Latest Jaunts @yeeguy
Latest Jaunts @yeeguy
Explore the significant themes related to World War II through an engaging quiz format. This interactive challenge invites participants to test their knowledge on historical facts, key figures, and impactful events of the era, providing both an educational experience and an enjoyable challenge for history enthusiasts.
Latest Jaunts @yeeguy
@maithri5 days ago
Latest Jaunts
@yeeguy
3 Followers
TerraFuture Seed Syndicate r8.pptx
TerraFuture
A Portfolio Approach to Invest in Nature-Based Solutions Opportunities , starting in Africa
Our Planet Our Future


1
0
ï½
0
îª
34
#carboncredits #sustainablefinance #naturebasedsolutions #clima
22 hours ago
@yeeguy
3 Followers
Terraformation Workshop at NOAH 2023.pptx
Terraformation
NOAH 2023
Carbon Forestry as a
Securitizable Asset Class
Yee Lee
ï½

1

0
yee@terraformation com
0
#forests #nbs #carbonremovals #terraformation
22 hours ago
@yeeguy
3 Followers
Terraformation at NOAH24.pptx
Terraformation
NOAH 2024
Yee Lee
yee@terraformation com
Terraformation


0
0
ï½
0
#forests #nbs #carbonremovals #terraformation
22 hours ago
@roshni
3 Followers
World War-2 Quiz
World War II Quiz
Roshni Ranjan
ï½
0
@yeeguy
+
FOLLOW
terrafuture org
verponts
Terraformation

2

@roshni
This is a quiz on World War-2 I have made.
Lets see how much you know about the World War-2! ... m
#history #historyquiz #trivia #adolfhitler #worldwar
1 day ago
@roshni
3 Followers
My Sketching and Drawing Album
MY SKETCHING & PAINTING ALBUM
BY ROSHNI RANJAN


2
@roshni
These are some of the sketches and paintings I
have created.
Take a stroll through my ... more
See more comments
#landscape #sketching #drawing #creativeexpression #painting
1 day ago
@roshni
3 Followers
Planets Quiz


2
@roshni
This is a quiz I created for the students in my
hobby class. Let's see how much you know!
#spacequiz #solarsystem #educationalchallenge #astronomyfun #
1 day ago
@roshni
3 Followers
Dandelions - A Poem
Dandelions
One Sunday morning,
After a light shower of rain
A small, beautiful dandelion rose in the air
It was different
the others, pure white
from
It glistened in the sunlight, sunny and bright!
The small dandelion was dancing around
All the other dandelions prancing around
Suddenly; a fierce wind picked up the air
The dandelion was angry at
separated from its friends.
being
It started to thunder and then came a rumbling sound
Thunder crashed, and it started to rain
The dandelion was swept away!
The wind whirled it around and rain poured water on it.
again!
The poor dandelion was sad
Then the dandelion saw a ray of light!


3
@roshni
Thank you!
See more comments
#childrensliterature #dandelions #adventure #joy #naturepoetry
1 day ago
@roshni
3 Followers
Conservation Of Forests
CONSERVATION OF
FORESTS
and
Devanshi Jain
+
FOLLOW
îª
27
3
ï½
0
+
FOLLOW
îª
37
1
ï½
+
FOLLOW
îª
27
2
ï½
0

2

@roshni
This is a project I made with my classmates on
Conservation of Forests.
The students who ... more
#a
"
orestation #enviroment #climatechange #wildlifeconservation
1 day ago
@roshni
3 Followers
Spreading Awareness on Mental Health
MENTAL
HEALTH
BY: ROSHNI
RANJAN
TRISHA BANERJEE
KRITI IYER


2
@roshni
This is a presentation that I have made with my
classmates on Mental Health. The students who have ... m
#mentalhealthawareness #health #depression #mentalhealth #me
1 day ago
@jonathan
21 Followers
Leveraged ETFs vs Margin Loans: why borrowing is
better
Leveraged ETFs vs. Margin Loans: Why Borrowing is Often Better
Leveraged ETFs, like TQQQ (3x leveraged Nasdaq-100) and UPRO (3x leveraged S&P
glance, they seem like a safer, simpler alternative to borrowing money to invest.
500), offer an easy way to amplify returns without taking on a margin Ioan. At first
However, when you compare the two approaches, margin loans often outperform
leveraged ETFs over time
The reason lies in how leveraged ETFs are structured, and
erode returns
how their mechanics_like beta slippage, roll yield, and tracking errors
To illustrate, lets compare the outcomes of investing $100 using a leveraged ETF
versus borrowing $100 to double your exposure to the underlying index.
The Scenario: A Volatile Market
Imagine an index starts at $100, rises 10% to $110, and then falls back 10% to $99.
We'll look at the outcomes for a 3x leveraged ETF and a margin loan over these two
Leveraged ETF (3x Daily Performance)
Day
goes from $100 to $130_
Day
1: The index rises from $100 to $110 (+10%). The ETF gains 30%, so it
2: The index falls from $110 to $99 (-10%). The ETF loses 30%, so it drops
from $130 to $91.
After two days: The index is down
% , but the leveraged ETF is down 9%.
Using a Margin Loan (2x Leverage)
With a margin loan, you invest $200 total-~your $100 plus $100 borrowed. If the index
your $220 becomes $198_
After two days: Your portfolio is down 1%, matching the index.
Why Borrowing Outperforms Leveraged ETFs
1. Beta Slippage: The Compounding Problem
Leveraged ETFs are designed to multiply the daily returns of the index, not its
long-term performance _
When the index rises and falls, the compounding effect works
9% while the index fell only 1%, a dramatic divergence caused by this daily
against the ETF over time, particularly in volatile markets. In our example, the ETF fell
compounding effect. With a margin loan, your returns directly track the index's
performance, so you avoid this slippage.


0
0
ï½
0
îª
43
#financeeducation #marginloans #investmentstrategy #financiala
1 day ago
@jonathan
21 Followers
Investing strategy for individual equities
Investing for the Long Haul: A Strategy to Minimize Decisions
and Maximize Returns
Investing is 90% a game of psychology: The biggest challenge isn't
One way to master this is by creating a strategy that minimizes the
number of decisions you need to make. Fewer decisions mean fewer
opportunities for mistakes, and in the long run, this can translate to
companies, building small initial positions, and protecting my
better outcomes. My approach focuses on selecting dominant
psychology while letting compounding do the heavy lifting
Ready to post
Complete file details
Title (Required)
Jaunt
ï
Category
Business
#Tags (Use # for tags, separate with space)
Summary (upto 500 characters)


0
0
ï½
0
îª
25
#emotionalintelligence #longterminvesting #investingstrategy #in
1 day ago
ï
Let AI suggest a summary
This presentation explores innovative
platforms that optimize dietary
requirement gathering for food services.
@jonathan
21 Followers
An American Life
It highlights how these platforms reduce
Add a comment
M
What's on your mind with this
doc/deck? (shows up as first
comment)
î©
PUBLISH YOUR POST
AN
A MERIGAN
FAMILY
ï½

0

0
0
îª
38
#culturalnarrative #americanheritage #personalstory #familyhistor
1 day ago
@jonathan
21 Followers
An American Life
AN
A MERIGAN
FAMILY
ï½

0

0
0
îª
26
#heritagestories #mythsandtruths #boutellelegacy #familyhistory
1 day ago
@Vikram
5 Followers
Digestive system
The Digestive
System!
By; Josh, Sameera, and Vikram
1


0
ï½
0
îª
81
#biology #humanbody #digestivesystem #nutrition #health
4 days ago
@jonathan
21 Followers
Material tranforms from disentangled NeRF
representations
Material transforms from disentangled NeRF representations
Ivan Lopes
Jean-François Lalonde?
'nria
Raoul de Charette
2Université Laval
novelviews
Transfer BRDF transform to new scenes
complex
o
scenes. From
observations of a scene in nwo conditions: original and transformed, we leverage a joint Neural Radiance Field (NeRF ) optimization to learn
Leamned
1
Learnlng BRDF transform from multivlews
Figure I: Proposed method We illustrate our approach for inferring unknown material transformations in
material mapping function F which models the observed changes at the material level accurately (e.g the topmost transform on the left is
Abstract
In this paper; we first propose
novel method for
disentangled Neural Radiance Field (NeRF) representations, our approach learns to map Bidirectional Reflectance Distribution
transferring material transformations across different scenes. Building on
Functions (BRDF) from pairs of scenes observed in varying conditions, such as dry and wet. The learned transformations can
then be applied to unseen scenes with similar materials,therefore effectively rendering the transformation learned with an
arbitrary level of intensity Extensive experiments on synthetic scenes and real-world objects validate the effectiveness of our
only the versatility of our method but also its potential for practical applications in computer graphics. We publish our method
implementation, along with our synthetichreal datasets on https:/ /github.com/astra
Introduction
key
In computer graphics and vision, inverse rendering is
ing
toextract-
material information and allowing re-rendering under novel
While neural repre-
sentations have largely taken over the traditional Physically-Based
conditions (viewpoint, lighting, materials, etc.)
Rendering (PBR) techniques, recent works have demonstrated that
presery-
the two representations can be combined [JLX* 23]. thus
ing
the editability and expressivity of PBR representations along
with the flexibility of neural representations.

1

0
ï½
1 week ago
@jonathan
21 Followers
Incentive Design With Spillovers
INCENTIVE DESIGN
KRISHNA DASARATHA
ABSTRACT.
principal
uses
WITH SPILLOVERS
BENJAMIN GOLUB. AND ANANT SHAH
payments conditioned
stochastic outcomes of a team
project to elicit costly effort from the team members. We
ization of a classic first-order
from network games.
tive pay
across
agents; a
'develop
multi-agent general-
approach to contract optimization by leveraging methods
The main results characterize the optimal allocation of incen-
agents and outcomes.
Incentive optimality requires equalizing; across
product of (i) individual productivity (ii) organizational centrality and (iii)
responsiveness to monetary incentives.
Dasaratha:
western
krishnadasaratha@
University:
to Drew
Shah:
Fudenberg and his reading group for a very valuable discussion_
ben golub @gmail com,
North-
We thank
We are
and also for helpful comments
Omer Tamuz, Marina Halac, Thomas Steinke, Marzena Rostek, Daron Ravid, Jose Betancourt
to Ilya Segal,
Mailath;
George
lencia, Roberto Corrao, Jacopo Perego; Juan Ortner,
Gottardi, and Michael Powell,

E
1

0
0
ï½
grateful
Va-
Adam Szeidl, Sam Wycherley; Michael Ostrovsky; Elliot Lipnowski, Yifan Dai, Melika Liporace; Piero
as well
0
îª
84
1 week ago
@kapilmohan
9 Followers
Can AI Sniff Like Humans?
SNIFF AI: Is MY
YOUR
SPICY
PERCEPTUAL ALIGNMENT WITH HUMAN SMELL ExPERIENCES
Shu
Zetao Zhou? , Christopher Dawes"
Giada Brianz
and Marianna Obrist
'Zhong
Department of Computer Science, University College London; United Kingdom
2Division of Psychology and Language Sciences, University College London; United Kingdom
Further erploration analyses on how
Al claude
lavender: (The scent is floral
and fresh with a subtle sweetness and
herbal undertones.Ithas
calming and
soothing quality that can evoke feelings
of relaxation and tranquility.The aroma is
clean and
light, reminiscent of
breeze passing through
on
garden ,
The relerence kent
gentle
Alex Wolitzky;
Jason Hartline,
as many seminar and conference participants.
'gmail
0
îª
143
When considering the appearance of
scene
coat of varnish)
certain transfor-
canalter the ma-
scene
change drastically . Currently, estimating the PBR characteristics of
known material after such
scene again in the desired target condition. This process is both
and
complex
transformation requires capturing the
laborious due t0 the variety of possible transforma -
tions; such as wetness, dust, varnish, painting. etc. In this work
aim to lcarn
BRDF transformation from
it to different scenes.
source scene and
WC
apply
MYTHS
HALF
Will Boutelle
LIFE
TRUTHS
MYTHS
TRUTHS
LIFE
& HALF TRUTHS
Will Boutelle
1
ï½
0
3
g
1
5
g
1

0
quite
this Smell. The Sent
more
fowery and Jweet compared
Smells
does
which
Smells _
I: SniffAl: an overview of user study and examples from the user study.
Figure
ABSTRACT
Aligning Al with human intent is important, yet perceptual alignment
how Al interprets what
on
This work focuses
user study with 40 participants to investigate how well AI can interpret
olfaction; human smell
interactive tasks. with our
hear, or smell-
experiences.
human
designed Al system attempting to guess what scent the participants were experiencing based on their
remains underexplored.
We conducted
descriptions of scents. Participants performed "sniff and describe'
descriptions. These tasks evaluated the Large Language Model's (LLMs) contextual understanding
and representation of scent relationships within its internal states
space:
Results indicated limited perceptual alignment, with biases towards certain scents, like lemon and
Both quantitative and qualitative methods were used to evaluate the Al system
failing
peppermint, and continued
high-dimensional embedding
identify others, like rosemary. We discuss these
HCI systems with multisensory experience integration.
performance.
'findings in light
alignment advancements; highlighting the limitations and opportunities for enhancing
Introduction
Aligning Artificial intelligence (Al) behaviour with human preference is critical for the future of AL An important yet
between Alassessments and human
often overlooked aspect of this alignment is the perceptual alignment . Perceptual alignment refers to the agreement

0
ï½
2 weeks ago
@jonathan
21 Followers
SMoA: Improving Multi-agent Large Language
Models with Sparse Mixture-of-Agents
SMoA: Improving Multi-agent Large Language Models with
Sparse Mixture-of-Agents
Dawei Li'
Kumar Satvik Chaudhary
Zhen Tan' , Peijia Qian? , Yifan Li' ,
'School of Computing, and Augmented Intelligence, Arizona State University
Lijie Hu' , Jiayi Shens
2Independent Researcher
Abdullah University of Science and Technology
'Computer Science and Engineering, Michigan State University
SUniversity of Texas at Austin
'King
Abstract
multi
While
significantly enhance the performance of
agent systems have been shown
Large Language Models (LLMs)
across
var-
ious tasks and applications, the dense interac -
tion between
pers their efficiency and diversity. To address
scaling
these challenges,
agents potentially ham-
inspiration from
pose a sparse mixture-of-agents (SMoA) frame -
the sparse mixture-of-agents (SMoE) and pro -
work to improve the efficiency and diversity
of multi-agent LLMs. Unlike completely con-
nected structures, SMoA introduces novel Re-
sponse Selection and Early Stopping mecha-
nisms to sparsify information flows among in-
dividual LLM agents; striking a balance be-
tween
ally; inspired by the expert diversity principle
in SMoE frameworks for workload balance
between experts.
we
scriptions to each LLM agent, fostering diverse
and
On
reasoning, alignment, and fairness bench-
marks demonstrate that SMoA
formance
agents approaches but with significantly lower
comparable to traditional mixture-of-
computational costs. Further analysis reveals
that SMoA
pacity
is more stable, has a greater
to scale,
ca-
and offers considerable po-
tential through hyper-parameter optimization.
Code and data will be available
at:
Ilgithub. com/David-Li0406/SMoA.
Introduction
The rapid development of Large Language Models
(LLMs) (Brown et al., 2020; Anil et al., 2023; Gan
et al., 2023; Dubey et al., 2024) in recent years
has significantly advanced in a series of NLP tasks;
such
Tong
as
et al., 2023; Jin et al., 2024a), knowledge dis-
covery (Li et al , 2024a,b; Tan et al , 2024a) and
While these powerful foundation models have been
question answering (Wang et al., 2022;
systems (Li et al, 2022; Sun et al., 2024).
dialogue
proven to benefit from extensive training data and

1
1

0
0
ï½
2 weeks ago
@jonathan
21 Followers
Causal Responsibility Attribution for Human-AI
Collaboration
Causal Responsibility Attribution for Human-AI Collaboration
Yahang Qi
ETH Zurich
Bernhard Schölkopf
MPI
Tübingen
Zhijing Jin
University of Toronto
YAHAQI@ETHZ.CH
BS@TUEBINGEN .MPG.DE
ZJIN@CS.TORONTO.EDU
0
îª
92
https:
assign distinct role de-
divergent thinking. Extensive experiments
achieves per-
Prompt
Output
Multi-agent debate
Output
MoA
Output
SMoA (ours)
Figure I: Comparison among the pipeline structures of
MAD, MoA and SMoA
larger model sizes, further scaling up these mod-
retraining
els is exceptionally costly, often requiring necessi -
several trillion tokens (Zhang
tating
et al., 2024). To overcome this limitation, multi -
agent LLMs (Liang et al , 2023;
et al , 2024b)
have been explored, enabling LLM systems to in-
Wang
corporate multiple agents; each focused on distinct
objectives and tasks.
The
Wang
based structure
(Liang et al., 2023;
et al., 2024b) is one of the
layer-|
multi-agent systems.
various
LLMs
to
most funda-
involves
perform
(MAD) (Liang et al., 2023; Du et al., 2023) or dis-
multi-agent debate
cussion over multiple rounds to mimic human be-
havior in problem-solving.
While effective in vari -
ous applications, early layer-based methods (Li
agent each time, hindering their utilization in
et al., 2023) process user queries with only one
real-
(MoA)
-world scenarios. Recently, mixture-of-agents
et al., 2023;
et al., 2024b) has
been devised, which uses multiple processors per
(Zhang
Wang
layer to handle queries simultaneously for time ef-
these references to produce a final answer:
ficiency purposes. An aggregator then synthesizes
Although MoA improves the time efficiency of
multi-agent LLMs, it still faces
significant chal -
lenges. The first issue is the high token computa-
tional cost. While MoA reduces processing time
instructing
subjective judgments across different sensory modalities, such as vision, hearing,
0
îª
65
ž
1
1
1

0
in critical sectors
such as healthcare (Budd et al , 2021), finance (Cohen et al, 2023), and autonomous driving (Badue
Abstract
As Artificial Intelligence (Al) systems increasingly influence decision-making across various fields,
the need to attribute responsibility for undesirable outcomes has become essential, though compli -
cated by the complex interplay between humans and AL
actual
to an outcome and rely on real-world measures of blameworthiness that may misalign with respon -
Existing attribution methods based
on
causality and Shapley values tend to disproportionately blame agents who contribute more
sible Alstandards. This paper presents a causal framework
Structural Causal Models (SCMs)
to systematically attribute responsibility in human-AI systems; measuring overall blameworthiness
using
while employing counterfactual reasoning to account for agents' expected epistemic levels. Two
Keywords: Responsibility Attribution, Causal Inference, Human-AI Collaboration; Decision-Making
case studies illustrate the framework 's adaptability in diverse human-AI collaboration scenarios.
1. Introduction
As Artificial Intelligence (Al) systems increasingly influence decision
-making
et al., 2021), the need to clearly define and attribute responsibility when outcomes are undesirable
Or
failures occur becomes crucial
complicates traditional accountability mechanisms due to the shared decision-making responsibili -
Santoni de Sio and Van den Hoven, 2018). The integration of AI
ties between humans and algorithms.
attribution; given their interactive, complex, and high-stakes nature
Human-AI systems pose unique challenges for responsibility
Amershi et al., 2019). On one
hand, the ability of humans to override or modify Al-driven decisions introduces an element of un-
predictability in outcomes. On the other hand, Al decisions often rely on huge datasets or complex
anticipate Al behaviour:
models that lack full transparency;
To address these
in multi-agent settings.
making it difficult for human counterparts to fully understand or
challenges; various methods have been proposed for responsibility attribution
Chockler and Halpern (2004)
and Halpern and Kleiman-Weiner (2018)
introduced frameworks to quantify blameworthiness based
was
On
causal relationships.
This definition
further extended to multi-agent settings by attributing blameworthiness through the Shapley
value, as demonstrated by
has been studied in decentralized , partially observable Markov decision processes
Friedenberg and Halpern (2019). Additionally; responsibility attribution
of actual causality (Triantafyllou et al., 2022) _
2016) to measure the degree of blameworthiness among agents.
Our code and data arc at
https:/ /github
Preprint. Under review.

0
ï½
0
îª
63
2 weeks ago
@BPGPeixoto
4 Followers
Who Defines Me? A book about SJIA.
WHO
DEFINES
A
non-fictional book about
juvenile arthritis
These approaches rely on actual causality (Halpern,
com / yahang-qi/Causal-Attr-Human-AI.git.
the concept
using

BY
Beatriz Peixoto
1

1
@BPGPeixoto
A 10th grade project where I wrote a
book about SJIA
#personaljourney #sjiafoundation #chronicillness #personalproject
3 weeks ago
î«
Home
Upload
ï½
0
îª
67
1
1
ï½
Science Quiz
By Roshni Ranjan
0
0
î«
mations (such
@yeeguy
applying
Golub:
Pu
ﶪ
Pus
+
+
+
+
+
blooming
Pu
2
M
FOLLOW
îª
19
FOLLOW
îª
13
FOLLOW
îª
28
FOLLOW
îª
FOLLOW
îª
26
x
Use
28

Related Jaunts
More from author
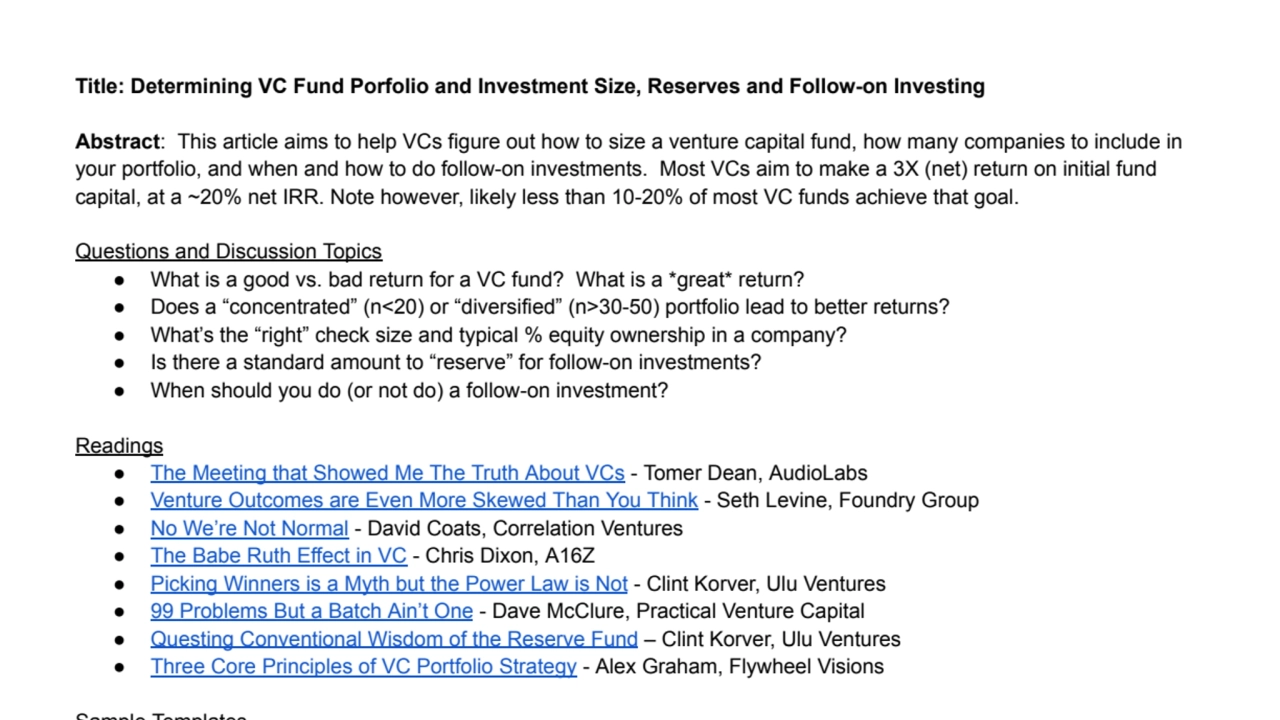
Determining VC Fund Portfolio and Investment Size, Reserves and Follow-on Investing
@maithri
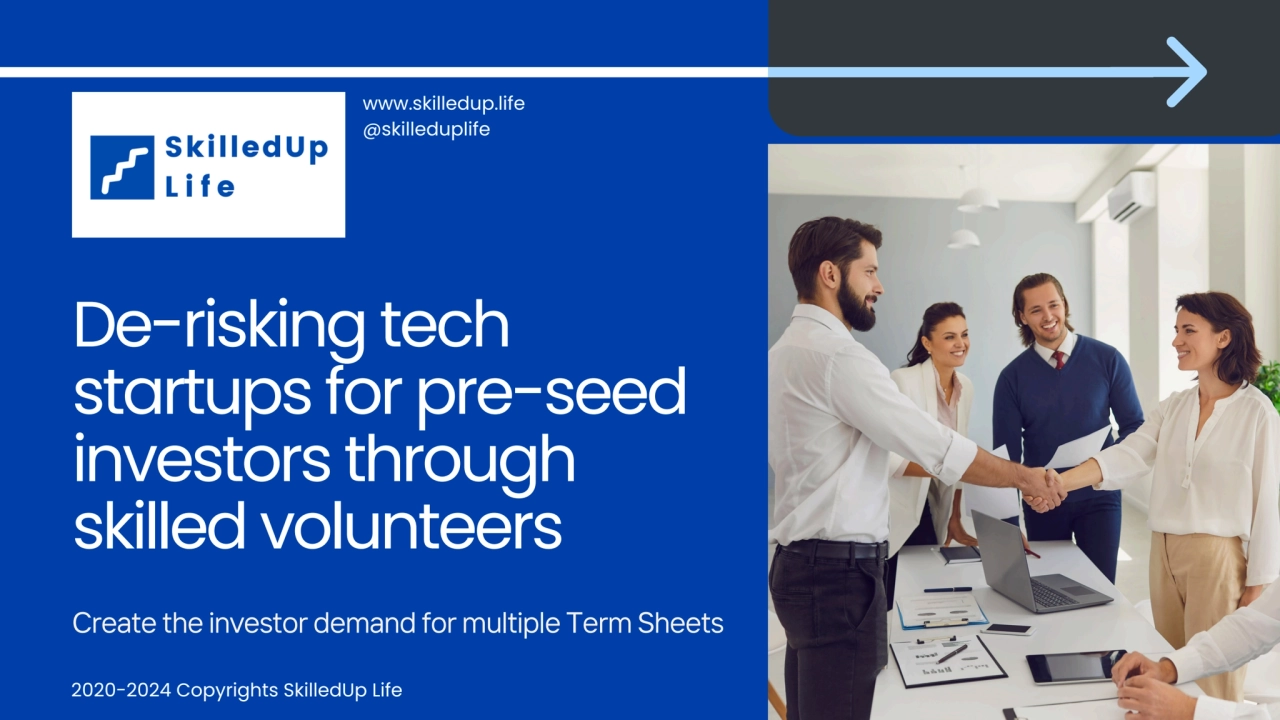
De-risking tech startups for pre-seed investors through skilled volunteers
@maithri
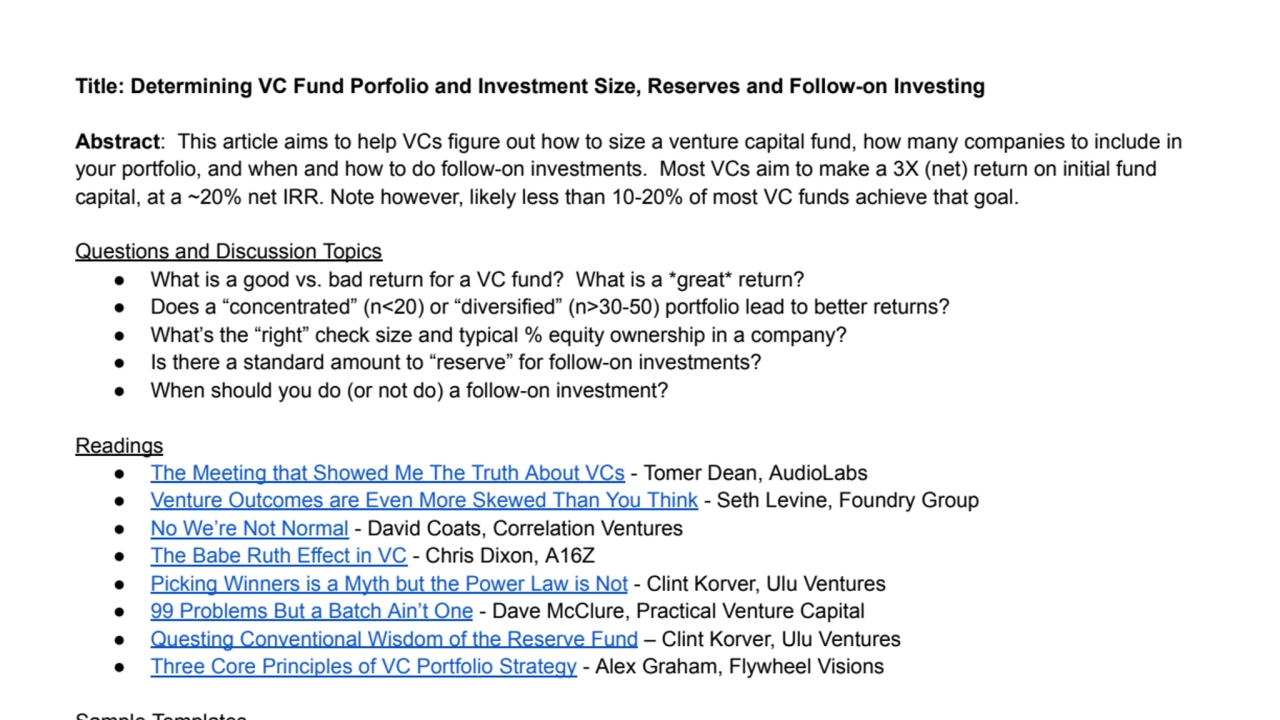