientific literature, propose experiments, and even generate hypotheses for researchers. This
facilitates the discovery of more efficient biomass conversion technologies, improved feedstock selection,
and enhanced biomass-to-energy processes.
4.8.Predictive Maintenance
AI-driven predictive maintenance systems enhance the reliability of biomass energy facilities. By
analyzing data from sensors and equipment, AI can predict when maintenance is required, thus reducing
downtime and averting costly breakdowns. This proactive approach to maintenance augments overall
efficiency and longevity of biomass energy infrastructure.
4.9.Education and Outreach
ChatGPT proves valuable in educating the public about the merits of biomass energy as a renewable and
sustainable energy source. It can generate informative content, respond to inquiries, and engage individuals
keen on learning more about biomass energy, thereby promoting its adoption and acceptance.
4.10. Policy and Decision Support
AI provides invaluable insights and assistance to policymakers in shaping strategies and regulations
concerning biomass energy. ChatGPT can analyze data, produce reports, and offer recommendations to
facilitate informed decision-making regarding subsidies, incentives, and environmental standards for
biomass energy initiatives.
Generative artificial intelligence, such as ChatGPT, and analogous technologies stand poised to
revolutionize the biomass energy sector. By optimizing resource assessment, supply chain management,
energy production, emissions reduction, and research and development, AI can significantly augment the
efficiency, sustainability, and overall viability of biomass energy as a renewable energy source. In a world
where combating climate change and reducing fossil fuel reliance is imperative, the integration of AI into the
biomass energy industry marks a promising stride towards a more sustainable and environmentally friendly
energy future.
5. Contribution of ChatGPT in Hydropower
Renewable and sustainable energy sources are paramount in combatting the global energy crisis and
alleviating the consequences of climate change. Among these sources, hydropower, a well-established and
widely adopted technology, stands out as a significant contributor to renewable energy production. It
harnesses the kinetic energy of flowing water to generate electricity, providing a clean and reliable power
source. However, optimizing, managing, and ensuring the environmental sustainability of hydropower
systems present complex challenges [36, 37]. In this context, the utilization of ChatGPT and similar
generative artificial intelligence (AI) models holds immense potential to transform the hydropower industry.
5.1.Utilizing Data for Informed Decision-Making
One of the primary ways in which ChatGPT and generative AI can benefit the hydropower sector is
through data-driven decision-making [38]. Hydropower plants generate vast quantities of data, encompassing
Journal of Advances in Artificial Intelligence 10 Volume 2, Number 1, 2024](https://d2z384uprhdr6y.cloudfront.net/3_G5_6F0hqJ6DNBN8KN4I0qlTu6fyNkxS7I1Je8C2uw/rt:fill/q:100/w:1280/h:0/gravity:sm/czM6Ly9qYXVudC1wcm9kdWN0aW9uLXVwbG9hZHMvMjAyNC8xMi8yNS8xMDE0ZTQ0OC03ZGViLTQyODMtYjRkMi03MDRlMGQ5OTI0Mzcvc2xpZGVfMTAtbC53ZWJw.webp)
![water flow rates, weather conditions, turbine efficiency, and grid demand. Analyzing this data is pivotal for
fine-tuning energy production and guaranteeing system reliability. Generative AI models like ChatGPT can
sift through this data, pinpoint patterns, and offer real-time recommendations to plant operators and
engineers. For instance, ChatGPT can scrutinize historical data to predict water flow patterns, allowing
operators to anticipate fluctuations in power generation and optimize reservoir management. Furthermore,
it can offer insights into turbine performance, suggesting maintenance schedules to maximize efficiency and
minimize downtime. Leveraging AI-driven analytics, the hydropower sector can amplify energy output and
trim operational costs, thereby fostering a more sustainable and dependable energy supply.
5.2.Assessing Environmental Impact
Hydropower projects often undergo scrutiny due to their environmental impact on river ecosystems,
aquatic life, and local communities [39]. Generative AI can play a pivotal role in evaluating and mitigating
these effects. ChatGPT can simulate the ecological consequences of various hydropower operation scenarios,
aiding policymakers and project developers in making informed decisions. By simulating scenarios and
analyzing potential outcomes, generative AI can help identify the most ecologically sustainable operating
strategies, such as seasonal flow variations or the creation of fish-friendly fish ladders and bypass channels.
Additionally, AI can assist in monitoring and mitigating the effects of climate change on river systems, which
can impact hydropower generation reliability. In this manner, ChatGPT and similar AI models contribute to
making hydropower a more environmentally responsible form of renewable energy.
5.3.Enhancing Predictive Maintenance
The reliability of hydropower plants is vital for their long-term sustainability. Unforeseen equipment
failures can disrupt energy production and result in costly downtime. Predictive maintenance, facilitated by
generative AI, can significantly reduce these risks. Generative AI models like ChatGPT can analyze sensor data
from turbines, generators, and other critical components of hydropower plants. By recognizing wear
patterns and potential failure indicators, AI can forecast maintenance needs well in advance. Plant operators
can then schedule maintenance during periods of low demand or favorable weather conditions, minimizing
disruptions to energy production. This proactive approach not only bolsters reliability but also prolongs the
lifespan of hydropower equipment, rendering the energy source more sustainable in the long run.
5.4.Addressing Energy Storage and Grid Integration Challenges
ChatGPT and generative AI can also aid in confronting one of the key challenges of hydropower generation:
energy storage and grid integration. Hydropower often experiences intermittency, reliant on water flow,
which can fluctuate due to seasonal and weather variations. Generative AI can optimize the incorporation of
hydropower into the broader energy grid by predicting energy generation and consumption patterns. For
instance, ChatGPT can analyze historical data to predict periods of high energy demand and low hydropower
generation. It can then recommend the utilization of energy storage solutions like pumped hydro storage or
advanced battery systems to store surplus energy during abundance and release it when demand peaks. This
enhances hydropower's reliability as a sustainable energy source, ensuring a consistent energy supply for
consumers.
5.5.Facilitating Infrastructure Planning and Design
Developing new hydropower projects necessitates meticulous planning and design to maximize energy
generation while minimizing environmental impacts. ChatGPT and generative AI can provide assistance in
this process by simulating various project scenarios and assessing their feasibility. These AI models can
scrutinize topographical data, water flow patterns, and environmental constraints to propose optimal dam
Journal of Advances in Artificial Intelligence 11 Volume 2, Number 1, 2024](https://d2z384uprhdr6y.cloudfront.net/1G-rsB9nyyW9jNGyTIlZhFsmUwRcnJ4Ch41X7ElJ3JA/rt:fill/q:100/w:1280/h:0/gravity:sm/czM6Ly9qYXVudC1wcm9kdWN0aW9uLXVwbG9hZHMvMjAyNC8xMi8yNS8xMDE0ZTQ0OC03ZGViLTQyODMtYjRkMi03MDRlMGQ5OTI0Mzcvc2xpZGVfMTEtbC53ZWJw.webp)
![locations and reservoir sizes [40]. They can also contribute to designing efficient and environmentally
friendly fish passage systems. By offering precise, data-driven insights, AI can assist project developers in
making informed decisions that strike a balance between energy generation and environmental
sustainability.
5.6.Improving Energy Forecasting and Market Optimization
To ensure the efficient utilization of hydropower resources, it is imperative to accurately forecast energy
generation and market conditions. Generative AI can be leveraged to construct sophisticated forecasting
models that account for factors such as weather, river flow, and grid demand. ChatGPT can scrutinize
historical data and meteorological information to provide short-term and long-term energy generation
forecasts. These forecasts can be employed by energy market participants to optimize their operations,
encompassing energy trading and dispatch. By precisely predicting energy supply and demand, AI-driven
forecasting can enhance the economic viability of hydropower projects, rendering them more competitive in
the energy market.
5.7.Fostering Human-Machine Collaboration
Although AI can autonomously perform various tasks, human expertise remains invaluable in the
hydropower industry. ChatGPT and similar AI models can facilitate collaboration between humans and
machines, thereby enhancing decision-making and problem-solving. For instance, plant operators and
engineers can interact with ChatGPT to access real-time data, receive recommendations, and simulate
different operational scenarios. This partnership between humans and machines enables quicker and more
informed decisions, ultimately elevating the overall efficiency and sustainability of hydropower plants.
Hydropower has long been acknowledged as a reliable and sustainable source of renewable energy.
Nevertheless, its full potential remains untapped, with challenges related to data analysis, environmental
impact assessment, equipment reliability, and grid integration persisting. Generative AI models like ChatGPT
offer innovative solutions to address these challenges and unlock the true potential of hydropower as a clean
and renewable energy source.
By enabling data-driven decision-making, predicting maintenance needs, assessing environmental impacts,
optimizing energy storage and grid integration, and aiding in infrastructure planning and design, ChatGPT
and generative AI can revolutionize the hydropower industry. These technologies facilitate a more sustainable
and efficient use of hydropower resources, contributing to the global transition to cleaner energy sources
and the mitigation of climate change. As the world continues to prioritize renewable energy, the role of AI in
advancing hydropower cannot be understated, paving the way for a more sustainable and resilient energy
future.
6. Role of ChatGPT in Geothermal Energy
Artificial Intelligence models like ChatGPT have the potential to play a significant role in advancing the field
of geothermal energy, elevating its status as a reliable and sustainable source of renewable power [41].
Geothermal energy taps into the Earth's inherent heat from its core, offering a consistent and
environmentally friendly energy supply. Despite its immense potential, the efficient and sustainable
utilization of geothermal energy faces numerous challenges. AI technologies, including ChatGPT, hold
promise in effectively addressing these challenges by aiding in resource assessment, exploration,
optimization, and the overall management of geothermal energy systems. One of the primary ways in which
ChatGPT and similar AI models can contribute to geothermal energy is by enhancing resource assessment
and exploration [42, 43]. The identification of suitable geothermal reservoirs is paramount for successful
geothermal project development. AI models excel at analyzing extensive geological data, encompassing
Journal of Advances in Artificial Intelligence 12 Volume 2, Number 1, 2024](https://d2z384uprhdr6y.cloudfront.net/Ot6exrOuBjWkG1Ka5ZsociP6HyfWrLZumlfIaBW9By4/rt:fill/q:100/w:1280/h:0/gravity:sm/czM6Ly9qYXVudC1wcm9kdWN0aW9uLXVwbG9hZHMvMjAyNC8xMi8yNS8xMDE0ZTQ0OC03ZGViLTQyODMtYjRkMi03MDRlMGQ5OTI0Mzcvc2xpZGVfMTItbC53ZWJw.webp)
![parameters such as subsurface temperature gradients, rock permeability, and seismic data, to pinpoint
potential geothermal reservoirs with high energy-producing potential. These AI models can process vast
datasets more swiftly and accurately than human experts, assisting geologists and geophysicists in
determining the optimal locations for drilling geothermal wells.
Furthermore, AI can significantly optimize drilling operations, which are intricate and costly processes
when reaching deep into the Earth's crust to access geothermal reservoirs. AI algorithms can fine-tune
drilling parameters, including drill bit selection, drilling speed, and well trajectory, resulting in increased
drilling efficiency and cost reduction. Additionally, AI can predict potential drilling challenges by analyzing
historical drilling data and geological information, enabling proactive measures to mitigate risks and
minimize downtime [40, 44]. ChatGPT and analogous AI models also facilitate reservoir management in
geothermal energy systems. Once a geothermal well becomes operational, maintaining efficient energy
production becomes imperative. AI continuously monitors well performance by scrutinizing real-time data
such as temperature, pressure, and flow rates. By identifying anomalies and patterns within the data, AI can
offer early warnings of potential issues, allowing operators to take prompt corrective actions and maximize
energy production.
Another crucial role for AI lies in optimizing the overall geothermal energy production process. AI-driven
predictive maintenance can reduce equipment downtime by anticipating when components such as pumps,
turbines, and heat exchangers are likely to fail. This enables scheduled maintenance rather than unexpected
breakdowns, ensuring uninterrupted energy generation. Furthermore, AI can enhance the efficiency of
geothermal power plants by optimizing power generation processes. Machine learning algorithms can
analyze historical operational data to uncover opportunities for enhancing energy conversion and heat
transfer processes. This optimization can lead to increased energy output and reduced operational costs,
making geothermal energy even more competitive among other renewable energy sources. Geothermal
reservoirs can be intricate, and their behavior may evolve over time. AI models assist in reservoir
monitoring and management by analyzing data from multiple sensors positioned within the reservoir,
encompassing measurements of temperature, pressure, and chemical composition. AI can detect subtle
changes in reservoir conditions, aiding operators in making informed decisions to maximize reservoir
longevity and sustain energy production. Additionally, ChatGPT and similar AI models can serve as valuable
tools in geothermal energy education and public outreach. AI-powered chatbots and virtual assistants can
disseminate information to the public about geothermal energy, its advantages, and its environmental impact.
These AI tools can answer inquiries and promote awareness of geothermal energy as a sustainable and
dependable energy source [40, 45, 46]. ChatGPT and akin Generative Artificial Intelligence models hold
immense potential for advancing geothermal energy as a renewable and sustainable energy source. They
contribute to resource assessment, exploration, drilling optimization, reservoir management, predictive
maintenance, and process optimization. As technology continues to advance, the synergy between AI and
geothermal energy will likely yield even more innovative solutions and deeper integration of geothermal
power into the global energy landscape.
7. Contribution of ChatGPT in Energy Storage
Artificial intelligence models hold the potential to make substantial contributions to the realm of energy
storage for sustainable and renewable energy sources [47–49]. Here's how ChatGPT and analogous AI models
can play a central role in advancing energy storage:
Optimizing energy storage systems: AI's primary contribution to energy storage lies in optimization [47,
50]. AI models, including ChatGPT, can analyze copious amounts of data from diverse sources, encompassing
weather patterns, energy demand fluctuations, and historical energy production data. By forecasting periods
Journal of Advances in Artificial Intelligence 13 Volume 2, Number 1, 2024](https://d2z384uprhdr6y.cloudfront.net/reeCiIHus7CAA5b1hz3Ci1d7-kZIDGIeLBne_xsFm7A/rt:fill/q:100/w:1280/h:0/gravity:sm/czM6Ly9qYXVudC1wcm9kdWN0aW9uLXVwbG9hZHMvMjAyNC8xMi8yNS8xMDE0ZTQ0OC03ZGViLTQyODMtYjRkMi03MDRlMGQ5OTI0Mzcvc2xpZGVfMTMtbC53ZWJw.webp)
![of peak energy production and demand, AI can facilitate the efficient management of energy storage. This
ensures that surplus energy generated during high-production periods is stored effectively for use during
peak demand, thus optimizing the overall energy ecosystem.
Advancing battery technology: AI can greatly assist in the research and enhancement of battery technology,
a pivotal element of energy storage systems [51, 52]. AI models can simulate various chemical compositions
and structural configurations, identifying materials that offer superior energy density, longer life cycles, and
faster charging capabilities. This expedited material discovery process can result in the creation of more
efficient and cost-effective energy storage solutions, ultimately diminishing dependence on fossil fuels.
Predictive maintenance: AI can extend the longevity and reliability of energy storage systems by predicting
maintenance requirements. Continuous monitoring and data analysis by AI models can detect potential
issues, enabling scheduled maintenance before they escalate into critical problems. This proactive approach
minimizes downtime and ensures energy storage systems operate at peak performance, boosting overall
efficiency.
Grid management: Renewable energy sources often generate power intermittently, posing a challenge for
grid management. AI can assist in balancing supply and demand by forecasting energy generation and
consumption patterns. ChatGPT can engage in natural language conversations with grid operators,
delivering real-time insights and recommendations for effective grid management. This dynamic decision
support can optimize the use of energy storage systems, stabilizing the grid and diminishing reliance on
fossil fuels.
Energy forecasting: Precise energy production and consumption forecasting are imperative for the
planning and management of renewable energy systems. AI models like ChatGPT can analyze historical data,
weather patterns, and other pertinent factors to furnish accurate energy production forecasts. This
information empowers grid operators and energy providers to make informed decisions regarding the
utilization of energy storage systems, reducing waste and maximizing renewable resource utilization.
Demand response: AI-powered chatbots, such as ChatGPT, can interact with consumers to promote
sustainable energy practices. By providing real-time information on energy demand, pricing, and availability,
AI can incentivize consumers to shift their energy consumption to periods when renewable energy is
abundant and cost-effective. This demand response mechanism can diminish the necessity for additional
energy storage capacity during peak demand periods.
Policy and regulation support: AI can also lend a hand in the formulation of policies and regulations that
foster the adoption of renewable energy and energy storage technologies. ChatGPT can scrutinize extensive
volumes of legislative documents, scientific literature, and industry reports to offer policymakers valuable
insights and recommendations for crafting effective policies that encourage the adoption of sustainable
energy solutions.
8. Contribution of ChatGPT in Grid Integration and Smart Grids
Generative artificial intelligence (AI) models hold significant promise in advancing grid integration and
smart grids for sustainable and renewable energy. They offer a range of applications that can boost the
efficiency, dependability, and eco-friendliness of energy systems [53, 54]. One pivotal area where ChatGPT
and similar AI models can make a substantial impact is in demand forecasting and optimization. Smart grids
rely heavily on precise predictions of energy demand to optimize resource allocation, enhance grid stability,
and reduce energy wastage. ChatGPT can effectively analyze historical data, weather patterns, and other
pertinent information to generate accurate forecasts of energy demand. These forecasts, in turn, assist grid
operators in making well-informed decisions regarding the allocation of renewable energy resources, such as
wind and solar power, to meet expected demand levels. By decreasing reliance on fossil fuels and optimizing
energy distribution, AI-powered demand forecasting contributes significantly to the creation of a more
Journal of Advances in Artificial Intelligence 14 Volume 2, Number 1, 2024](https://d2z384uprhdr6y.cloudfront.net/H-1nQzauujXIPFU4a518SUi54ca7K-PHx5Woa3n34Oo/rt:fill/q:100/w:1280/h:0/gravity:sm/czM6Ly9qYXVudC1wcm9kdWN0aW9uLXVwbG9hZHMvMjAyNC8xMi8yNS8xMDE0ZTQ0OC03ZGViLTQyODMtYjRkMi03MDRlMGQ5OTI0Mzcvc2xpZGVfMTQtbC53ZWJw.webp)
![sustainable energy grid. Table 3 shows the role of ChatGPT in grid integration and smart grids.
Moreover, AI plays a crucial role in real-time grid management by continuously analyzing grid data [55, 56].
Smart grids generate vast amounts of data from diverse sensors and devices, and the ability to process this
data in real time is vital for maintaining grid stability. AI models can swiftly analyze this data, detecting
anomalies or grid issues and suggesting corrective measures. For instance, if a sudden drop in solar power
production occurs due to cloud cover, ChatGPT can recommend boosting output from other renewable
sources or scaling up energy storage systems to compensate. Such real-time decision-making enhances the
reliability and resilience of the grid, particularly in coping with the intermittent nature of renewable energy
sources. Furthermore, ChatGPT can elevate energy management at the consumer level. Utilizing chatbots or
virtual assistants, AI can interact with consumers and offer tailored suggestions for energy conservation and
optimization. It can recommend adjustments to thermostat settings, optimize the charging of electric vehicles
to coincide with peak renewable energy production, or even schedule energy-intensive tasks like laundry
during off-peak hours. These personalized recommendations empower consumers to reduce their energy
bills and contribute to load balancing on the grid.
Table 3. Role of ChatGPT in Grid Integration and Smart Grids
Sr.
No.
Contribution Description Benefits Limitations
1 Information
Dissemination
Real-time updates on grid tech &
regs.
Enhanced awareness Reliability of information
2 Education and Training Tool for smart grid education Knowledge transfer Limited interaction
3 Problem Solving Troubleshooting grid issues Rapid issue resolution Complex, unique
problems
4 Data Analysis Identifying patterns & anomalies Data-driven insights Data quality, privacy
concerns
5 Policy Insights Policy recommendations Informed
decision-making
Interpretation of
regulations
6 Simulation and
Modeling
Grid scenario simulation &
modeling
Improved planning Model accuracy,
complexity
7 Energy Management Energy efficiency
recommendations
Cost reduction Real-time adaptation
required
8 Customer Engagement Customer inquiries & info
provision
Enhanced satisfaction Limited emotional
intelligence
9 Regulatory Compliance Compliance support for regs &
standards
Reduced non-compliance
risk
Interpretation of
regulations
10 Forecasting Predictions on demand & grid
stability
Improved resource
allocation
Prediction accuracy
AI models also play a pivotal role in predicting equipment failures and optimizing maintenance within the
renewable energy sector. Wind turbines, solar panels, and other renewable energy infrastructure require
regular maintenance for peak performance. By analyzing sensor data and historical maintenance records,
ChatGPT can predict when maintenance is required and recommend cost-effective scheduling for repairs.
This proactive approach reduces downtime, extends the lifespan of renewable energy assets, and ultimately
bolsters the sustainability of the energy infrastructure. Additionally, AI-driven predictive maintenance
extends to the grid infrastructure itself. Smart grids encompass various components, including transformers,
substations, and power lines. ChatGPT can analyze data from these components to anticipate potential
failures or inefficiencies, enabling grid operators to take preventive measures and prevent costly
disruptions.
In grid integration, AI models streamline the seamless incorporation of distributed energy resources
(DERs) into the grid. DERs, such as rooftop solar panels and home energy storage systems, can locally
Journal of Advances in Artificial Intelligence 15 Volume 2, Number 1, 2024](https://d2z384uprhdr6y.cloudfront.net/GZWhEIJrearaFH02KP1zm98NhXAfTvxn8LxCNezxptY/rt:fill/q:100/w:1280/h:0/gravity:sm/czM6Ly9qYXVudC1wcm9kdWN0aW9uLXVwbG9hZHMvMjAyNC8xMi8yNS8xMDE0ZTQ0OC03ZGViLTQyODMtYjRkMi03MDRlMGQ5OTI0Mzcvc2xpZGVfMTUtbC53ZWJw.webp)
![generate and store electricity. AI optimizes the coordination of these resources, ensuring they inject surplus
energy back into the grid when needed and charge when renewable energy generation is high. This flexibility
in managing DERs enhances grid reliability and diminishes the need for centralized fossil fuel power plants.
Furthermore, ChatGPT can supports grid operators in integrating electric vehicles (EVs) into the energy
ecosystem. With the increasing adoption of EVs, managing their charging and discharging patterns is critical
for grid stability [57]. AI provides EV owners with recommendations on optimal charging times to align with
renewable energy availability, alleviating the strain on the grid during peak demand. ChatGPT and analogous
generative AI models have the potential to transform grid integration and smart grids for renewable and
sustainable energy. By enhancing demand forecasting, real-time grid management, consumer engagement,
predictive maintenance, and the integration of DERs and EVs, these technologies substantially improve the
efficiency, reliability, and sustainability of energy systems. As renewable energy sources continue to play a
central role in the transition to a greener future, AI-driven solutions are indispensable in ensuring the
seamless integration of these resources into the grid.
9. Role of ChatGPT in Energy Efficiency
Generative Artificial Intelligence (AI), exemplified by models like ChatGPT, holds substantial promise for
enhancing energy efficiency within the realm of renewable and sustainable energy [58, 59]. These AI
systems can assume a pivotal role in streamlining various facets of energy production, consumption, and
management, thus propelling the evolution towards a more sustainable and environmentally conscious
energy ecosystem. A primary avenue through which ChatGPT and akin AI models can bolster energy
efficiency revolves around data analysis and forecasting. These AI systems excel at processing copious
real-time data, a crucial capability for renewable energy sources like solar and wind. They can scrutinize
meteorological patterns, historical energy production data, and consumption trends to furnish precise
predictions. For instance, ChatGPT can predict optimal periods for renewable energy generation and
anticipate peak demand times. Such predictive prowess enables superior planning and utilization of
renewable energy resources, mitigating energy wastage and optimizing production.
Moreover, ChatGPT can aid in optimizing energy distribution and grid management [60, 61]. It can
scrutinize regional demand patterns and proffer suggestions for tailoring the distribution of renewable
energy resources accordingly. Through intelligent routing of energy to high-priority areas, AI can curtail
transmission losses and ensure judicious use of renewable energy. Additionally, AI can oversee the well-being
and performance of grid infrastructure, promptly identifying issues such as equipment malfunctions or
voltage fluctuations. This proactive maintenance can forestall energy losses and power outages, elevating
overall energy efficiency. Furthermore, ChatGPT can elevate the efficiency of renewable energy systems
themselves. In the case of solar panels, AI can continually fine-tune panel angles and orientations to harness
the maximum sunlight. Similarly, for wind turbines, AI can adjust rotor speed and direction based on wind
conditions to optimize energy generation while minimizing wear and tear. These optimizations have the
potential to significantly augment the energy output of renewable sources, rendering them more competitive
with fossil fuels.
AI can also play an indispensable role in energy conservation and demand-side management [62]. It can
engage with users to furnish real-time energy-saving recommendations, such as optimizing thermostat
settings, managing lighting, or scheduling appliance usage during off-peak hours. By imparting personalized
energy-saving advice, AI can help curtail energy consumption at the individual and household levels, thus
contributing to overall energy efficiency. Additionally, AI-driven chatbots like ChatGPT can enhance energy
efficiency in industrial and commercial settings. They can aid in monitoring and regulating intricate systems,
such as manufacturing processes or building HVAC systems, to minimize energy wastage. Furthermore,
Journal of Advances in Artificial Intelligence 16 Volume 2, Number 1, 2024](https://d2z384uprhdr6y.cloudfront.net/7LdmMP0dX0nV_aMlka6COC8ysYsv0a0ZRw4KDzzAvxM/rt:fill/q:100/w:1280/h:0/gravity:sm/czM6Ly9qYXVudC1wcm9kdWN0aW9uLXVwbG9hZHMvMjAyNC8xMi8yNS8xMDE0ZTQ0OC03ZGViLTQyODMtYjRkMi03MDRlMGQ5OTI0Mzcvc2xpZGVfMTYtbC53ZWJw.webp)
![these chatbots can assist companies in identifying opportunities for energy efficiency enhancements and
offer counsel on sustainable practices, ultimately slashing energy costs and environmental impact.
In the arena of energy research and development, ChatGPT can expedite innovation. It can analyze
extensive datasets concerning renewable energy technologies, materials, and scientific investigations,
facilitating researchers in discerning promising avenues for advancing energy efficiency and sustainability.
By supporting scientists and engineers in generating novel ideas and hypotheses, AI can expedite the
formulation of groundbreaking technologies in the realm of renewable energy. Nonetheless, it is imperative
to acknowledge the challenges and ethical considerations entailed in deploying AI within the energy sector.
Concerns like data privacy, cybersecurity, and algorithmic bias must be addressed to ensure the responsible
integration of AI into energy efficiency initiatives. Furthermore, the transition towards a more sustainable
energy paradigm necessitates collaboration among governments, industries, and AI developers to ensure that
AI technologies align with broader environmental and societal objectives.
Generative AI such as ChatGPT holds the potential to revolutionize energy efficiency within renewable and
sustainable energy. Through data analysis, predictive capabilities, grid optimization, and energy conservation,
AI can make substantial contributions towards diminishing energy wastage and optimizing the advantages of
renewable energy resources. As AI technology continues to progress and intertwine with the energy sector,
it has the potential to expedite the transition towards a more sustainable and ecologically friendly energy
landscape, thus aiding in the battle against climate change and securing a cleaner energy future.
10. Contribution of ChatGPT in Materials Science and Nanotechnology
Generative artificial intelligence, exemplified by models like ChatGPT offers significant promise in
advancing the realms of Materials Science and Nanotechnology for Renewable and Sustainable Energy. These
cutting-edge technologies hold the potential to make substantial contributions by expediting research,
innovating materials, optimizing energy systems, and promoting communication and collaboration among
scientists and researchers in these domains [63, 64]. One of the primary avenues through which AI models
like ChatGPT can make impactful contributions to Materials Science and Nanotechnology is through their
ability to swiftly analyze and predict material properties. Within these fields, researchers often face the
formidable task of exploring an extensive array of materials to identify those suited for applications in
renewable and sustainable energy. AI can play a pivotal role by simulating the behaviors of diverse materials
under varying conditions, thereby saving valuable time and resources. With its vast knowledge base, ChatGPT
can assist researchers in formulating hypotheses and suggesting potential materials for specific purposes,
thus expediting the initial phases of material discovery.
Moreover, AI can optimize the design of materials at the nanoscale, a pivotal aspect of enhancing the
efficiency and sustainability of energy-related devices like solar cells and batteries [65, 66]. ChatGPT can aid
in the creation of nanostructures with precise properties, assisting scientists in fabricating materials that
enhance energy conversion and storage. By considering crucial factors such as size, shape, composition, and
surface properties, AI models can contribute to the development of materials that maximize energy output
while minimizing their environmental impact. Beyond materials design, AI can also play a pivotal role in the
sustainable energy sector by streamlining manufacturing processes. ChatGPT can offer recommendations for
more efficient and environmentally friendly manufacturing methods, ultimately leading to reductions in
waste and energy consumption during production. This, in turn, can result in cost savings and a diminished
carbon footprint, aligning seamlessly with the objectives of sustainable energy production. Furthermore,
collaboration and the dissemination of knowledge are integral components of materials science and
nanotechnology. ChatGPT and analogous AI models can function as potent communication tools for scientists
on a global scale. They can facilitate discussions, provide answers to inquiries, and even assist in the
Journal of Advances in Artificial Intelligence 17 Volume 2, Number 1, 2024](https://d2z384uprhdr6y.cloudfront.net/bTk576PJaEqbv7FgYDeXh74OBHbBxa0DMJZDIoSA_mk/rt:fill/q:100/w:1280/h:0/gravity:sm/czM6Ly9qYXVudC1wcm9kdWN0aW9uLXVwbG9hZHMvMjAyNC8xMi8yNS8xMDE0ZTQ0OC03ZGViLTQyODMtYjRkMi03MDRlMGQ5OTI0Mzcvc2xpZGVfMTctbC53ZWJw.webp)
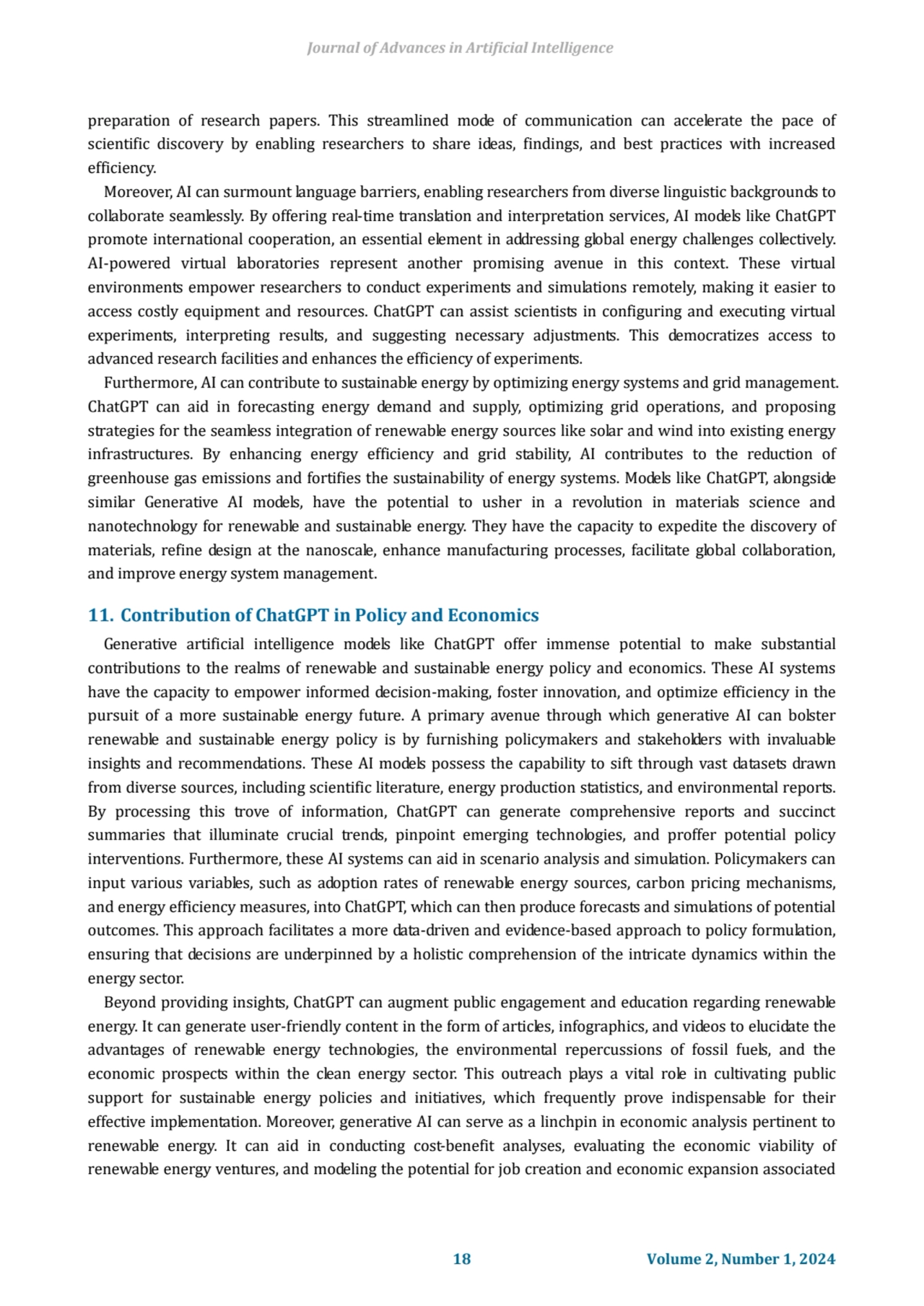
![with the clean energy sector. These insights are of paramount importance to both governmental and private
sector decision-makers, as they assist in the judicious allocation of resources and the identification of
investment opportunities aligned with sustainability objectives.
Another noteworthy contribution of ChatGPT and analogous AI models lies in the optimization of energy
systems [67, 68]. The integration of renewable energy sources into the grid presents challenges owing to
their intermittency and variability. AI can be instrumental in managing these challenges by optimizing
energy generation, storage, and distribution. For instance, AI can anticipate energy demand patterns and
adjust renewable energy production accordingly, thereby curtailing energy wastage and ensuring a stable
supply. This optimization not only fosters sustainability but also translates into long-term cost savings.
AI-driven analytics can further aid in the identification and prioritization of renewable energy projects. By
analyzing geographic, meteorological, and infrastructure data, these systems can proffer recommendations
on the most suitable locations for solar farms, wind turbines, and other renewable energy installations. This
streamlines the decision-making process for investors and mitigates the risks associated with project
development. Furthermore, generative AI can facilitate international collaboration on renewable energy
initiatives. Climate change and energy sustainability are global challenges that necessitate cooperation
among nations. ChatGPT can assist in the translation of documents and discussions related to renewable
energy policy and economics, simplifying the sharing of knowledge and best practices among experts and
policymakers from diverse countries. It can also facilitate cross-cultural communication and negotiation,
fostering international accords and initiatives aimed at sustainable energy development.
12. Contribution of ChatGPT in Tidal and Wave Energy
Artificial Intelligence models such as ChatGPT hold significant promise in advancing the field of tidal and
wave energy production, contributing to renewable and sustainable energy solutions [69, 70]. Tidal and wave
energy sources harness the natural motion of oceans and seas, and AI stands poised to play a pivotal role in
optimizing their efficiency and viability. A fundamental avenue through which ChatGPT and similar AI models
can make a meaningful impact in the realm of tidal and wave energy is through data analysis and predictive
capabilities. These AI models excel at processing and interpreting vast datasets, a critical requirement for
comprehending the intricate behavior of tides and waves across different geographical regions. By delving
into historical tidal and wave data, AI systems can identify patterns and trends, empowering energy
companies and researchers to enhance their capacity to forecast optimal energy generation conditions. This
predictive prowess is invaluable for strategizing energy generation and grid integration, ensuring a steady
and consistent supply of renewable energy.
Moreover, ChatGPT can lend its expertise to the design of more efficient and cost-effective wave and tidal
energy systems. AI-driven simulations can replicate the behavior of diverse device configurations,
fine-tuning factors like shape, size, and placement to maximize energy capture. This iterative design process
can substantially reduce the trial-and-error phase in technology development, expediting progress within the
field. Additionally, AI can scrutinize and enhance the materials employed in these systems, augmenting their
durability and resilience in challenging marine environments, ultimately curbing maintenance expenses and
prolonging the lifespan of these renewable energy installations. AI-driven predictive maintenance represents
another invaluable contribution to tidal and wave energy. The upkeep of offshore energy equipment can be
both expensive and logistically complex. AI can continuously monitor the condition of equipment, sensors,
and other components, foreseeing when maintenance or repairs are required. This proactive approach can
help avert costly downtimes and ensure the longevity of these installations, enhancing the reliability and
economic feasibility of tidal and wave energy solutions. ChatGPT and analogous AI models can also elevate
the control systems governing tidal and wave energy converters. These systems must adapt swiftly to
Journal of Advances in Artificial Intelligence 19 Volume 2, Number 1, 2024](https://d2z384uprhdr6y.cloudfront.net/9nhWgJvwemUkaPqPdkZ8dJ2BKymnCTZzBoqAr1kYNXw/rt:fill/q:100/w:1280/h:0/gravity:sm/czM6Ly9qYXVudC1wcm9kdWN0aW9uLXVwbG9hZHMvMjAyNC8xMi8yNS8xMDE0ZTQ0OC03ZGViLTQyODMtYjRkMi03MDRlMGQ5OTI0Mzcvc2xpZGVfMTktbC53ZWJw.webp)
![ever-changing oceanic conditions to optimize energy capture. AI can process real-time sensor data and make
instantaneous decisions to fine-tune device settings for peak efficiency. This dynamic control can yield higher
energy yields, especially in challenging marine environments characterized by unpredictable wave patterns.
Furthermore, AI can simplify the integration of tidal and wave energy into existing power grids [71].
These renewable energy sources often generate power intermittently, contingent upon oceanic conditions.
AI algorithms can manage this variable energy output, coordinating with other renewables such as wind and
solar, as well as storage systems like batteries. This ensures a dependable and steady energy supply,
diminishing dependence on fossil fuels and contributing to a more sustainable energy mix. ChatGPT and
analogous AI models can also assist in addressing environmental concerns linked to tidal and wave energy.
Through data analysis pertaining to the impact of these energy systems on marine ecosystems, AI can
facilitate the development of mitigation strategies aimed at minimizing harm to marine life. This proactive
approach ensures that renewable energy projects are not just sustainable but also environmentally
responsible.
13. Contribution of ChatGPT in Advanced Biofuels
Generative artificial intelligence (AI), as exemplified by models like ChatGPT, holds significant promise in
advancing the realm of biofuels for sustainable and renewable energy [72, 73]. Biofuels are pivotal in our
transition to a more environmentally friendly and sustainable energy landscape, offering a renewable
alternative to fossil fuels. AI technologies, such as ChatGPT, can play a pivotal role in various aspects of biofuel
development, encompassing optimization of production processes, resource utilization enhancement, and
progress in research within this domain. One of the primary domains where ChatGPT and analogous
generative AI systems can make substantial contributions is in the optimization of biofuel production
processes. The production of biofuels involves intricate biochemical reactions, and enhancing these
processes is critical for improving yields and reducing costs. Generative AI can assist in this optimization by
scrutinizing extensive datasets and pinpointing ideal conditions for fermentation, enzymatic reactions, and
other steps involved in biofuel production. By harnessing machine learning algorithms, AI models can
empower researchers and engineers to discover more efficient and cost-effective techniques for converting
biomass into biofuels.
Moreover, ChatGPT can facilitate real-time monitoring and control of biofuel production processes. It can process and
analyze data from sensors deployed in bioreactors, fermentation tanks, and other equipment to ensure seamless
production. This AI-driven monitoring can swiftly detect anomalies and deviations from optimal conditions, enabling
timely interventions to prevent costly disruptions and enhance overall production efficiency. Consequently, the
reliability and consistency of biofuel production can be elevated, bolstering its scalability and commercial viability.
Generative AI can also play a pivotal role in designing novel biofuels and optimizing existing biofuel formulations.
Researchers can employ AI-powered simulations and molecular modeling to predict biofuel properties and performance,
including aspects like energy density, combustion efficiency, and environmental impact. Through the generation and
analysis of extensive datasets containing chemical structures and properties, AI models can propose promising
candidates for biofuel development, potentially leading to the discovery of more efficient and sustainable biofuels.
Another significant avenue where generative AI can contribute to advanced biofuels is in the optimization
of feedstock selection and resource utilization [74, 75]. The choice of biomass feedstock holds utmost
importance in biofuel production, impacting both environmental sustainability and economic feasibility. AI
models can analyze geographical and climatic data, along with factors like biomass availability and growth
rates, to identify the most suitable feedstock options for specific regions. This ensures that biofuel production
aligns with local conditions, optimizing resource utilization and minimizing environmental impact.
Furthermore, generative AI can aid in the development of sustainable agricultural practices for biomass
cultivation [76–78]. It can analyze parameters such as soil quality, climate patterns, and crop rotation
strategies to optimize the growth of biofuel feedstock crops, reducing reliance on synthetic fertilizers and
Journal of Advances in Artificial Intelligence 20 Volume 2, Number 1, 2024](https://d2z384uprhdr6y.cloudfront.net/zIC4Kn2Hgb3CBuFpWnV1SQKskWNOUJMd50rtJH2_j4E/rt:fill/q:100/w:1280/h:0/gravity:sm/czM6Ly9qYXVudC1wcm9kdWN0aW9uLXVwbG9hZHMvMjAyNC8xMi8yNS8xMDE0ZTQ0OC03ZGViLTQyODMtYjRkMi03MDRlMGQ5OTI0Mzcvc2xpZGVfMjAtbC53ZWJw.webp)
![pesticides. By promoting sustainable agriculture, AI contributes to the overall sustainability of the biofuel
supply chain. Beyond these practical applications, ChatGPT and similar AI models can foster collaboration
and knowledge-sharing among researchers and experts in advanced biofuels. They can assist in data analysis,
literature review, and the formulation of research hypotheses, thereby expediting innovation in biofuel
development. AI-powered chatbots and virtual assistants can also provide answers and information regarding
the latest advancements, facilitating the task of staying updated for scientists and engineers in the rapidly
evolving field of biofuels.
14. Conclusions
This research highlights the significant role that ChatGPT and generative artificial intelligence (AI) play in
advancing a wide range of renewable and sustainable energy technologies. AI, including ChatGPT, makes
valuable contributions and enhances performance in various areas such as solar energy, wind energy,
biomass energy, hydropower, geothermal energy, energy storage, grid integration, energy efficiency,
materials science, policy and economics, environmental impact and sustainability, energy access and equity,
tidal and wave energy, and advanced biofuels. These technologies hold the potential to drive innovation,
boost efficiency, and optimize sustainability in their respective domains. For example, AI can improve the
efficiency of solar panels, enable predictive maintenance for wind turbines, and assist in selecting the right
biomass feedstock. It can optimize hydropower operations, simulate the behavior of geothermal reservoirs,
and ensure the reliability of energy storage systems. AI also plays a vital role in managing smart grids,
providing recommendations for energy-efficient designs, and accelerating the discovery of materials for
renewable technologies. Additionally, it can model various policy scenarios, address environmental concerns,
and promote equitable access to energy resources. ChatGPT and generative AI have the potential to expedite
the global transition toward renewable and sustainable energy sources, mitigating environmental impacts
and enhancing energy accessibility while fostering economic growth. Nonetheless, it remains essential to
carefully consider the ethical, environmental, and societal implications associated with deploying AI in the
energy sector. Responsible integration of AI technologies will be critical to maximize benefits and minimize
potential risks as we strive for a cleaner and more sustainable energy future.
Conflict of Interest
The authors declare no conflict of interest.
References
[1] Vaishya, R., Javaid, M., Khan, I. H., & Haleem, A. (2020). Artificial Intelligence (AI) applications for
COVID-19 pandemic. Diabetes & Metabolic Syndrome: Clinical Research & Reviews, 14(4), 337–339.
[2] Haleem, A., Javaid, M., & Khan, I. H. (2019). Current status and applications of Artificial Intelligence (AI)
in medical field: An overview. Current Medicine Research and Practice, 9(6), 231–237.
[3] Winston, P. H. (1984). Artificial Intelligence. Addison-Wesley Longman Publishing Co., Inc.
[4] Haleem, A., Vaishya, R., Javaid, M., & Khan, I. H. (2020). Artificial Intelligence (AI) applications in
orthopaedics: an innovative technology to embrace. Journal of Clinical Orthopaedics and Trauma,
11(Suppl 1), S80.
[5] Owusu, P. A., & Asumadu-Sarkodie, S. (2016). A review of renewable energy sources, sustainability
issues and climate change mitigation. Cogent Engineering, 3(1), 1167990.
[6] Østergaard, P. A., Duic, N., Noorollahi, Y., Mikulcic, H., & Kalogirou, S. (2020). Sustainable development
using renewable energy technology. Renewable Energy, 146, 2430–2437.
[7] Fontes, C. H. D. O., & Freires, F. G. M. (2018). Sustainable and renewable energy supply chain: A system
Journal of Advances in Artificial Intelligence 21 Volume 2, Number 1, 2024](https://d2z384uprhdr6y.cloudfront.net/qO4myAOoe-CI7AHI36diQ0VMszTGjSSasd7kdCD5Wi0/rt:fill/q:100/w:1280/h:0/gravity:sm/czM6Ly9qYXVudC1wcm9kdWN0aW9uLXVwbG9hZHMvMjAyNC8xMi8yNS8xMDE0ZTQ0OC03ZGViLTQyODMtYjRkMi03MDRlMGQ5OTI0Mzcvc2xpZGVfMjEtbC53ZWJw.webp)
![dynamics overview. Renewable and Sustainable Energy Reviews, 82, 247–259.
[8] Montzka, S. A., Dlugokencky, E. J., & Butler, J. H. (2011). Non-CO2 greenhouse gases and climate change.
Nature, 476(7358), 43–50.
[9] Hussain, A., Arif, S. M., & Aslam, M. (2017). Emerging renewable and sustainable energy technologies:
State of the art. Renewable and Sustainable Energy Reviews, 71, 12–28.
[10] Kannan, N., & Vakeesan, D. (2016). Solar energy for future world: A review. Renewable and Sustainable
Energy Reviews, 62, 1092–1105.
[11] Liu, C., Li, F., Ma, L. P., & Cheng, H. M. (2010). Advanced materials for energy storage. Advanced Materials,
22(8), E28–E62.
[12] Ahmad, T., Zhang, D., Huang, C., Zhang, H., Dai, N., Song, Y., & Chen, H. (2021). Artificial intelligence in
sustainable energy industry: Status Quo, challenges and opportunities. Journal of Cleaner Production, 289,
125834.
[13] Patwa, N., Sivarajah, U., Seetharaman, A., Sarkar, S., Maiti, K., & Hingorani, K. (2021). Towards a circular
economy: An emerging economies context. Journal of Business Research, 122, 725–735.
[14] Milidonis, K., Blanco, M. J., Grigoriev, V., Panagiotou, C. F., Bonanos, A. M., Constantinou, M., ... &
Asselineau, C. A. (2021). Review of application of AI techniques to solar tower systems. Solar Energy,
224, 500–515.
[15] Meinel, A. B., & Meinel, M. P. (1977). Applied solar energy: An introduction. NASA STI/Recon Technical
Report A, 77, 33445.
[16] Gong, J., Li, C., & Wasielewski, M. R. (2019). Advances in solar energy conversion. Chemical Society
Reviews, 48(7), 1862–1864.
[17] Jiang, J. P., & Li, D. X. (2010). Optimal placement and decentralized robust vibration control for
spacecraft smart solar panel structures. Smart Materials and Structures, 19(8), 085020.
[18] Shin, W., Han, J., & Rhee, W. (2021). AI-assistance for predictive maintenance of renewable energy
systems. Energy, 221, 119775.
[19] Yang, D., Li, W., Yagli, G. M., & Srinivasan, D. (2021). Operational solar forecasting for grid integration:
Standards, challenges, and outlook. Solar Energy, 224, 930–937.
[20] Yang, D., Wang, W., Gueymard, C. A., Hong, T., Kleissl, J., Huang, J., ... & Peters, I. M. (2022). A review of
solar forecasting, its dependence on atmospheric sciences and implications for grid integration: Towards
carbon neutrality. Renewable and Sustainable Energy Reviews, 161, 112348.
[21] Chui, K. T., Lytras, M. D., & Visvizi, A. (2018). Energy sustainability in smart cities: Artificial intelligence,
smart monitoring, and optimization of energy consumption. Energies, 11(11), 2869.
[22] Wei, N., Li, C., Peng, X., Zeng, F., & Lu, X. (2019). Conventional models and artificial intelligence-based
models for energy consumption forecasting: A review. Journal of Petroleum Science and Engineering, 181,
106187.
[23] Mohammad, A., & Mahjabeen, F. (2023). Revolutionizing solar energy with AI-driven enhancements in
photovoltaic technology. BULLET: Jurnal Multidisiplin Ilmu, 2(4), 1174–1187.
[24] Abdalla, A. N., Nazir, M. S., Tao, H., Cao, S., Ji, R., Jiang, M., & Yao, L. (2021). Integration of energy storage
system and renewable energy sources based on artificial intelligence: An overview. Journal of Energy
Storage, 40, 102811.
[25] Haggi, H., Song, M., & Sun, W. (2019). A review of smart grid restoration to enhance cyber-physical
system resilience. 2019 IEEE Innovative Smart Grid Technologies-Asia (ISGT Asia), 4008–4013.
[26] Sulaiman, A., Nagu, B., Kaur, G., Karuppaiah, P., Alshahrani, H., Reshan, M. S. A., ... & Shaikh, A. (2023).
Artificial Intelligence-Based Secured Power Grid Protocol for Smart City. Sensors, 23(19), 8016.
[27] Zhao, E., Sun, S., & Wang, S. (2022). New developments in wind energy forecasting with artificial
Journal of Advances in Artificial Intelligence 22 Volume 2, Number 1, 2024](https://d2z384uprhdr6y.cloudfront.net/Wmc8aAMtlgyxoVxRNeIoNxMlheSj69Q3QXV2yA5v9sU/rt:fill/q:100/w:1280/h:0/gravity:sm/czM6Ly9qYXVudC1wcm9kdWN0aW9uLXVwbG9hZHMvMjAyNC8xMi8yNS8xMDE0ZTQ0OC03ZGViLTQyODMtYjRkMi03MDRlMGQ5OTI0Mzcvc2xpZGVfMjItbC53ZWJw.webp)
![intelligence and big data: A scientometric insight. Data Science and Management, 5(2), 84–95.
[28] Garcia Marquez, F. P., & Peinado Gonzalo, A. (2021). A comprehensive review of artificial intelligence and
wind energy. Archives of Computational Methods in Engineering, 1–24.
[29] Higgins, S., & Stathopoulos, T. (2021). Application of artificial intelligence to urban wind energy. Building
and Environment, 197, 107848.
[30] Sholapurkar, R. B., & Mahajan, Y. S. (2015). Review of wind energy development and policy in India.
Energy Technology & Policy, 2(1), 122–132.
[31] Dragomir, G., Șerban, A., Năstase, G., & Brezeanu, A. I. (2016). Wind energy in Romania: A review from
2009 to 2016. Renewable and Sustainable Energy Reviews, 64, 129–143.
[32] Abrahamson, W. G., & Caswell, H. (1982). On the comparative allocations of biomass, energy, and
nutrients in plants. Ecology, 63(4), 982–991.
[33] Meena, M., Shubham, S., Paritosh, K., Pareek, N., & Vivekanand, V. (2021). Production of biofuels from
biomass: Predicting the energy employing artificial intelligence modelling. Bioresource Technology, 340,
125642.
[34] Liao, M., & Yao, Y. (2021). Applications of artificial intelligence‐based modeling for bioenergy systems: A
review. GCB Bioenergy, 13(5), 774–802.
[35] Sakheta, A., Nayak, R., O'Hara, I., & Ramirez, J. (2023). A review on modelling of thermochemical
processing of biomass for biofuels and prospects of artificial intelligence-enhanced approaches.
Bioresource Technology, 129490.
[36] Kumar, K., & Saini, R. P. (2021). Application of artificial intelligence for the optimization of hydropower
energy generation. EAI Endorsed Transactions on Industrial Networks and Intelligent Systems, 8(28),
e1-e1.
[37] Rane, N. L., Achari, A., Choudhary, S. P., Mallick, S. K., Pande, C. B., Srivastava, A., & Moharir, K. (2023). A
decision framework for potential dam site selection using GIS, MIF and TOPSIS in Ulhas river basin,
India. Journal of Cleaner Production, 138890.
[38] Feng, Z. K., Niu, W. J., Zhang, T. H., Wang, W. C., & Yang, T. (2023). Deriving hydropower reservoir
operation policy using data-driven artificial intelligence model based on pattern recognition and
metaheuristic optimizer. Journal of Hydrology, 624, 129916.
[39] Quaranta, E., Bejarano, M. D., Comoglio, C., Fuentes-Pérez, J. F., Pérez-Díaz, J. I., Sanz-Ronda, F. J., ... &
Tuhtan, J. A. (2023). Digitalization and real-time control to mitigate environmental impacts along rivers:
Focus on artificial barriers, hydropower systems and European priorities. Science of The Total
Environment, 875, 162489.
[40] Moharir, K. N., Pande, C. B., Gautam, V. K., Singh, S. K., & Rane, N. L. (2023). Integration of hydrogeological
data, GIS and AHP techniques applied to delineate groundwater potential zones in sandstone, limestone
and shales rocks of the Damoh district,(MP) central India. Environmental Research, 228, 115832.
[41] Jha, S. K., Bilalovic, J., Jha, A., Patel, N., & Zhang, H. (2017). Renewable energy: Present research and
future scope of Artificial Intelligence. Renewable and Sustainable Energy Reviews, 77, 297–317.
[42] Khosravi, A., Syri, S., Zhao, X., & Assad, M. E. H. (2019). An artificial intelligence approach for
thermodynamic modeling of geothermal based-organic Rankine cycle equipped with solar system.
Geothermics, 80, 138–154.
[43] Hai, T., Ali, M. A., Chaturvedi, R., Almojil, S. F., Almohana, A. I., Alali, A. F., ... & Shamseldin, M. A. (2023). A
low-temperature driven organic Rankine cycle for waste heat recovery from a geothermal driven Kalina
cycle: 4E analysis and optimization based on artificial intelligence. Sustainable Energy Technologies and
Assessments, 55, 102895.
[44] Aminzadeh, F., Temizel, C., & Hajizadeh, Y. (2022). Artificial Intelligence and Data Analytics for Energy
Journal of Advances in Artificial Intelligence 23 Volume 2, Number 1, 2024](https://d2z384uprhdr6y.cloudfront.net/KXsiRtsHq5AY7ylX2SyKbw5zHY5NzCegS79rQQ-5BB0/rt:fill/q:100/w:1280/h:0/gravity:sm/czM6Ly9qYXVudC1wcm9kdWN0aW9uLXVwbG9hZHMvMjAyNC8xMi8yNS8xMDE0ZTQ0OC03ZGViLTQyODMtYjRkMi03MDRlMGQ5OTI0Mzcvc2xpZGVfMjMtbC53ZWJw.webp)
![Exploration and Production. John Wiley & Sons.
[45] Bist, N., Tripathi, G., Sircar, A., & Yadav, K. (2021). Artificial intelligence based optimizing solutions for
the geothermal power plants. Proceedings, 46th Workshop on Geothermal Reservoir Engineering,
Stanford University, Stanford, California.
[46] Khanmohammadi, S., Musharavati, F., & Tariq, R. (2022). A framework of data modeling and artificial
intelligence for environmental-friendly energy system: Application of Kalina cycle improved with fuel
cell and thermoelectric module. Process Safety and Environmental Protection, 164, 499–516.
[47] Abdalla, A. N., Nazir, M. S., Tao, H., Cao, S., Ji, R., Jiang, M., & Yao, L. (2021). Integration of energy storage
system and renewable energy sources based on artificial intelligence: An overview. Journal of Energy
Storage, 40, 102811.
[48] He, Z., Guo, W., & Zhang, P. (2022). Performance prediction, optimal design and operational control of
thermal energy storage using artificial intelligence methods. Renewable and Sustainable Energy Reviews,
156, 111977.
[49] He, Z., Guo, W., & Zhang, P. (2022). Performance prediction, optimal design and operational control of
thermal energy storage using artificial intelligence methods. Renewable and Sustainable Energy Reviews,
156, 111977.
[50] Olabi, A. G., Abdelghafar, A. A., Maghrabie, H. M., Sayed, E. T., Rezk, H., Al Radi, M., ... & Abdelkareem, M. A.
(2023). Application of artificial intelligence for prediction, optimization, and control of thermal energy
storage systems. Thermal Science and Engineering Progress, 101730.
[51] Boretti, A. (2021). Integration of solar thermal and photovoltaic, wind, and battery energy storage
through AI in NEOM city. Energy and AI, 3, 100038.
[52] Krishna, G., Singh, R., Gehlot, A., Akram, S. V., Priyadarshi, N., & Twala, B. (2022). Digital technology
implementation in battery-management systems for sustainable energy storage: Review, challenges, and
recommendations. Electronics, 11(17), 2695.
[53] Ali, S. S., & Choi, B. J. (2020). State-of-the-art artificial intelligence techniques for distributed smart grids:
A review. Electronics, 9(6), 1030.
[54] Khosrojerdi, F., Akhigbe, O., Gagnon, S., Ramirez, A., & Richards, G. (2021). Integrating artificial
intelligence and analytics in smart grids: a systematic literature review. International Journal of Energy
Sector Management, 16(2), 318–338.
[55] Hua, W., Chen, Y., Qadrdan, M., Jiang, J., Sun, H., & Wu, J. (2022). Applications of blockchain and artificial
intelligence technologies for enabling prosumers in smart grids: A review. Renewable and Sustainable
Energy Reviews, 161, 112308.
[56] Bayrak, G., & Yılmaz, A. (2021). Detection and classification of power quality disturbances in smart grids
using artificial intelligence methods. In Artificial Intelligence (AI) (pp. 149-170). CRC Press.
[57] Rane, N. L., Achari, A., Saha, A., Poddar, I., Rane, J., Pande, C. B., & Roy, R. (2023). An integrated GIS, MIF,
and TOPSIS approach for appraising electric vehicle charging station suitability zones in Mumbai, India.
Sustainable Cities and Society, 104717.
[58] Zhu, S., Ota, K., & Dong, M. (2022). Energy-efficient artificial intelligence of things with intelligent edge.
IEEE Internet of Things Journal, 9(10), 7525–7532.
[59] Merabet, G. H., Essaaidi, M., Haddou, M. B., Qolomany, B., Qadir, J., Anan, M., ... & Benhaddou, D. (2021).
Intelligent building control systems for thermal comfort and energy-efficiency: A systematic review of
artificial intelligence-assisted techniques. Renewable and Sustainable Energy Reviews, 144, 110969.
[60] Di Santo, K. G., Di Santo, S. G., Monaro, R. M., & Saidel, M. A. (2018). Active demand side management for
households in smart grids using optimization and artificial intelligence. Measurement, 115, 152–161.
[61] Khan, A. A., Laghari, A. A., Rashid, M., Li, H., Javed, A. R., & Gadekallu, T. R. (2023). Artificial intelligence
Journal of Advances in Artificial Intelligence 24 Volume 2, Number 1, 2024](https://d2z384uprhdr6y.cloudfront.net/ubSTTwxvFtRLrBXr8wpMdG5Po39HdEuhYbGHTXA0xFc/rt:fill/q:100/w:1280/h:0/gravity:sm/czM6Ly9qYXVudC1wcm9kdWN0aW9uLXVwbG9hZHMvMjAyNC8xMi8yNS8xMDE0ZTQ0OC03ZGViLTQyODMtYjRkMi03MDRlMGQ5OTI0Mzcvc2xpZGVfMjQtbC53ZWJw.webp)
![and blockchain technology for secure smart grid and power distribution Automation: A State-of-the-Art
Review. Sustainable Energy Technologies and Assessments, 57, 103282.
[62] Rocha, H. R., Honorato, I. H., Fiorotti, R., Celeste, W. C., Silvestre, L. J., & Silva, J. A. (2021). An Artificial
Intelligence based scheduling algorithm for demand-side energy management in Smart Homes. Applied
Energy, 282, 116145.
[63] Sacha, G. M., & Varona, P. (2013). Artificial intelligence in nanotechnology. Nanotechnology, 24(45),
452002.
[64] Rosa, V., Ho, D., Sabino-Silva, R., Siqueira, W. L., & Silikas, N. (2021). Fighting viruses with materials
science: Prospects for antivirus surfaces, drug delivery systems and artificial intelligence. Dental
Materials, 37(3), 496–507.
[65] Ammar, R. B., Ammar, M. B., & Oualha, A. (2020). Photovoltaic power forecast using empirical models
and artificial intelligence approaches for water pumping systems. Renewable Energy, 153, 1016–1028.
[66] Zhang, S., Wang, J., Liu, H., Tong, J., & Sun, Z. (2021). Prediction of energy photovoltaic power generation
based on artificial intelligence algorithm. Neural Computing and Applications, 33, 821–835.
[67] Zahraee, S. M., Assadi, M. K., & Saidur, R. (2016). Application of artificial intelligence methods for hybrid
energy system optimization. Renewable and Sustainable Energy Reviews, 66, 617–630.
[68] Kanase-Patil, A. B., Kaldate, A. P., Lokhande, S. D., Panchal, H., Suresh, M., & Priya, V. (2020). A review of
artificial intelligence-based optimization techniques for the sizing of integrated renewable energy
systems in smart cities. Environmental Technology Reviews, 9(1), 111–136.
[69] Rodriguez-Delgado, C., & Bergillos, R. J. (2021). Wave energy assessment under climate change through
artificial intelligence. Science of the Total Environment, 760, 144039.
[70] López, I., & Iglesias, G. (2014). Efficiency of OWC wave energy converters: A virtual laboratory. Applied
Ocean Research, 44, 63–70.
[71] Li, L., Gao, Y., Ning, D. Z., & Yuan, Z. M. (2021). Development of a constraint non-causal wave energy
control algorithm based on artificial intelligence. Renewable and Sustainable Energy Reviews, 138,
110519.
[72] Sakheta, A., Nayak, R., O'Hara, I., & Ramirez, J. (2023). A review on modelling of thermochemical
processing of biomass for biofuels and prospects of artificial intelligence-enhanced approaches.
Bioresource Technology, 129490.
[73] Liu, J., Zhou, F., Abed, A. M., Le, B. N., Dai, L., Ali, H. E., ... & Zhang, G. (2022). Macroalgae as a potential
source of biomass for generation of biofuel: Artificial intelligence, challenges, and future insights
towards a sustainable environment. Fuel, 126826.
[74] Ahmad, J., Awais, M., Rashid, U., Ngamcharussrivichai, C., Naqvi, S. R., & Ali, I. (2023). A systematic and
critical review on effective utilization of artificial intelligence for bio-diesel production techniques. Fuel,
338, 127379.
[75] Venkataramana, S. H., Shivalingaiah, K., Davanageri, M. B., Selvan, C. P., Lakshmikanthan, A.,
Chandrashekarappa, M. P. G., ... & Linul, E. (2022). Niger seed oil-based biodiesel production using
transesterification process: experimental investigation and optimization for higher biodiesel yield using
box–behnken design and artificial intelligence tools. Applied Sciences, 12(12), 5987.
[76] Gautam, V. K., Pande, C. B., Moharir, K. N., Varade, A. M., Rane, N. L., Egbueri, J. C., & Alshehri, F. (2023).
Prediction of sodium hazard of irrigation purpose using artificial neural network modelling.
Sustainability, 15(9), 7593.
[77] Aniza, R., Chen, W. H., Pétrissans, A., Hoang, A. T., Ashokkumar, V., & Petrissans, M. (2023). A review of
biowaste remediation and valorization for environmental sustainability: Artificial intelligence approach.
Environmental Pollution, 121363.
Journal of Advances in Artificial Intelligence 25 Volume 2, Number 1, 2024](https://d2z384uprhdr6y.cloudfront.net/YtOj3nr0d4_CV_2jowrAiGjQnYaQ25ND8_C1AuzWeS0/rt:fill/q:100/w:1280/h:0/gravity:sm/czM6Ly9qYXVudC1wcm9kdWN0aW9uLXVwbG9hZHMvMjAyNC8xMi8yNS8xMDE0ZTQ0OC03ZGViLTQyODMtYjRkMi03MDRlMGQ5OTI0Mzcvc2xpZGVfMjUtbC53ZWJw.webp)
![[78] Liao, M., & Yao, Y. (2021). Applications of artificial intelligence‐based modeling for bioenergy systems: A
review. GCB Bioenergy, 13(5), 774–802.
Copyright © 2024 by the authors. This is an open access article distributed under the Creative Commons
Attribution License which permits unrestricted use, distribution, and reproduction in any medium, provided
the original work is properly cited (CC BY 4.0).
Journal of Advances in Artificial Intelligence 26 Volume 2, Number 1, 2024](https://d2z384uprhdr6y.cloudfront.net/TgQ6yNTZYgdbo-VfwtHDCfGCwJByq3bLgRMRfKz6vE0/rt:fill/q:100/w:1280/h:0/gravity:sm/czM6Ly9qYXVudC1wcm9kdWN0aW9uLXVwbG9hZHMvMjAyNC8xMi8yNS8xMDE0ZTQ0OC03ZGViLTQyODMtYjRkMi03MDRlMGQ5OTI0Mzcvc2xpZGVfMjYtbC53ZWJw.webp)
Chat GPT uses 10 times as much Energy as Google Search -Energy Consumption Increase
- 1. Contribution of ChatGPT and Other Generative Artificial Intelligence (AI) in Renewable and Sustainable Energy Nitin Liladhar Rane Vivekanand Education Society's College of Architecture (VESCOA), Mumbai, India. Email: nitinrane33@gmail.com (N.L.R.) Manuscript submitted November 21, 2023; accepted November 28, 2023; published January 3, 2024 Abstract: In the dynamic field of renewable and sustainable energy, Artificial Intelligence (AI) integration has emerged as a crucial catalyst for enhancing efficiency, cost reduction, and addressing multifaceted challenges. This work delves into the performance and contributions of ChatGPT, a prominent representative of large AI language models, within the context of renewable and sustainable energy. The research endeavors to explore ChatGPT's efficacy and role across a diverse spectrum of renewable energy aspects, spanning solar energy, wind energy, biomass energy, hydropower, geothermal energy, energy storage, grid integration, energy efficiency, materials science, policy, economics, environmental impact, energy accessibility, and advanced biofuels. The investigation commences with solar energy, where ChatGPT showcases impressive capabilities in data analysis, modeling, and forecasting. It facilitates solar panel placement optimization, energy production prediction, and overall efficiency enhancement. Likewise, in the realm of wind energy, ChatGPT proves invaluable for tasks such as wind turbine control, maintenance scheduling, and wind speed prediction. In biomass energy, ChatGPT aids in feedstock selection, process optimization, and emissions reduction. In the hydropower sector, it supports reservoir management and river flow prediction, thereby optimizing energy output. Geothermal energy benefits from ChatGPT's geological analysis, reservoir modeling, and exploration strategies. Energy storage solutions are indispensable for grid stability, and ChatGPT contributes to the advancement of cutting-edge battery technologies, augmenting energy storage capacity and lifespan. Furthermore, ChatGPT's insights foster the evolution of smart grids, ensuring efficient energy distribution and effective demand management. Additionally, ChatGPT provides invaluable insights into policy and economics, offering recommendations for incentivizing the adoption of renewable energy sources while considering environmental impact and sustainability. It also contributes to the advancement of tidal and wave energy technologies, as well as the development of advanced biofuels. The outcomes of this research paper underscore the potential of AI and ChatGPT in shaping the future of renewable energy, expediting progress toward a sustainable energy ecosystem. Keywords: ChatGPT, artificial intelligence, generative AI, large language models, renewable energy, sustainable energy 1. Introduction In an age marked by unprecedented technological progress, the significance of artificial intelligence (AI) has grown substantially in addressing the world's most urgent challenges [1–4]. Among these challenges, the pursuit of sustainable and renewable energy sources emerges as a critical mission to combat climate change and ensure the long-term health of our planet [5–7]. This research paper explores the role and performance of advanced generative AI models like ChatGPT in the realm of renewable and sustainable energy. The rise of Journal of Advances in Artificial Intelligence 1 Volume 2, Number 1, 2024 DOI: 10.18178/JAAI.2024.2.1.1-26
- 2. such large language models has opened new avenues for enhancing our comprehension, decision-making, and innovation in this field. By scrutinizing their capabilities and limitations in comparison to other AI approaches, we aim to uncover the transformative potential of AI in expediting the transition to a greener and more sustainable energy landscape. The global energy landscape is undergoing a profound shift, driven by the urgent need to curtail greenhouse gas emissions and mitigate climate change's effects [8]. This transformation encompasses a diverse range of energy sources and technologies, including solar and wind energy, hydropower, geothermal energy, and advanced biofuels [9]. It also involves complex aspects such as energy storage, grid integration, energy efficiency, materials science, policy and economics, environmental impact, energy access, and equity. As we embark on this transformative journey, harnessing AI's power, especially through generative AI models like ChatGPT, becomes crucial to optimize, innovate, and accelerate the development and deployment of sustainable and renewable energy solutions. Solar energy is a promising and abundant renewable power source with the potential to revolutionize our energy landscape [10]. AI can boost the efficiency of photovoltaic systems, optimize the placement of solar panels, and forecast solar irradiance more accurately. Similarly, wind energy benefits from advanced AI algorithms that optimize turbine placement, predict wind patterns, and enhance maintenance procedures, ensuring a more reliable and efficient energy source. Biomass energy, hydropower, and geothermal energy also gain from AI applications that optimize plant operations and resource allocation for sustainable energy production. Energy storage plays a pivotal role in enabling renewable energy sources to mitigate intermittency and ensure a stable power supply [11]. AI-driven algorithms can optimize energy storage systems, maximizing their capacity and lifespan. Grid integration and the development of smart grids are crucial components of a sustainable energy future, and AI can manage the intricate dynamics of these systems. Furthermore, AI-driven advancements in energy efficiency, materials science, and nanotechnology have the potential to reduce energy consumption and enhance the performance of renewable energy technologies. Fig. 1 shows the co-occurrence analysis of the keywords. Fig. 1. Co-occurrence analysis of the keywords. AI also profoundly influences the policy and economics surrounding renewable energy [12, 13]. AI can Journal of Advances in Artificial Intelligence 2 Volume 2, Number 1, 2024
- 3. model various scenarios, analyze policy effectiveness, and predict the economic impacts of different energy strategies. In addressing environmental impact and sustainability, AI can assist in monitoring ecosystems, assessing the carbon footprint of energy production, and optimizing resource management to minimize harm to the environment. Energy access and equity are global imperatives, and AI can contribute to developing solutions that ensure marginalized communities have access to clean and affordable energy. Tidal and wave energy, often overlooked but highly promising, can also benefit from AI's data-driven insights and predictive modeling. Advanced biofuels, with their potential to reduce carbon emissions in transportation and industry, can be further optimized through AI-driven processes in biotechnology and chemical engineering. ChatGPT, as a representative of large language models, possesses remarkable generative capabilities that have already found applications across various domains. Its ability to generate human-like text and understand and respond to natural language inputs makes it a valuable tool for communication, knowledge generation, and problem-solving. However, its potential in renewable and sustainable energy remains relatively untapped. In this research paper, we explore ChatGPT's performance and contribution in enhancing our understanding of renewable energy technologies, aiding in decision-making processes, and fostering innovation in this critical field. AI systems, particularly large language models, have faced scrutiny and challenges related to bias, ethical considerations, and responsible AI deployment. Addressing these concerns is paramount to ensure that AI is leveraged responsibly and ethically in the pursuit of renewable and sustainable energy solutions. This research paper, structured around the multifaceted aspects of renewable and sustainable energy, aims to illuminate the transformative potential of AI, with a particular focus on ChatGPT, in addressing these global challenges. By examining the current state of AI applications in each of the specified focus areas and assessing the impact of ChatGPT alongside other AI models, we seek to provide insights into the role of generative AI in revolutionizing the renewable and sustainable energy landscape. Furthermore, we aim to highlight the ethical considerations and responsible AI practices that should guide our endeavors in harnessing AI's power for the betterment of planet and its inhabitants. Fig. 2 shows the co-authorship analysis. Fig. 2. Co-authorship analysis. In the subsequent sections of this research paper, we have delved into each of the focus areas in detail, Journal of Advances in Artificial Intelligence 3 Volume 2, Number 1, 2024
- 4. exploring the contributions and potential of AI models like ChatGPT. Additionally, we have delved into the ethical and responsible AI considerations that must accompany these advancements. Through this comprehensive analysis, we aspire to provide a holistic perspective on the symbiotic relationship between AI, renewable energy, and the sustainable future we all strive to achieve. 2. Contribution of ChatGPT in Solar Energy Generative artificial intelligence (AI) systems, such as ChatGPT, possess the capacity to make substantial contributions to the advancement of solar energy as a renewable and sustainable power source [14]. Solar energy harnesses the sun's energy to generate electricity and heat, offering a multitude of environmental and economic advantages [15, 16]. AI technologies can play a pivotal role in elevating the efficiency, dependability, and affordability of solar energy systems. Enhancing solar panel placement and design: One of the primary challenges in solar energy generation is the optimization of solar panel placement and design to maximize energy output [17]. AI, including generative models like ChatGPT, can facilitate this process. By scrutinizing geographical data, weather patterns, and local shading variables, AI can propose ideal sites for solar panel installations. Furthermore, AI can contribute to the creation of tailored solar panel configurations that consider unique architectural constraints and aesthetic preferences, thereby increasing the appeal of solar adoption for homeowners and businesses. Predictive maintenance and fault detection: AI can elevate the reliability of solar energy systems by means of predictive maintenance and fault detection [18]. Solar panels are subjected to various environmental stressors, leading to wear and tear over time. Generative AI can evaluate historical and real-time data from solar installations to predict maintenance needs, thereby reducing downtime and elevating energy production. Additionally, AI-powered algorithms can swiftly pinpoint and diagnose issues in solar panels, enabling technicians to promptly address them, minimizing energy losses, and extending equipment lifespan. Energy forecasting and grid integration: The effective integration of solar energy into the power grid necessitates precise energy forecasting [19, 20]. ChatGPT and comparable AI models can scrutinize historical weather data, solar panel performance data, and other pertinent information to forecast solar energy generation with exceptional accuracy. This information is invaluable for grid operators, allowing them to efficiently balance energy supply and demand. AI can also assist in optimizing the grid's response to fluctuations in solar generation, thereby ensuring a stable and dependable energy supply. 2.1.Energy Consumption Optimization Generative AI can be applied to optimize energy consumption in residential, commercial, and industrial settings [21, 22]. ChatGPT can contribute to the development of personalized energy management strategies, taking into account factors such as user schedules, energy-efficient appliances, and real-time electricity pricing. This can result in reduced energy costs, reduced reliance on fossil fuels, and an overall more sustainable energy consumption pattern. Advancing solar materials and technologies: AI can expedite the development of cutting-edge solar materials and technologies [23]. By analyzing extensive datasets and simulating various materials and designs, AI-driven research can pinpoint innovative materials with superior efficiency and durability. Generative models like ChatGPT can assist in the design and refinement of solar cells, aiding researchers in uncovering breakthroughs that render solar energy more accessible and cost-effective. 2.2.Energy Storage Solutions Journal of Advances in Artificial Intelligence 4 Volume 2, Number 1, 2024
- 5. The intermittent nature of solar energy poses a challenge; which AI can help address through the optimization of energy storage solutions like batteries [24]. Generative AI models can analyze historical energy consumption patterns and environmental data to ascertain the most efficient size, type, and configuration of energy storage systems. This ensures surplus solar energy is stored for use during overcast days or at night, reducing the reliance on backup fossil fuel power generation. 2.3.Policy and Market Insights AI can provide valuable insights into the policy and market dynamics surrounding solar energy. ChatGPT can analyze legislative documents, market trends, and public sentiment to assist policymakers in making informed decisions that bolster solar adoption. Additionally, AI can aid businesses in identifying market opportunities and investment strategies related to solar energy, ultimately promoting growth in the sector. 2.4.Customer Engagement and Education Effective communication plays a pivotal role in promoting the adoption of renewable energy sources. Generative AI can be employed to generate engaging and personalized content for consumers, aiding them in comprehending the advantages of solar energy and the steps involved in installation. AI-driven chatbots can answer queries, provide real-time estimates of energy savings, and guide potential customers through the decision-making process, making solar energy more accessible to a wider audience. 2.5.Environmental Impact Assessment AI can also contribute to the sustainability aspect of solar energy by evaluating its environmental footprint. Generative models can analyze data related to the manufacturing, installation, and operation of solar panels to identify areas where resource efficiency and carbon footprint can be improved. This information can guide the industry towards more sustainable practices, mitigating any negative environmental consequences associated with solar energy production. 2.6.Data security and Grid Resilience As solar energy systems become increasingly interconnected and reliant on digital infrastructure, AI can play a pivotal role in ensuring data security and grid resilience [25]. ChatGPT and similar AI models can aid in the development of robust cybersecurity protocols to safeguard solar installations from cyber threats. Additionally, AI can simulate and analyze grid scenarios to identify vulnerabilities and recommend strategies to enhance grid resilience against potential disruptions [26]. ChatGPT and generative AI technologies hold the potential to revolutionize the solar energy industry by addressing critical challenges and fostering innovation. From optimizing solar panel placement and design to enhancing energy forecasting, AI can enhance the efficiency, dependability, and affordability of solar energy systems. Furthermore, AI can support research into advanced materials and technologies, facilitate policy and market analysis, educate consumers, and assess the environmental impact of solar energy. As the world strives to transition to more sustainable energy sources, the synergy between AI and solar energy promises a greener and more sustainable future. Table 1 shows the role of ChatGPT and generative AI in solar energy. Table 1. Contribution of ChatGPT and Generative AI in Solar Energy Sr. No. Aspect of Solar Energy Contribution of ChatGPT and Generative AI Potential Benefits Challenges and Considerations 1 Solar Panel Design - Generating innovative solar panel designs by simulating various materials, shapes, and configurations to maximize efficiency and aesthetics. - Increased energy generation - Improved aesthetics and integration - Material feasibility - Manufacturing constraints 2 Energy Production - Utilizing historical data and weather patterns - Reduced energy - Data accuracy Journal of Advances in Artificial Intelligence 5 Volume 2, Number 1, 2024
- 6. Forecasting to provide accurate solar energy production forecasts, helping grid operators manage energy supply and demand efficiently. wastage - Better grid stability - Weather variability 3 Solar Farm Optimization - Optimizing the placement and orientation of solar panels in large solar farms to maximize energy output and minimize shading. - Higher energy yields - Land use efficiency - Site-specific considerations - Cost of reconfiguration 4 Efficiency Enhancement - Suggesting improvements in solar cell materials and coatings to increase energy conversion efficiency through materials science simulations. - Enhanced solar cell performance - Reduced manufacturing costs - Real-world validation - Time and resource constraints 5 Maintenance and Repairs - Assisting in identifying and diagnosing issues with solar panels through image analysis, reducing downtime and maintenance costs. - Minimized downtime - Cost savings - Data privacy - Sensor availability 6 Energy Storage - Recommending energy storage solutions based on energy generation patterns and demand forecasts, optimizing the use of batteries or other storage technologies. - Enhanced grid reliability - Lower energy costs - Battery degradation - Initial investment 7 Customer Engagement - Enhancing customer understanding of solar technology and benefits through AI-generated content, answering FAQs, and explaining complex concepts. - Increased adoption rates - Informed decision-making - Ethical use of AI - Privacy concerns 8 Policy and Regulations - Analyzing and summarizing policy documents, helping policymakers make informed decisions regarding solar energy incentives and regulations. - Streamlined policy development - Reduced ambiguity - Ethical considerations - Interpretation nuances 9 Supply Chain Optimization - Optimizing the supply chain for solar equipment, predicting demand fluctuations, and suggesting inventory management strategies. - Cost savings - Improved sustainability - Data accuracy - Supplier relationships 10 Environmental Impact Assessment - Assessing the environmental impact of solar projects, considering factors like land use, water usage, and emissions, to make informed decisions on project planning. - Sustainable project development - Regulatory compliance - Complex modeling - Data availability 11 Energy Market Integration - Providing insights into integrating solar energy into existing energy markets, considering pricing models, grid integration, and market dynamics. - Market competitiveness - Grid stability - Regulatory hurdles - Market volatility 12 Research and Development - Generating hypotheses and suggesting research directions for advancing solar technology, aiding scientists and engineers in their work. - Accelerated innovation - Targeted research efforts - Scientific validation - Resource allocation 13 Public Awareness Campaigns - Generating engaging and informative content for educational initiatives and public awareness campaigns to promote solar energy adoption. - Increased public support - Behavior change - Ethical messaging - Cultural differences 14 Data Analysis - Analyzing large datasets of solar energy production and consumption to identify trends, anomalies, and optimization opportunities. - Informed decision-making - Data-driven insights - Data privacy - Data quality 3. Role of ChatGPT in Wind Energy Generative artificial intelligence, such as ChatGPT, holds the promise of making substantial contributions to the renewable and sustainable energy sector, with a particular focus on wind energy [27, 28]. Wind energy plays a pivotal role in the worldwide transition toward cleaner and more sustainable power generation [29]. The utilization of wind to produce electricity offers a multitude of environmental and economic advantages, and AI-driven technologies stand poised to revolutionize wind energy production, rendering it more efficient, cost-effective, and ecologically friendly. One primary avenue through which ChatGPT and similar generative Journal of Advances in Artificial Intelligence 6 Volume 2, Number 1, 2024
- 7. AI models can impact the realm of wind energy is advanced forecasting and predictive modeling. Given that wind energy generation is heavily contingent on weather conditions, especially wind speed and direction, precise weather forecasts are essential for optimizing wind farm operations. Generative AI models, including ChatGPT, have the capability to analyze extensive historical weather data, current atmospheric conditions, and predictive models to generate highly accurate forecasts for wind energy production. These forecasts empower wind farm operators to make informed decisions regarding electricity generation, thereby reducing the intermittency typically associated with wind energy and enhancing grid stability. Furthermore, ChatGPT can provide real-time insights and recommendations based on continuously changing weather data. By processing this information, it can propose adjustments to turbine settings, such as rotor speed and blade pitch, to maximize energy capture during fluctuating wind conditions. This dynamic optimization can significantly boost the overall efficiency of wind energy production, rendering it a more dependable and appealing source of renewable energy. Beyond forecasting, generative AI can also assist in the design and layout of wind farms. The optimization of wind farm layouts is crucial for maximizing energy generation while minimizing adverse environmental impacts. AI models can scrutinize terrain data, wind patterns, and other geographic factors to suggest the most efficient placement of wind turbines within a given area. By optimizing turbine placement, developers can reduce the land footprint of wind farms, mitigate disruption to local ecosystems, and enhance overall energy output. Moreover, ChatGPT can facilitate the maintenance and operation of wind turbines. Wind turbine maintenance can be a costly and labor-intensive endeavor, often requiring routine inspections and repairs. AI-driven predictive maintenance models can analyze sensor data from turbines, including factors such as temperature, vibration, and oil quality, to predict when components may fail or require maintenance. Proactively scheduling maintenance can reduce downtime and extend the lifespan of turbines, ultimately yielding a higher return on investment for these renewable energy assets. Generative AI can also play a pivotal role in optimizing energy storage systems, a critical aspect of managing the intermittent nature of wind energy. AI models can scrutinize historical energy production data, demand patterns, and pricing information to make informed decisions about when to store excess energy and when to release it into the grid. This aids in balancing supply and demand, reducing wastage, and maximizing the economic feasibility of wind energy projects. Furthermore, ChatGPT can contribute to the development of innovative wind turbine designs and materials. AI-powered simulations can model and evaluate various turbine configurations, taking into account factors like aerodynamics, material durability, and environmental conditions. By rapidly iterating through design options, AI can assist engineers in identifying more efficient and cost-effective solutions, potentially leading to the creation of more powerful and long-lasting wind turbines. Another significant area where generative AI can make a substantial impact is in energy policy and regulation [30, 31]. ChatGPT can assist in the analysis of extensive volumes of legislative documents, research papers, and industry reports to provide insights into the latest developments in wind energy policy, regulatory changes, and market trends. This information can be invaluable for policymakers in making informed decisions concerning incentives, subsidies, and regulations that support the growth of wind energy as a sustainable energy source. Moreover, generative AI can contribute to public awareness and education about wind energy. ChatGPT can generate informative content, articles, and presentations to help elucidate the advantages of wind energy, dispel misconceptions, and address concerns. By providing accurate and accessible information, AI can play a pivotal role in shaping public perception and garnering support for wind energy projects. Table 2 shows the role of ChatGPT and AI in Wind Energy. Generative artificial intelligence, exemplified by ChatGPT, possesses the potential to transform the wind energy sector across various critical domains. It can enhance the precision of wind energy production forecasts, optimize wind farm layouts, streamline turbine maintenance and operation, aid in energy storage Journal of Advances in Artificial Intelligence 7 Volume 2, Number 1, 2024
- 8. management, stimulate innovative turbine design, inform energy policies, and promote public awareness. By harnessing the capabilities of AI, the wind energy industry can become more efficient, cost-effective, and environmentally sustainable, thereby making a significant contribution to the global transition to renewable and sustainable energy sources. Table 2. Role of ChatGPT and AI in Wind Energy Sr. No. Contribution Description Role of ChatGPT and AI in Wind Energy 1 Data Analysis AI can analyze extensive datasets from weather, turbine performance, and energy production to optimize wind farm operations and maintenance. ChatGPT assists in data interpretation and generates insights. AI conducts data processing and analysis. 2 Predictive Maintenance AI predicts equipment failures and maintenance needs in advance, reducing downtime and optimizing wind turbine performance. ChatGPT communicates maintenance predictions and provides explanations for AI-generated alerts. 3 Energy Forecasting AI models provide accurate short-term and long-term wind energy forecasts, aiding grid operators in effective energy supply planning. ChatGPT generates user-friendly reports and visualizations based on AI-generated forecasts for non-technical stakeholders. 4 Design Optimization AI-driven simulations optimize wind turbine designs for enhanced efficiency, improved energy capture, and reduced environmental impact. ChatGPT assists engineers in brainstorming design ideas and generating design documentation, while AI handles simulations and optimizations. 5 Wind Farm Layout AI helps choose optimal layouts for wind farms, considering wind patterns, terrain, and environmental factors to maximize energy generation. ChatGPT facilitates stakeholder discussions and generates layout recommendation reports, while AI conducts layout simulations and calculations. 6 Grid Integration AI manages the integration of wind energy into the electrical grid, ensuring stable power supply and minimizing grid congestion. ChatGPT explains grid integration strategies and benefits to policymakers and the public, while AI oversees grid optimization algorithms and control systems. 7 Energy Efficiency AI controls and optimizes wind turbine operation for maximum energy extraction from available wind resources, enhancing overall efficiency. ChatGPT provides insights into AI-driven control strategies and their efficiency impact, while AI executes real-time control decisions. 8 Maintenance Scheduling AI schedules maintenance based on real-time data, weather conditions, and turbine health, reducing costs and increasing uptime. ChatGPT assists in communicating maintenance schedules to field teams and generates maintenance outcome reports. AI generates scheduling recommendations. 9 Environmental Impact AI assesses and minimizes the environmental impact of wind energy projects, including wildlife conservation and noise pollution considerations. ChatGPT communicates environmental impact assessments to regulatory bodies and the public, addressing concerns and providing explanations, while AI performs impact assessments and modeling. 10 Customer Interaction Chatbots and AI-powered customer service provide information about wind energy benefits, incentives, and encourage adoption among consumers. ChatGPT serves as a conversational interface, answering questions, providing information, and guiding consumers through adoption processes. AI powers chatbot functionality. 11 Research Assistance AI assists researchers in wind energy-related studies by generating reports, analyzing research papers, and providing insights into the latest developments. ChatGPT helps researchers brainstorm ideas, generates research summaries, and explains complex concepts, while AI assists in data analysis and document generation. 12 Policy and Regulation AI analyzes policy documents and regulations, assisting governments and ChatGPT assists policymakers and legal experts in understanding and interpreting complex regulations Journal of Advances in Artificial Intelligence 8 Volume 2, Number 1, 2024
- 9. organizations in informed decisions to promote wind energy adoption and sustainability. and generates policy reports, while AI performs regulatory analysis and compliance checks. 4. Contribution of ChatGPT in Biomass Energy Generative artificial intelligence, exemplified by ChatGPT, possesses significant potential to advance the utilization of biomass energy as a sustainable and renewable energy source [32]. Biomass energy is harnessed from organic materials such as wood, agricultural residues, and waste products, rendering it a valuable renewable energy resource with the capacity to mitigate greenhouse gas emissions and reduce dependence on fossil fuels. 4.1.Biomass Resource Assessment and Prediction A central challenge in biomass energy production revolves around the dependable assessment and prediction of biomass resources [33]. AI algorithms, including ChatGPT, can analyze extensive data from diverse sources, encompassing satellite imagery, meteorological forecasts, and historical data, to estimate the availability and growth potential of biomass. This information proves indispensable for biomass power plants and biofuel manufacturers, facilitating efficient operational planning and ensuring a steady supply of feedstock. 4.2.Optimization of Biomass Supply Chain AI holds the capability to optimize the entire biomass supply chain, encompassing harvesting, transportation, storage, and processing. ChatGPT can contribute to scheduling and route planning for biomass collection and transportation, considering factors such as fuel efficiency, logistics costs, and environmental impact. Through supply chain streamlining, AI aids in waste reduction, energy conservation, and cost minimization. 4.3.Biomass Conversion Technologies The conversion of biomass into energy typically involves diverse technologies like combustion, gasification, and fermentation. AI can enhance the optimization of these processes by continuously analyzing real-time data from sensors and making adjustments to enhance efficiency and decrease emissions [34, 35]. For instance, AI can fine-tune combustion parameters in biomass power plants to optimize energy output while minimizing environmental repercussions. 4.4.Energy Production Forecasting AI models, akin to ChatGPT, can develop predictive models for biomass energy production. Through scrutiny of historical data, weather predictions, and operational variables, AI can forecast energy production levels, empowering grid operators and energy firms to adeptly integrate biomass energy into the electrical grid. Precise forecasts underpin grid stability and diminish the need for backup power sources. 4.5.Energy Demand Management AI also contributes to demand-side management in the realm of biomass energy. By analyzing consumer behavior and energy consumption patterns, AI aids in harmonizing the supply and demand for biomass energy. Smart grids infused with AI algorithms optimize energy distribution, ensuring judicious use of biomass energy and minimizing wastage. 4.6.Emissions Monitoring and Reduction Despite its renewable nature, biomass energy can still generate emissions if not managed meticulously. AI Journal of Advances in Artificial Intelligence 9 Volume 2, Number 1, 2024
- 10. stands poised to monitor emissions in real-time, employing sensors and data analysis to detect and mitigate pollutant levels. ChatGPT plays a role in data interpretation and decision-making, ensuring adherence to environmental regulations and reduction of the carbon footprint associated with biomass energy operations. 4.7.Research and Development AI can expedite research and development endeavors in the sphere of biomass energy. ChatGPT can scrutinize scientific literature, propose experiments, and even generate hypotheses for researchers. This facilitates the discovery of more efficient biomass conversion technologies, improved feedstock selection, and enhanced biomass-to-energy processes. 4.8.Predictive Maintenance AI-driven predictive maintenance systems enhance the reliability of biomass energy facilities. By analyzing data from sensors and equipment, AI can predict when maintenance is required, thus reducing downtime and averting costly breakdowns. This proactive approach to maintenance augments overall efficiency and longevity of biomass energy infrastructure. 4.9.Education and Outreach ChatGPT proves valuable in educating the public about the merits of biomass energy as a renewable and sustainable energy source. It can generate informative content, respond to inquiries, and engage individuals keen on learning more about biomass energy, thereby promoting its adoption and acceptance. 4.10. Policy and Decision Support AI provides invaluable insights and assistance to policymakers in shaping strategies and regulations concerning biomass energy. ChatGPT can analyze data, produce reports, and offer recommendations to facilitate informed decision-making regarding subsidies, incentives, and environmental standards for biomass energy initiatives. Generative artificial intelligence, such as ChatGPT, and analogous technologies stand poised to revolutionize the biomass energy sector. By optimizing resource assessment, supply chain management, energy production, emissions reduction, and research and development, AI can significantly augment the efficiency, sustainability, and overall viability of biomass energy as a renewable energy source. In a world where combating climate change and reducing fossil fuel reliance is imperative, the integration of AI into the biomass energy industry marks a promising stride towards a more sustainable and environmentally friendly energy future. 5. Contribution of ChatGPT in Hydropower Renewable and sustainable energy sources are paramount in combatting the global energy crisis and alleviating the consequences of climate change. Among these sources, hydropower, a well-established and widely adopted technology, stands out as a significant contributor to renewable energy production. It harnesses the kinetic energy of flowing water to generate electricity, providing a clean and reliable power source. However, optimizing, managing, and ensuring the environmental sustainability of hydropower systems present complex challenges [36, 37]. In this context, the utilization of ChatGPT and similar generative artificial intelligence (AI) models holds immense potential to transform the hydropower industry. 5.1.Utilizing Data for Informed Decision-Making One of the primary ways in which ChatGPT and generative AI can benefit the hydropower sector is through data-driven decision-making [38]. Hydropower plants generate vast quantities of data, encompassing Journal of Advances in Artificial Intelligence 10 Volume 2, Number 1, 2024
- 11. water flow rates, weather conditions, turbine efficiency, and grid demand. Analyzing this data is pivotal for fine-tuning energy production and guaranteeing system reliability. Generative AI models like ChatGPT can sift through this data, pinpoint patterns, and offer real-time recommendations to plant operators and engineers. For instance, ChatGPT can scrutinize historical data to predict water flow patterns, allowing operators to anticipate fluctuations in power generation and optimize reservoir management. Furthermore, it can offer insights into turbine performance, suggesting maintenance schedules to maximize efficiency and minimize downtime. Leveraging AI-driven analytics, the hydropower sector can amplify energy output and trim operational costs, thereby fostering a more sustainable and dependable energy supply. 5.2.Assessing Environmental Impact Hydropower projects often undergo scrutiny due to their environmental impact on river ecosystems, aquatic life, and local communities [39]. Generative AI can play a pivotal role in evaluating and mitigating these effects. ChatGPT can simulate the ecological consequences of various hydropower operation scenarios, aiding policymakers and project developers in making informed decisions. By simulating scenarios and analyzing potential outcomes, generative AI can help identify the most ecologically sustainable operating strategies, such as seasonal flow variations or the creation of fish-friendly fish ladders and bypass channels. Additionally, AI can assist in monitoring and mitigating the effects of climate change on river systems, which can impact hydropower generation reliability. In this manner, ChatGPT and similar AI models contribute to making hydropower a more environmentally responsible form of renewable energy. 5.3.Enhancing Predictive Maintenance The reliability of hydropower plants is vital for their long-term sustainability. Unforeseen equipment failures can disrupt energy production and result in costly downtime. Predictive maintenance, facilitated by generative AI, can significantly reduce these risks. Generative AI models like ChatGPT can analyze sensor data from turbines, generators, and other critical components of hydropower plants. By recognizing wear patterns and potential failure indicators, AI can forecast maintenance needs well in advance. Plant operators can then schedule maintenance during periods of low demand or favorable weather conditions, minimizing disruptions to energy production. This proactive approach not only bolsters reliability but also prolongs the lifespan of hydropower equipment, rendering the energy source more sustainable in the long run. 5.4.Addressing Energy Storage and Grid Integration Challenges ChatGPT and generative AI can also aid in confronting one of the key challenges of hydropower generation: energy storage and grid integration. Hydropower often experiences intermittency, reliant on water flow, which can fluctuate due to seasonal and weather variations. Generative AI can optimize the incorporation of hydropower into the broader energy grid by predicting energy generation and consumption patterns. For instance, ChatGPT can analyze historical data to predict periods of high energy demand and low hydropower generation. It can then recommend the utilization of energy storage solutions like pumped hydro storage or advanced battery systems to store surplus energy during abundance and release it when demand peaks. This enhances hydropower's reliability as a sustainable energy source, ensuring a consistent energy supply for consumers. 5.5.Facilitating Infrastructure Planning and Design Developing new hydropower projects necessitates meticulous planning and design to maximize energy generation while minimizing environmental impacts. ChatGPT and generative AI can provide assistance in this process by simulating various project scenarios and assessing their feasibility. These AI models can scrutinize topographical data, water flow patterns, and environmental constraints to propose optimal dam Journal of Advances in Artificial Intelligence 11 Volume 2, Number 1, 2024
- 12. locations and reservoir sizes [40]. They can also contribute to designing efficient and environmentally friendly fish passage systems. By offering precise, data-driven insights, AI can assist project developers in making informed decisions that strike a balance between energy generation and environmental sustainability. 5.6.Improving Energy Forecasting and Market Optimization To ensure the efficient utilization of hydropower resources, it is imperative to accurately forecast energy generation and market conditions. Generative AI can be leveraged to construct sophisticated forecasting models that account for factors such as weather, river flow, and grid demand. ChatGPT can scrutinize historical data and meteorological information to provide short-term and long-term energy generation forecasts. These forecasts can be employed by energy market participants to optimize their operations, encompassing energy trading and dispatch. By precisely predicting energy supply and demand, AI-driven forecasting can enhance the economic viability of hydropower projects, rendering them more competitive in the energy market. 5.7.Fostering Human-Machine Collaboration Although AI can autonomously perform various tasks, human expertise remains invaluable in the hydropower industry. ChatGPT and similar AI models can facilitate collaboration between humans and machines, thereby enhancing decision-making and problem-solving. For instance, plant operators and engineers can interact with ChatGPT to access real-time data, receive recommendations, and simulate different operational scenarios. This partnership between humans and machines enables quicker and more informed decisions, ultimately elevating the overall efficiency and sustainability of hydropower plants. Hydropower has long been acknowledged as a reliable and sustainable source of renewable energy. Nevertheless, its full potential remains untapped, with challenges related to data analysis, environmental impact assessment, equipment reliability, and grid integration persisting. Generative AI models like ChatGPT offer innovative solutions to address these challenges and unlock the true potential of hydropower as a clean and renewable energy source. By enabling data-driven decision-making, predicting maintenance needs, assessing environmental impacts, optimizing energy storage and grid integration, and aiding in infrastructure planning and design, ChatGPT and generative AI can revolutionize the hydropower industry. These technologies facilitate a more sustainable and efficient use of hydropower resources, contributing to the global transition to cleaner energy sources and the mitigation of climate change. As the world continues to prioritize renewable energy, the role of AI in advancing hydropower cannot be understated, paving the way for a more sustainable and resilient energy future. 6. Role of ChatGPT in Geothermal Energy Artificial Intelligence models like ChatGPT have the potential to play a significant role in advancing the field of geothermal energy, elevating its status as a reliable and sustainable source of renewable power [41]. Geothermal energy taps into the Earth's inherent heat from its core, offering a consistent and environmentally friendly energy supply. Despite its immense potential, the efficient and sustainable utilization of geothermal energy faces numerous challenges. AI technologies, including ChatGPT, hold promise in effectively addressing these challenges by aiding in resource assessment, exploration, optimization, and the overall management of geothermal energy systems. One of the primary ways in which ChatGPT and similar AI models can contribute to geothermal energy is by enhancing resource assessment and exploration [42, 43]. The identification of suitable geothermal reservoirs is paramount for successful geothermal project development. AI models excel at analyzing extensive geological data, encompassing Journal of Advances in Artificial Intelligence 12 Volume 2, Number 1, 2024
- 13. parameters such as subsurface temperature gradients, rock permeability, and seismic data, to pinpoint potential geothermal reservoirs with high energy-producing potential. These AI models can process vast datasets more swiftly and accurately than human experts, assisting geologists and geophysicists in determining the optimal locations for drilling geothermal wells. Furthermore, AI can significantly optimize drilling operations, which are intricate and costly processes when reaching deep into the Earth's crust to access geothermal reservoirs. AI algorithms can fine-tune drilling parameters, including drill bit selection, drilling speed, and well trajectory, resulting in increased drilling efficiency and cost reduction. Additionally, AI can predict potential drilling challenges by analyzing historical drilling data and geological information, enabling proactive measures to mitigate risks and minimize downtime [40, 44]. ChatGPT and analogous AI models also facilitate reservoir management in geothermal energy systems. Once a geothermal well becomes operational, maintaining efficient energy production becomes imperative. AI continuously monitors well performance by scrutinizing real-time data such as temperature, pressure, and flow rates. By identifying anomalies and patterns within the data, AI can offer early warnings of potential issues, allowing operators to take prompt corrective actions and maximize energy production. Another crucial role for AI lies in optimizing the overall geothermal energy production process. AI-driven predictive maintenance can reduce equipment downtime by anticipating when components such as pumps, turbines, and heat exchangers are likely to fail. This enables scheduled maintenance rather than unexpected breakdowns, ensuring uninterrupted energy generation. Furthermore, AI can enhance the efficiency of geothermal power plants by optimizing power generation processes. Machine learning algorithms can analyze historical operational data to uncover opportunities for enhancing energy conversion and heat transfer processes. This optimization can lead to increased energy output and reduced operational costs, making geothermal energy even more competitive among other renewable energy sources. Geothermal reservoirs can be intricate, and their behavior may evolve over time. AI models assist in reservoir monitoring and management by analyzing data from multiple sensors positioned within the reservoir, encompassing measurements of temperature, pressure, and chemical composition. AI can detect subtle changes in reservoir conditions, aiding operators in making informed decisions to maximize reservoir longevity and sustain energy production. Additionally, ChatGPT and similar AI models can serve as valuable tools in geothermal energy education and public outreach. AI-powered chatbots and virtual assistants can disseminate information to the public about geothermal energy, its advantages, and its environmental impact. These AI tools can answer inquiries and promote awareness of geothermal energy as a sustainable and dependable energy source [40, 45, 46]. ChatGPT and akin Generative Artificial Intelligence models hold immense potential for advancing geothermal energy as a renewable and sustainable energy source. They contribute to resource assessment, exploration, drilling optimization, reservoir management, predictive maintenance, and process optimization. As technology continues to advance, the synergy between AI and geothermal energy will likely yield even more innovative solutions and deeper integration of geothermal power into the global energy landscape. 7. Contribution of ChatGPT in Energy Storage Artificial intelligence models hold the potential to make substantial contributions to the realm of energy storage for sustainable and renewable energy sources [47–49]. Here's how ChatGPT and analogous AI models can play a central role in advancing energy storage: Optimizing energy storage systems: AI's primary contribution to energy storage lies in optimization [47, 50]. AI models, including ChatGPT, can analyze copious amounts of data from diverse sources, encompassing weather patterns, energy demand fluctuations, and historical energy production data. By forecasting periods Journal of Advances in Artificial Intelligence 13 Volume 2, Number 1, 2024
- 14. of peak energy production and demand, AI can facilitate the efficient management of energy storage. This ensures that surplus energy generated during high-production periods is stored effectively for use during peak demand, thus optimizing the overall energy ecosystem. Advancing battery technology: AI can greatly assist in the research and enhancement of battery technology, a pivotal element of energy storage systems [51, 52]. AI models can simulate various chemical compositions and structural configurations, identifying materials that offer superior energy density, longer life cycles, and faster charging capabilities. This expedited material discovery process can result in the creation of more efficient and cost-effective energy storage solutions, ultimately diminishing dependence on fossil fuels. Predictive maintenance: AI can extend the longevity and reliability of energy storage systems by predicting maintenance requirements. Continuous monitoring and data analysis by AI models can detect potential issues, enabling scheduled maintenance before they escalate into critical problems. This proactive approach minimizes downtime and ensures energy storage systems operate at peak performance, boosting overall efficiency. Grid management: Renewable energy sources often generate power intermittently, posing a challenge for grid management. AI can assist in balancing supply and demand by forecasting energy generation and consumption patterns. ChatGPT can engage in natural language conversations with grid operators, delivering real-time insights and recommendations for effective grid management. This dynamic decision support can optimize the use of energy storage systems, stabilizing the grid and diminishing reliance on fossil fuels. Energy forecasting: Precise energy production and consumption forecasting are imperative for the planning and management of renewable energy systems. AI models like ChatGPT can analyze historical data, weather patterns, and other pertinent factors to furnish accurate energy production forecasts. This information empowers grid operators and energy providers to make informed decisions regarding the utilization of energy storage systems, reducing waste and maximizing renewable resource utilization. Demand response: AI-powered chatbots, such as ChatGPT, can interact with consumers to promote sustainable energy practices. By providing real-time information on energy demand, pricing, and availability, AI can incentivize consumers to shift their energy consumption to periods when renewable energy is abundant and cost-effective. This demand response mechanism can diminish the necessity for additional energy storage capacity during peak demand periods. Policy and regulation support: AI can also lend a hand in the formulation of policies and regulations that foster the adoption of renewable energy and energy storage technologies. ChatGPT can scrutinize extensive volumes of legislative documents, scientific literature, and industry reports to offer policymakers valuable insights and recommendations for crafting effective policies that encourage the adoption of sustainable energy solutions. 8. Contribution of ChatGPT in Grid Integration and Smart Grids Generative artificial intelligence (AI) models hold significant promise in advancing grid integration and smart grids for sustainable and renewable energy. They offer a range of applications that can boost the efficiency, dependability, and eco-friendliness of energy systems [53, 54]. One pivotal area where ChatGPT and similar AI models can make a substantial impact is in demand forecasting and optimization. Smart grids rely heavily on precise predictions of energy demand to optimize resource allocation, enhance grid stability, and reduce energy wastage. ChatGPT can effectively analyze historical data, weather patterns, and other pertinent information to generate accurate forecasts of energy demand. These forecasts, in turn, assist grid operators in making well-informed decisions regarding the allocation of renewable energy resources, such as wind and solar power, to meet expected demand levels. By decreasing reliance on fossil fuels and optimizing energy distribution, AI-powered demand forecasting contributes significantly to the creation of a more Journal of Advances in Artificial Intelligence 14 Volume 2, Number 1, 2024
- 15. sustainable energy grid. Table 3 shows the role of ChatGPT in grid integration and smart grids. Moreover, AI plays a crucial role in real-time grid management by continuously analyzing grid data [55, 56]. Smart grids generate vast amounts of data from diverse sensors and devices, and the ability to process this data in real time is vital for maintaining grid stability. AI models can swiftly analyze this data, detecting anomalies or grid issues and suggesting corrective measures. For instance, if a sudden drop in solar power production occurs due to cloud cover, ChatGPT can recommend boosting output from other renewable sources or scaling up energy storage systems to compensate. Such real-time decision-making enhances the reliability and resilience of the grid, particularly in coping with the intermittent nature of renewable energy sources. Furthermore, ChatGPT can elevate energy management at the consumer level. Utilizing chatbots or virtual assistants, AI can interact with consumers and offer tailored suggestions for energy conservation and optimization. It can recommend adjustments to thermostat settings, optimize the charging of electric vehicles to coincide with peak renewable energy production, or even schedule energy-intensive tasks like laundry during off-peak hours. These personalized recommendations empower consumers to reduce their energy bills and contribute to load balancing on the grid. Table 3. Role of ChatGPT in Grid Integration and Smart Grids Sr. No. Contribution Description Benefits Limitations 1 Information Dissemination Real-time updates on grid tech & regs. Enhanced awareness Reliability of information 2 Education and Training Tool for smart grid education Knowledge transfer Limited interaction 3 Problem Solving Troubleshooting grid issues Rapid issue resolution Complex, unique problems 4 Data Analysis Identifying patterns & anomalies Data-driven insights Data quality, privacy concerns 5 Policy Insights Policy recommendations Informed decision-making Interpretation of regulations 6 Simulation and Modeling Grid scenario simulation & modeling Improved planning Model accuracy, complexity 7 Energy Management Energy efficiency recommendations Cost reduction Real-time adaptation required 8 Customer Engagement Customer inquiries & info provision Enhanced satisfaction Limited emotional intelligence 9 Regulatory Compliance Compliance support for regs & standards Reduced non-compliance risk Interpretation of regulations 10 Forecasting Predictions on demand & grid stability Improved resource allocation Prediction accuracy AI models also play a pivotal role in predicting equipment failures and optimizing maintenance within the renewable energy sector. Wind turbines, solar panels, and other renewable energy infrastructure require regular maintenance for peak performance. By analyzing sensor data and historical maintenance records, ChatGPT can predict when maintenance is required and recommend cost-effective scheduling for repairs. This proactive approach reduces downtime, extends the lifespan of renewable energy assets, and ultimately bolsters the sustainability of the energy infrastructure. Additionally, AI-driven predictive maintenance extends to the grid infrastructure itself. Smart grids encompass various components, including transformers, substations, and power lines. ChatGPT can analyze data from these components to anticipate potential failures or inefficiencies, enabling grid operators to take preventive measures and prevent costly disruptions. In grid integration, AI models streamline the seamless incorporation of distributed energy resources (DERs) into the grid. DERs, such as rooftop solar panels and home energy storage systems, can locally Journal of Advances in Artificial Intelligence 15 Volume 2, Number 1, 2024
- 16. generate and store electricity. AI optimizes the coordination of these resources, ensuring they inject surplus energy back into the grid when needed and charge when renewable energy generation is high. This flexibility in managing DERs enhances grid reliability and diminishes the need for centralized fossil fuel power plants. Furthermore, ChatGPT can supports grid operators in integrating electric vehicles (EVs) into the energy ecosystem. With the increasing adoption of EVs, managing their charging and discharging patterns is critical for grid stability [57]. AI provides EV owners with recommendations on optimal charging times to align with renewable energy availability, alleviating the strain on the grid during peak demand. ChatGPT and analogous generative AI models have the potential to transform grid integration and smart grids for renewable and sustainable energy. By enhancing demand forecasting, real-time grid management, consumer engagement, predictive maintenance, and the integration of DERs and EVs, these technologies substantially improve the efficiency, reliability, and sustainability of energy systems. As renewable energy sources continue to play a central role in the transition to a greener future, AI-driven solutions are indispensable in ensuring the seamless integration of these resources into the grid. 9. Role of ChatGPT in Energy Efficiency Generative Artificial Intelligence (AI), exemplified by models like ChatGPT, holds substantial promise for enhancing energy efficiency within the realm of renewable and sustainable energy [58, 59]. These AI systems can assume a pivotal role in streamlining various facets of energy production, consumption, and management, thus propelling the evolution towards a more sustainable and environmentally conscious energy ecosystem. A primary avenue through which ChatGPT and akin AI models can bolster energy efficiency revolves around data analysis and forecasting. These AI systems excel at processing copious real-time data, a crucial capability for renewable energy sources like solar and wind. They can scrutinize meteorological patterns, historical energy production data, and consumption trends to furnish precise predictions. For instance, ChatGPT can predict optimal periods for renewable energy generation and anticipate peak demand times. Such predictive prowess enables superior planning and utilization of renewable energy resources, mitigating energy wastage and optimizing production. Moreover, ChatGPT can aid in optimizing energy distribution and grid management [60, 61]. It can scrutinize regional demand patterns and proffer suggestions for tailoring the distribution of renewable energy resources accordingly. Through intelligent routing of energy to high-priority areas, AI can curtail transmission losses and ensure judicious use of renewable energy. Additionally, AI can oversee the well-being and performance of grid infrastructure, promptly identifying issues such as equipment malfunctions or voltage fluctuations. This proactive maintenance can forestall energy losses and power outages, elevating overall energy efficiency. Furthermore, ChatGPT can elevate the efficiency of renewable energy systems themselves. In the case of solar panels, AI can continually fine-tune panel angles and orientations to harness the maximum sunlight. Similarly, for wind turbines, AI can adjust rotor speed and direction based on wind conditions to optimize energy generation while minimizing wear and tear. These optimizations have the potential to significantly augment the energy output of renewable sources, rendering them more competitive with fossil fuels. AI can also play an indispensable role in energy conservation and demand-side management [62]. It can engage with users to furnish real-time energy-saving recommendations, such as optimizing thermostat settings, managing lighting, or scheduling appliance usage during off-peak hours. By imparting personalized energy-saving advice, AI can help curtail energy consumption at the individual and household levels, thus contributing to overall energy efficiency. Additionally, AI-driven chatbots like ChatGPT can enhance energy efficiency in industrial and commercial settings. They can aid in monitoring and regulating intricate systems, such as manufacturing processes or building HVAC systems, to minimize energy wastage. Furthermore, Journal of Advances in Artificial Intelligence 16 Volume 2, Number 1, 2024
- 17. these chatbots can assist companies in identifying opportunities for energy efficiency enhancements and offer counsel on sustainable practices, ultimately slashing energy costs and environmental impact. In the arena of energy research and development, ChatGPT can expedite innovation. It can analyze extensive datasets concerning renewable energy technologies, materials, and scientific investigations, facilitating researchers in discerning promising avenues for advancing energy efficiency and sustainability. By supporting scientists and engineers in generating novel ideas and hypotheses, AI can expedite the formulation of groundbreaking technologies in the realm of renewable energy. Nonetheless, it is imperative to acknowledge the challenges and ethical considerations entailed in deploying AI within the energy sector. Concerns like data privacy, cybersecurity, and algorithmic bias must be addressed to ensure the responsible integration of AI into energy efficiency initiatives. Furthermore, the transition towards a more sustainable energy paradigm necessitates collaboration among governments, industries, and AI developers to ensure that AI technologies align with broader environmental and societal objectives. Generative AI such as ChatGPT holds the potential to revolutionize energy efficiency within renewable and sustainable energy. Through data analysis, predictive capabilities, grid optimization, and energy conservation, AI can make substantial contributions towards diminishing energy wastage and optimizing the advantages of renewable energy resources. As AI technology continues to progress and intertwine with the energy sector, it has the potential to expedite the transition towards a more sustainable and ecologically friendly energy landscape, thus aiding in the battle against climate change and securing a cleaner energy future. 10. Contribution of ChatGPT in Materials Science and Nanotechnology Generative artificial intelligence, exemplified by models like ChatGPT offers significant promise in advancing the realms of Materials Science and Nanotechnology for Renewable and Sustainable Energy. These cutting-edge technologies hold the potential to make substantial contributions by expediting research, innovating materials, optimizing energy systems, and promoting communication and collaboration among scientists and researchers in these domains [63, 64]. One of the primary avenues through which AI models like ChatGPT can make impactful contributions to Materials Science and Nanotechnology is through their ability to swiftly analyze and predict material properties. Within these fields, researchers often face the formidable task of exploring an extensive array of materials to identify those suited for applications in renewable and sustainable energy. AI can play a pivotal role by simulating the behaviors of diverse materials under varying conditions, thereby saving valuable time and resources. With its vast knowledge base, ChatGPT can assist researchers in formulating hypotheses and suggesting potential materials for specific purposes, thus expediting the initial phases of material discovery. Moreover, AI can optimize the design of materials at the nanoscale, a pivotal aspect of enhancing the efficiency and sustainability of energy-related devices like solar cells and batteries [65, 66]. ChatGPT can aid in the creation of nanostructures with precise properties, assisting scientists in fabricating materials that enhance energy conversion and storage. By considering crucial factors such as size, shape, composition, and surface properties, AI models can contribute to the development of materials that maximize energy output while minimizing their environmental impact. Beyond materials design, AI can also play a pivotal role in the sustainable energy sector by streamlining manufacturing processes. ChatGPT can offer recommendations for more efficient and environmentally friendly manufacturing methods, ultimately leading to reductions in waste and energy consumption during production. This, in turn, can result in cost savings and a diminished carbon footprint, aligning seamlessly with the objectives of sustainable energy production. Furthermore, collaboration and the dissemination of knowledge are integral components of materials science and nanotechnology. ChatGPT and analogous AI models can function as potent communication tools for scientists on a global scale. They can facilitate discussions, provide answers to inquiries, and even assist in the Journal of Advances in Artificial Intelligence 17 Volume 2, Number 1, 2024
- 18. preparation of research papers. This streamlined mode of communication can accelerate the pace of scientific discovery by enabling researchers to share ideas, findings, and best practices with increased efficiency. Moreover, AI can surmount language barriers, enabling researchers from diverse linguistic backgrounds to collaborate seamlessly. By offering real-time translation and interpretation services, AI models like ChatGPT promote international cooperation, an essential element in addressing global energy challenges collectively. AI-powered virtual laboratories represent another promising avenue in this context. These virtual environments empower researchers to conduct experiments and simulations remotely, making it easier to access costly equipment and resources. ChatGPT can assist scientists in configuring and executing virtual experiments, interpreting results, and suggesting necessary adjustments. This democratizes access to advanced research facilities and enhances the efficiency of experiments. Furthermore, AI can contribute to sustainable energy by optimizing energy systems and grid management. ChatGPT can aid in forecasting energy demand and supply, optimizing grid operations, and proposing strategies for the seamless integration of renewable energy sources like solar and wind into existing energy infrastructures. By enhancing energy efficiency and grid stability, AI contributes to the reduction of greenhouse gas emissions and fortifies the sustainability of energy systems. Models like ChatGPT, alongside similar Generative AI models, have the potential to usher in a revolution in materials science and nanotechnology for renewable and sustainable energy. They have the capacity to expedite the discovery of materials, refine design at the nanoscale, enhance manufacturing processes, facilitate global collaboration, and improve energy system management. 11. Contribution of ChatGPT in Policy and Economics Generative artificial intelligence models like ChatGPT offer immense potential to make substantial contributions to the realms of renewable and sustainable energy policy and economics. These AI systems have the capacity to empower informed decision-making, foster innovation, and optimize efficiency in the pursuit of a more sustainable energy future. A primary avenue through which generative AI can bolster renewable and sustainable energy policy is by furnishing policymakers and stakeholders with invaluable insights and recommendations. These AI models possess the capability to sift through vast datasets drawn from diverse sources, including scientific literature, energy production statistics, and environmental reports. By processing this trove of information, ChatGPT can generate comprehensive reports and succinct summaries that illuminate crucial trends, pinpoint emerging technologies, and proffer potential policy interventions. Furthermore, these AI systems can aid in scenario analysis and simulation. Policymakers can input various variables, such as adoption rates of renewable energy sources, carbon pricing mechanisms, and energy efficiency measures, into ChatGPT, which can then produce forecasts and simulations of potential outcomes. This approach facilitates a more data-driven and evidence-based approach to policy formulation, ensuring that decisions are underpinned by a holistic comprehension of the intricate dynamics within the energy sector. Beyond providing insights, ChatGPT can augment public engagement and education regarding renewable energy. It can generate user-friendly content in the form of articles, infographics, and videos to elucidate the advantages of renewable energy technologies, the environmental repercussions of fossil fuels, and the economic prospects within the clean energy sector. This outreach plays a vital role in cultivating public support for sustainable energy policies and initiatives, which frequently prove indispensable for their effective implementation. Moreover, generative AI can serve as a linchpin in economic analysis pertinent to renewable energy. It can aid in conducting cost-benefit analyses, evaluating the economic viability of renewable energy ventures, and modeling the potential for job creation and economic expansion associated Journal of Advances in Artificial Intelligence 18 Volume 2, Number 1, 2024
- 19. with the clean energy sector. These insights are of paramount importance to both governmental and private sector decision-makers, as they assist in the judicious allocation of resources and the identification of investment opportunities aligned with sustainability objectives. Another noteworthy contribution of ChatGPT and analogous AI models lies in the optimization of energy systems [67, 68]. The integration of renewable energy sources into the grid presents challenges owing to their intermittency and variability. AI can be instrumental in managing these challenges by optimizing energy generation, storage, and distribution. For instance, AI can anticipate energy demand patterns and adjust renewable energy production accordingly, thereby curtailing energy wastage and ensuring a stable supply. This optimization not only fosters sustainability but also translates into long-term cost savings. AI-driven analytics can further aid in the identification and prioritization of renewable energy projects. By analyzing geographic, meteorological, and infrastructure data, these systems can proffer recommendations on the most suitable locations for solar farms, wind turbines, and other renewable energy installations. This streamlines the decision-making process for investors and mitigates the risks associated with project development. Furthermore, generative AI can facilitate international collaboration on renewable energy initiatives. Climate change and energy sustainability are global challenges that necessitate cooperation among nations. ChatGPT can assist in the translation of documents and discussions related to renewable energy policy and economics, simplifying the sharing of knowledge and best practices among experts and policymakers from diverse countries. It can also facilitate cross-cultural communication and negotiation, fostering international accords and initiatives aimed at sustainable energy development. 12. Contribution of ChatGPT in Tidal and Wave Energy Artificial Intelligence models such as ChatGPT hold significant promise in advancing the field of tidal and wave energy production, contributing to renewable and sustainable energy solutions [69, 70]. Tidal and wave energy sources harness the natural motion of oceans and seas, and AI stands poised to play a pivotal role in optimizing their efficiency and viability. A fundamental avenue through which ChatGPT and similar AI models can make a meaningful impact in the realm of tidal and wave energy is through data analysis and predictive capabilities. These AI models excel at processing and interpreting vast datasets, a critical requirement for comprehending the intricate behavior of tides and waves across different geographical regions. By delving into historical tidal and wave data, AI systems can identify patterns and trends, empowering energy companies and researchers to enhance their capacity to forecast optimal energy generation conditions. This predictive prowess is invaluable for strategizing energy generation and grid integration, ensuring a steady and consistent supply of renewable energy. Moreover, ChatGPT can lend its expertise to the design of more efficient and cost-effective wave and tidal energy systems. AI-driven simulations can replicate the behavior of diverse device configurations, fine-tuning factors like shape, size, and placement to maximize energy capture. This iterative design process can substantially reduce the trial-and-error phase in technology development, expediting progress within the field. Additionally, AI can scrutinize and enhance the materials employed in these systems, augmenting their durability and resilience in challenging marine environments, ultimately curbing maintenance expenses and prolonging the lifespan of these renewable energy installations. AI-driven predictive maintenance represents another invaluable contribution to tidal and wave energy. The upkeep of offshore energy equipment can be both expensive and logistically complex. AI can continuously monitor the condition of equipment, sensors, and other components, foreseeing when maintenance or repairs are required. This proactive approach can help avert costly downtimes and ensure the longevity of these installations, enhancing the reliability and economic feasibility of tidal and wave energy solutions. ChatGPT and analogous AI models can also elevate the control systems governing tidal and wave energy converters. These systems must adapt swiftly to Journal of Advances in Artificial Intelligence 19 Volume 2, Number 1, 2024
- 20. ever-changing oceanic conditions to optimize energy capture. AI can process real-time sensor data and make instantaneous decisions to fine-tune device settings for peak efficiency. This dynamic control can yield higher energy yields, especially in challenging marine environments characterized by unpredictable wave patterns. Furthermore, AI can simplify the integration of tidal and wave energy into existing power grids [71]. These renewable energy sources often generate power intermittently, contingent upon oceanic conditions. AI algorithms can manage this variable energy output, coordinating with other renewables such as wind and solar, as well as storage systems like batteries. This ensures a dependable and steady energy supply, diminishing dependence on fossil fuels and contributing to a more sustainable energy mix. ChatGPT and analogous AI models can also assist in addressing environmental concerns linked to tidal and wave energy. Through data analysis pertaining to the impact of these energy systems on marine ecosystems, AI can facilitate the development of mitigation strategies aimed at minimizing harm to marine life. This proactive approach ensures that renewable energy projects are not just sustainable but also environmentally responsible. 13. Contribution of ChatGPT in Advanced Biofuels Generative artificial intelligence (AI), as exemplified by models like ChatGPT, holds significant promise in advancing the realm of biofuels for sustainable and renewable energy [72, 73]. Biofuels are pivotal in our transition to a more environmentally friendly and sustainable energy landscape, offering a renewable alternative to fossil fuels. AI technologies, such as ChatGPT, can play a pivotal role in various aspects of biofuel development, encompassing optimization of production processes, resource utilization enhancement, and progress in research within this domain. One of the primary domains where ChatGPT and analogous generative AI systems can make substantial contributions is in the optimization of biofuel production processes. The production of biofuels involves intricate biochemical reactions, and enhancing these processes is critical for improving yields and reducing costs. Generative AI can assist in this optimization by scrutinizing extensive datasets and pinpointing ideal conditions for fermentation, enzymatic reactions, and other steps involved in biofuel production. By harnessing machine learning algorithms, AI models can empower researchers and engineers to discover more efficient and cost-effective techniques for converting biomass into biofuels. Moreover, ChatGPT can facilitate real-time monitoring and control of biofuel production processes. It can process and analyze data from sensors deployed in bioreactors, fermentation tanks, and other equipment to ensure seamless production. This AI-driven monitoring can swiftly detect anomalies and deviations from optimal conditions, enabling timely interventions to prevent costly disruptions and enhance overall production efficiency. Consequently, the reliability and consistency of biofuel production can be elevated, bolstering its scalability and commercial viability. Generative AI can also play a pivotal role in designing novel biofuels and optimizing existing biofuel formulations. Researchers can employ AI-powered simulations and molecular modeling to predict biofuel properties and performance, including aspects like energy density, combustion efficiency, and environmental impact. Through the generation and analysis of extensive datasets containing chemical structures and properties, AI models can propose promising candidates for biofuel development, potentially leading to the discovery of more efficient and sustainable biofuels. Another significant avenue where generative AI can contribute to advanced biofuels is in the optimization of feedstock selection and resource utilization [74, 75]. The choice of biomass feedstock holds utmost importance in biofuel production, impacting both environmental sustainability and economic feasibility. AI models can analyze geographical and climatic data, along with factors like biomass availability and growth rates, to identify the most suitable feedstock options for specific regions. This ensures that biofuel production aligns with local conditions, optimizing resource utilization and minimizing environmental impact. Furthermore, generative AI can aid in the development of sustainable agricultural practices for biomass cultivation [76–78]. It can analyze parameters such as soil quality, climate patterns, and crop rotation strategies to optimize the growth of biofuel feedstock crops, reducing reliance on synthetic fertilizers and Journal of Advances in Artificial Intelligence 20 Volume 2, Number 1, 2024
- 21. pesticides. By promoting sustainable agriculture, AI contributes to the overall sustainability of the biofuel supply chain. Beyond these practical applications, ChatGPT and similar AI models can foster collaboration and knowledge-sharing among researchers and experts in advanced biofuels. They can assist in data analysis, literature review, and the formulation of research hypotheses, thereby expediting innovation in biofuel development. AI-powered chatbots and virtual assistants can also provide answers and information regarding the latest advancements, facilitating the task of staying updated for scientists and engineers in the rapidly evolving field of biofuels. 14. Conclusions This research highlights the significant role that ChatGPT and generative artificial intelligence (AI) play in advancing a wide range of renewable and sustainable energy technologies. AI, including ChatGPT, makes valuable contributions and enhances performance in various areas such as solar energy, wind energy, biomass energy, hydropower, geothermal energy, energy storage, grid integration, energy efficiency, materials science, policy and economics, environmental impact and sustainability, energy access and equity, tidal and wave energy, and advanced biofuels. These technologies hold the potential to drive innovation, boost efficiency, and optimize sustainability in their respective domains. For example, AI can improve the efficiency of solar panels, enable predictive maintenance for wind turbines, and assist in selecting the right biomass feedstock. It can optimize hydropower operations, simulate the behavior of geothermal reservoirs, and ensure the reliability of energy storage systems. AI also plays a vital role in managing smart grids, providing recommendations for energy-efficient designs, and accelerating the discovery of materials for renewable technologies. Additionally, it can model various policy scenarios, address environmental concerns, and promote equitable access to energy resources. ChatGPT and generative AI have the potential to expedite the global transition toward renewable and sustainable energy sources, mitigating environmental impacts and enhancing energy accessibility while fostering economic growth. Nonetheless, it remains essential to carefully consider the ethical, environmental, and societal implications associated with deploying AI in the energy sector. Responsible integration of AI technologies will be critical to maximize benefits and minimize potential risks as we strive for a cleaner and more sustainable energy future. Conflict of Interest The authors declare no conflict of interest. References [1] Vaishya, R., Javaid, M., Khan, I. H., & Haleem, A. (2020). Artificial Intelligence (AI) applications for COVID-19 pandemic. Diabetes & Metabolic Syndrome: Clinical Research & Reviews, 14(4), 337–339. [2] Haleem, A., Javaid, M., & Khan, I. H. (2019). Current status and applications of Artificial Intelligence (AI) in medical field: An overview. Current Medicine Research and Practice, 9(6), 231–237. [3] Winston, P. H. (1984). Artificial Intelligence. Addison-Wesley Longman Publishing Co., Inc. [4] Haleem, A., Vaishya, R., Javaid, M., & Khan, I. H. (2020). Artificial Intelligence (AI) applications in orthopaedics: an innovative technology to embrace. Journal of Clinical Orthopaedics and Trauma, 11(Suppl 1), S80. [5] Owusu, P. A., & Asumadu-Sarkodie, S. (2016). A review of renewable energy sources, sustainability issues and climate change mitigation. Cogent Engineering, 3(1), 1167990. [6] Østergaard, P. A., Duic, N., Noorollahi, Y., Mikulcic, H., & Kalogirou, S. (2020). Sustainable development using renewable energy technology. Renewable Energy, 146, 2430–2437. [7] Fontes, C. H. D. O., & Freires, F. G. M. (2018). Sustainable and renewable energy supply chain: A system Journal of Advances in Artificial Intelligence 21 Volume 2, Number 1, 2024
- 22. dynamics overview. Renewable and Sustainable Energy Reviews, 82, 247–259. [8] Montzka, S. A., Dlugokencky, E. J., & Butler, J. H. (2011). Non-CO2 greenhouse gases and climate change. Nature, 476(7358), 43–50. [9] Hussain, A., Arif, S. M., & Aslam, M. (2017). Emerging renewable and sustainable energy technologies: State of the art. Renewable and Sustainable Energy Reviews, 71, 12–28. [10] Kannan, N., & Vakeesan, D. (2016). Solar energy for future world: A review. Renewable and Sustainable Energy Reviews, 62, 1092–1105. [11] Liu, C., Li, F., Ma, L. P., & Cheng, H. M. (2010). Advanced materials for energy storage. Advanced Materials, 22(8), E28–E62. [12] Ahmad, T., Zhang, D., Huang, C., Zhang, H., Dai, N., Song, Y., & Chen, H. (2021). Artificial intelligence in sustainable energy industry: Status Quo, challenges and opportunities. Journal of Cleaner Production, 289, 125834. [13] Patwa, N., Sivarajah, U., Seetharaman, A., Sarkar, S., Maiti, K., & Hingorani, K. (2021). Towards a circular economy: An emerging economies context. Journal of Business Research, 122, 725–735. [14] Milidonis, K., Blanco, M. J., Grigoriev, V., Panagiotou, C. F., Bonanos, A. M., Constantinou, M., ... & Asselineau, C. A. (2021). Review of application of AI techniques to solar tower systems. Solar Energy, 224, 500–515. [15] Meinel, A. B., & Meinel, M. P. (1977). Applied solar energy: An introduction. NASA STI/Recon Technical Report A, 77, 33445. [16] Gong, J., Li, C., & Wasielewski, M. R. (2019). Advances in solar energy conversion. Chemical Society Reviews, 48(7), 1862–1864. [17] Jiang, J. P., & Li, D. X. (2010). Optimal placement and decentralized robust vibration control for spacecraft smart solar panel structures. Smart Materials and Structures, 19(8), 085020. [18] Shin, W., Han, J., & Rhee, W. (2021). AI-assistance for predictive maintenance of renewable energy systems. Energy, 221, 119775. [19] Yang, D., Li, W., Yagli, G. M., & Srinivasan, D. (2021). Operational solar forecasting for grid integration: Standards, challenges, and outlook. Solar Energy, 224, 930–937. [20] Yang, D., Wang, W., Gueymard, C. A., Hong, T., Kleissl, J., Huang, J., ... & Peters, I. M. (2022). A review of solar forecasting, its dependence on atmospheric sciences and implications for grid integration: Towards carbon neutrality. Renewable and Sustainable Energy Reviews, 161, 112348. [21] Chui, K. T., Lytras, M. D., & Visvizi, A. (2018). Energy sustainability in smart cities: Artificial intelligence, smart monitoring, and optimization of energy consumption. Energies, 11(11), 2869. [22] Wei, N., Li, C., Peng, X., Zeng, F., & Lu, X. (2019). Conventional models and artificial intelligence-based models for energy consumption forecasting: A review. Journal of Petroleum Science and Engineering, 181, 106187. [23] Mohammad, A., & Mahjabeen, F. (2023). Revolutionizing solar energy with AI-driven enhancements in photovoltaic technology. BULLET: Jurnal Multidisiplin Ilmu, 2(4), 1174–1187. [24] Abdalla, A. N., Nazir, M. S., Tao, H., Cao, S., Ji, R., Jiang, M., & Yao, L. (2021). Integration of energy storage system and renewable energy sources based on artificial intelligence: An overview. Journal of Energy Storage, 40, 102811. [25] Haggi, H., Song, M., & Sun, W. (2019). A review of smart grid restoration to enhance cyber-physical system resilience. 2019 IEEE Innovative Smart Grid Technologies-Asia (ISGT Asia), 4008–4013. [26] Sulaiman, A., Nagu, B., Kaur, G., Karuppaiah, P., Alshahrani, H., Reshan, M. S. A., ... & Shaikh, A. (2023). Artificial Intelligence-Based Secured Power Grid Protocol for Smart City. Sensors, 23(19), 8016. [27] Zhao, E., Sun, S., & Wang, S. (2022). New developments in wind energy forecasting with artificial Journal of Advances in Artificial Intelligence 22 Volume 2, Number 1, 2024
- 23. intelligence and big data: A scientometric insight. Data Science and Management, 5(2), 84–95. [28] Garcia Marquez, F. P., & Peinado Gonzalo, A. (2021). A comprehensive review of artificial intelligence and wind energy. Archives of Computational Methods in Engineering, 1–24. [29] Higgins, S., & Stathopoulos, T. (2021). Application of artificial intelligence to urban wind energy. Building and Environment, 197, 107848. [30] Sholapurkar, R. B., & Mahajan, Y. S. (2015). Review of wind energy development and policy in India. Energy Technology & Policy, 2(1), 122–132. [31] Dragomir, G., Șerban, A., Năstase, G., & Brezeanu, A. I. (2016). Wind energy in Romania: A review from 2009 to 2016. Renewable and Sustainable Energy Reviews, 64, 129–143. [32] Abrahamson, W. G., & Caswell, H. (1982). On the comparative allocations of biomass, energy, and nutrients in plants. Ecology, 63(4), 982–991. [33] Meena, M., Shubham, S., Paritosh, K., Pareek, N., & Vivekanand, V. (2021). Production of biofuels from biomass: Predicting the energy employing artificial intelligence modelling. Bioresource Technology, 340, 125642. [34] Liao, M., & Yao, Y. (2021). Applications of artificial intelligence‐based modeling for bioenergy systems: A review. GCB Bioenergy, 13(5), 774–802. [35] Sakheta, A., Nayak, R., O'Hara, I., & Ramirez, J. (2023). A review on modelling of thermochemical processing of biomass for biofuels and prospects of artificial intelligence-enhanced approaches. Bioresource Technology, 129490. [36] Kumar, K., & Saini, R. P. (2021). Application of artificial intelligence for the optimization of hydropower energy generation. EAI Endorsed Transactions on Industrial Networks and Intelligent Systems, 8(28), e1-e1. [37] Rane, N. L., Achari, A., Choudhary, S. P., Mallick, S. K., Pande, C. B., Srivastava, A., & Moharir, K. (2023). A decision framework for potential dam site selection using GIS, MIF and TOPSIS in Ulhas river basin, India. Journal of Cleaner Production, 138890. [38] Feng, Z. K., Niu, W. J., Zhang, T. H., Wang, W. C., & Yang, T. (2023). Deriving hydropower reservoir operation policy using data-driven artificial intelligence model based on pattern recognition and metaheuristic optimizer. Journal of Hydrology, 624, 129916. [39] Quaranta, E., Bejarano, M. D., Comoglio, C., Fuentes-Pérez, J. F., Pérez-Díaz, J. I., Sanz-Ronda, F. J., ... & Tuhtan, J. A. (2023). Digitalization and real-time control to mitigate environmental impacts along rivers: Focus on artificial barriers, hydropower systems and European priorities. Science of The Total Environment, 875, 162489. [40] Moharir, K. N., Pande, C. B., Gautam, V. K., Singh, S. K., & Rane, N. L. (2023). Integration of hydrogeological data, GIS and AHP techniques applied to delineate groundwater potential zones in sandstone, limestone and shales rocks of the Damoh district,(MP) central India. Environmental Research, 228, 115832. [41] Jha, S. K., Bilalovic, J., Jha, A., Patel, N., & Zhang, H. (2017). Renewable energy: Present research and future scope of Artificial Intelligence. Renewable and Sustainable Energy Reviews, 77, 297–317. [42] Khosravi, A., Syri, S., Zhao, X., & Assad, M. E. H. (2019). An artificial intelligence approach for thermodynamic modeling of geothermal based-organic Rankine cycle equipped with solar system. Geothermics, 80, 138–154. [43] Hai, T., Ali, M. A., Chaturvedi, R., Almojil, S. F., Almohana, A. I., Alali, A. F., ... & Shamseldin, M. A. (2023). A low-temperature driven organic Rankine cycle for waste heat recovery from a geothermal driven Kalina cycle: 4E analysis and optimization based on artificial intelligence. Sustainable Energy Technologies and Assessments, 55, 102895. [44] Aminzadeh, F., Temizel, C., & Hajizadeh, Y. (2022). Artificial Intelligence and Data Analytics for Energy Journal of Advances in Artificial Intelligence 23 Volume 2, Number 1, 2024
- 24. Exploration and Production. John Wiley & Sons. [45] Bist, N., Tripathi, G., Sircar, A., & Yadav, K. (2021). Artificial intelligence based optimizing solutions for the geothermal power plants. Proceedings, 46th Workshop on Geothermal Reservoir Engineering, Stanford University, Stanford, California. [46] Khanmohammadi, S., Musharavati, F., & Tariq, R. (2022). A framework of data modeling and artificial intelligence for environmental-friendly energy system: Application of Kalina cycle improved with fuel cell and thermoelectric module. Process Safety and Environmental Protection, 164, 499–516. [47] Abdalla, A. N., Nazir, M. S., Tao, H., Cao, S., Ji, R., Jiang, M., & Yao, L. (2021). Integration of energy storage system and renewable energy sources based on artificial intelligence: An overview. Journal of Energy Storage, 40, 102811. [48] He, Z., Guo, W., & Zhang, P. (2022). Performance prediction, optimal design and operational control of thermal energy storage using artificial intelligence methods. Renewable and Sustainable Energy Reviews, 156, 111977. [49] He, Z., Guo, W., & Zhang, P. (2022). Performance prediction, optimal design and operational control of thermal energy storage using artificial intelligence methods. Renewable and Sustainable Energy Reviews, 156, 111977. [50] Olabi, A. G., Abdelghafar, A. A., Maghrabie, H. M., Sayed, E. T., Rezk, H., Al Radi, M., ... & Abdelkareem, M. A. (2023). Application of artificial intelligence for prediction, optimization, and control of thermal energy storage systems. Thermal Science and Engineering Progress, 101730. [51] Boretti, A. (2021). Integration of solar thermal and photovoltaic, wind, and battery energy storage through AI in NEOM city. Energy and AI, 3, 100038. [52] Krishna, G., Singh, R., Gehlot, A., Akram, S. V., Priyadarshi, N., & Twala, B. (2022). Digital technology implementation in battery-management systems for sustainable energy storage: Review, challenges, and recommendations. Electronics, 11(17), 2695. [53] Ali, S. S., & Choi, B. J. (2020). State-of-the-art artificial intelligence techniques for distributed smart grids: A review. Electronics, 9(6), 1030. [54] Khosrojerdi, F., Akhigbe, O., Gagnon, S., Ramirez, A., & Richards, G. (2021). Integrating artificial intelligence and analytics in smart grids: a systematic literature review. International Journal of Energy Sector Management, 16(2), 318–338. [55] Hua, W., Chen, Y., Qadrdan, M., Jiang, J., Sun, H., & Wu, J. (2022). Applications of blockchain and artificial intelligence technologies for enabling prosumers in smart grids: A review. Renewable and Sustainable Energy Reviews, 161, 112308. [56] Bayrak, G., & Yılmaz, A. (2021). Detection and classification of power quality disturbances in smart grids using artificial intelligence methods. In Artificial Intelligence (AI) (pp. 149-170). CRC Press. [57] Rane, N. L., Achari, A., Saha, A., Poddar, I., Rane, J., Pande, C. B., & Roy, R. (2023). An integrated GIS, MIF, and TOPSIS approach for appraising electric vehicle charging station suitability zones in Mumbai, India. Sustainable Cities and Society, 104717. [58] Zhu, S., Ota, K., & Dong, M. (2022). Energy-efficient artificial intelligence of things with intelligent edge. IEEE Internet of Things Journal, 9(10), 7525–7532. [59] Merabet, G. H., Essaaidi, M., Haddou, M. B., Qolomany, B., Qadir, J., Anan, M., ... & Benhaddou, D. (2021). Intelligent building control systems for thermal comfort and energy-efficiency: A systematic review of artificial intelligence-assisted techniques. Renewable and Sustainable Energy Reviews, 144, 110969. [60] Di Santo, K. G., Di Santo, S. G., Monaro, R. M., & Saidel, M. A. (2018). Active demand side management for households in smart grids using optimization and artificial intelligence. Measurement, 115, 152–161. [61] Khan, A. A., Laghari, A. A., Rashid, M., Li, H., Javed, A. R., & Gadekallu, T. R. (2023). Artificial intelligence Journal of Advances in Artificial Intelligence 24 Volume 2, Number 1, 2024
- 25. and blockchain technology for secure smart grid and power distribution Automation: A State-of-the-Art Review. Sustainable Energy Technologies and Assessments, 57, 103282. [62] Rocha, H. R., Honorato, I. H., Fiorotti, R., Celeste, W. C., Silvestre, L. J., & Silva, J. A. (2021). An Artificial Intelligence based scheduling algorithm for demand-side energy management in Smart Homes. Applied Energy, 282, 116145. [63] Sacha, G. M., & Varona, P. (2013). Artificial intelligence in nanotechnology. Nanotechnology, 24(45), 452002. [64] Rosa, V., Ho, D., Sabino-Silva, R., Siqueira, W. L., & Silikas, N. (2021). Fighting viruses with materials science: Prospects for antivirus surfaces, drug delivery systems and artificial intelligence. Dental Materials, 37(3), 496–507. [65] Ammar, R. B., Ammar, M. B., & Oualha, A. (2020). Photovoltaic power forecast using empirical models and artificial intelligence approaches for water pumping systems. Renewable Energy, 153, 1016–1028. [66] Zhang, S., Wang, J., Liu, H., Tong, J., & Sun, Z. (2021). Prediction of energy photovoltaic power generation based on artificial intelligence algorithm. Neural Computing and Applications, 33, 821–835. [67] Zahraee, S. M., Assadi, M. K., & Saidur, R. (2016). Application of artificial intelligence methods for hybrid energy system optimization. Renewable and Sustainable Energy Reviews, 66, 617–630. [68] Kanase-Patil, A. B., Kaldate, A. P., Lokhande, S. D., Panchal, H., Suresh, M., & Priya, V. (2020). A review of artificial intelligence-based optimization techniques for the sizing of integrated renewable energy systems in smart cities. Environmental Technology Reviews, 9(1), 111–136. [69] Rodriguez-Delgado, C., & Bergillos, R. J. (2021). Wave energy assessment under climate change through artificial intelligence. Science of the Total Environment, 760, 144039. [70] López, I., & Iglesias, G. (2014). Efficiency of OWC wave energy converters: A virtual laboratory. Applied Ocean Research, 44, 63–70. [71] Li, L., Gao, Y., Ning, D. Z., & Yuan, Z. M. (2021). Development of a constraint non-causal wave energy control algorithm based on artificial intelligence. Renewable and Sustainable Energy Reviews, 138, 110519. [72] Sakheta, A., Nayak, R., O'Hara, I., & Ramirez, J. (2023). A review on modelling of thermochemical processing of biomass for biofuels and prospects of artificial intelligence-enhanced approaches. Bioresource Technology, 129490. [73] Liu, J., Zhou, F., Abed, A. M., Le, B. N., Dai, L., Ali, H. E., ... & Zhang, G. (2022). Macroalgae as a potential source of biomass for generation of biofuel: Artificial intelligence, challenges, and future insights towards a sustainable environment. Fuel, 126826. [74] Ahmad, J., Awais, M., Rashid, U., Ngamcharussrivichai, C., Naqvi, S. R., & Ali, I. (2023). A systematic and critical review on effective utilization of artificial intelligence for bio-diesel production techniques. Fuel, 338, 127379. [75] Venkataramana, S. H., Shivalingaiah, K., Davanageri, M. B., Selvan, C. P., Lakshmikanthan, A., Chandrashekarappa, M. P. G., ... & Linul, E. (2022). Niger seed oil-based biodiesel production using transesterification process: experimental investigation and optimization for higher biodiesel yield using box–behnken design and artificial intelligence tools. Applied Sciences, 12(12), 5987. [76] Gautam, V. K., Pande, C. B., Moharir, K. N., Varade, A. M., Rane, N. L., Egbueri, J. C., & Alshehri, F. (2023). Prediction of sodium hazard of irrigation purpose using artificial neural network modelling. Sustainability, 15(9), 7593. [77] Aniza, R., Chen, W. H., Pétrissans, A., Hoang, A. T., Ashokkumar, V., & Petrissans, M. (2023). A review of biowaste remediation and valorization for environmental sustainability: Artificial intelligence approach. Environmental Pollution, 121363. Journal of Advances in Artificial Intelligence 25 Volume 2, Number 1, 2024
- 26. [78] Liao, M., & Yao, Y. (2021). Applications of artificial intelligence‐based modeling for bioenergy systems: A review. GCB Bioenergy, 13(5), 774–802. Copyright © 2024 by the authors. This is an open access article distributed under the Creative Commons Attribution License which permits unrestricted use, distribution, and reproduction in any medium, provided the original work is properly cited (CC BY 4.0). Journal of Advances in Artificial Intelligence 26 Volume 2, Number 1, 2024